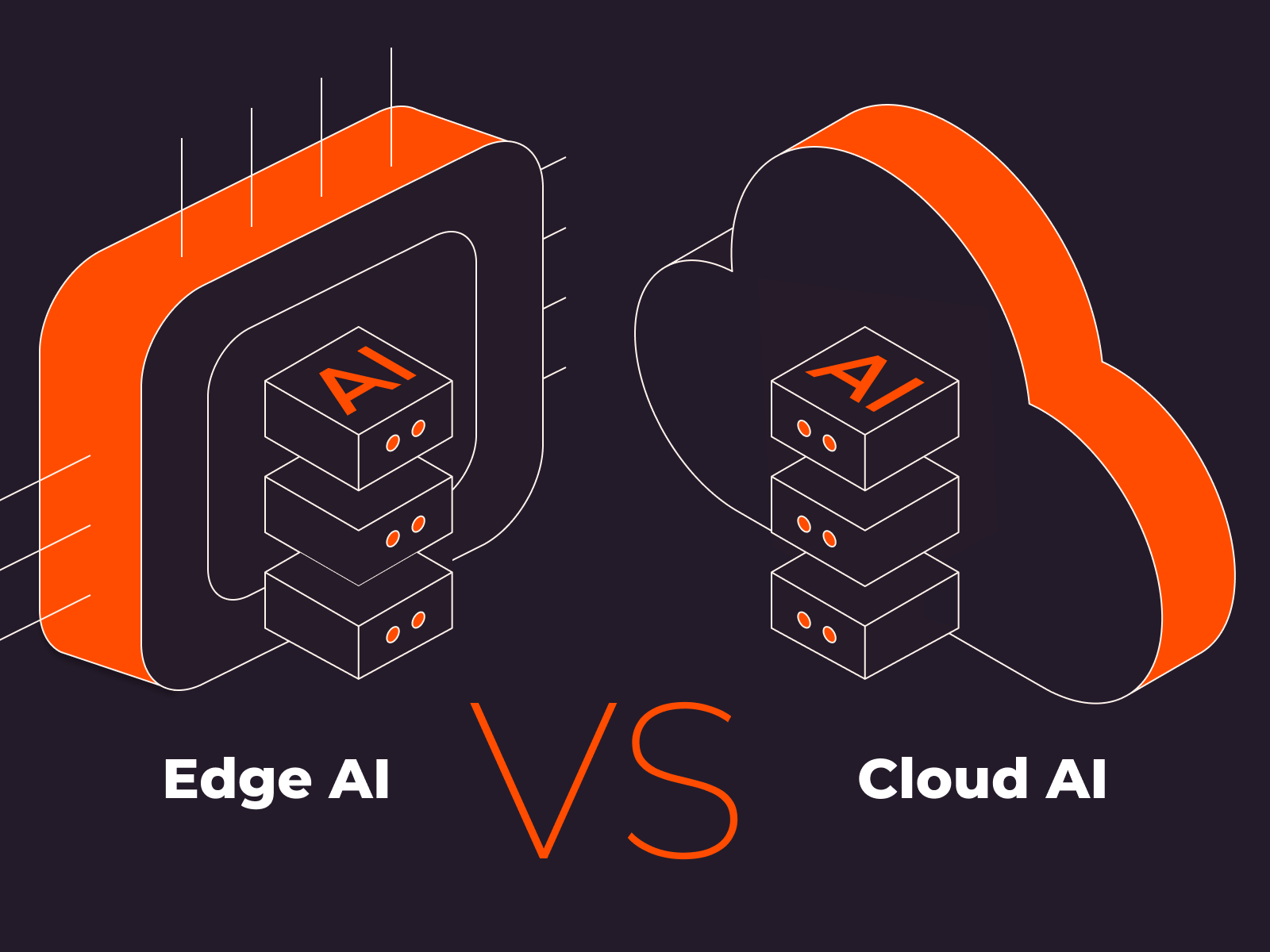
In todayâs fast-paced technological environment, grasping the distinct functions and capabilities of Edge AI and Cloud AI is essential for businesses aiming to utilize artificial intelligence effectively. Organizations must consider the advantages, disadvantages, and associated costs of these technologies. This article will offer a detailed overview of both Edge and Cloud AI, helping you determine the most suitable option for your project.
What is Edge AI
Edge AI is the deployment of artificial intelligence algorithms directly on local devices at the âedgeâ of the network, rather than depending on centralized cloud servers. This approach allows data processing to take place at or near the point of data production.
Processing Data Locally
Edge AI processes data instantly on the devices from which it is collected. This on-device processing eliminates the need for data to be transmitted over the network, resulting in faster response times and improved data security.
Examples of Edge AI Devices
Here are a few examples of Edge AI devices:
- IoT Devices. Smart thermostats, security cameras, and other connected devices that process data locally.
- Wearables. Devices like smartwatches and fitness trackers that monitor and analyze data right on the userâs body.
- Edge Servers. Localized servers that provide on-site processing capabilities for larger systems like manufacturing plants or retail chains.
Advantages of Edge AI
Here are a few advantages of Edge AI:
- Reduced Latency and Improved Real-Time Responses. Immediate processing of data without the lag associated with sending information to the cloud.
- Enhanced Privacy and Security. Keeps sensitive data localized, reducing the risk of data breaches during transmission.
- Operational Cost Savings. Decreases the need for continuous cloud computing resources and data transmission costs.
Disadvantages of Edge AI
Here are a few disadvantages of Edge AI:
- Limited Computing Power and Storage. Edge devices generally have less processing power and storage capacity compared to cloud servers.
- Higher Hardware Costs and Maintenance Needs. Initial setup and ongoing maintenance of edge devices can be more costly and complex.
- Potential Security Vulnerabilities. While data transmission risks are lower, edge devices themselves can be vulnerable to physical or cyber attacks.
Understanding the functionalities and limitations of Edge AI is crucial for businesses aiming to effectively implement AI solutions tailored to their specific operational environments and needs. In the next section, we will explore Cloud AI, discussing its advantages and addressing some concerns that need consideration.
What is Cloud AI
Cloud AI involves using centralized cloud servers for data processing and storage, allowing complex AI models and algorithms to be executed on a large scale. This technology takes advantage of the huge resources of cloud computing platforms, effortlessly integrating with services from Cloud providers. Here are two key aspects of how cloud AI works:
- Processing and Storage of Data on Remote Servers. Cloud AI systems handle vast amounts of data remotely, providing the computational power necessary to perform complex data processing tasks far beyond the capability of local devices.
- Integration with Cloud Computing Platforms. Cloud AI is deeply integrated with cloud computing platforms that provide robust, scalable environments supporting a wide range of AI functionalities.
Advantages of Cloud AI
Here are a few advantages of Cloud AI:
- Scalability and Flexibility. Easily scales to meet the demands of growing data and increasingly complex applications, accommodating fluctuations without the need for physical infrastructure changes.
- High Processing Power. Suitable for demanding AI tasks that require significant computational resources to perform activities such as deep learning and large-scale data analytics.
- Access to Large Datasets. Facilitates improved model training by providing access to extensive datasets that are often impractical to store and process on local devices.
Disadvantages of Cloud AI
Here are a few disadvantages of Cloud AI:
- Latency Issues in Real-Time Applications. The time taken for data to travel to and from the cloud can result in delays, which is critical in applications requiring instant decision-making.
- Security and Privacy Concerns. While cloud platforms generally offer robust security measures, transmitting sensitive data to and from the cloud can increase the risk of breaches.
- Dependence on Stable Internet Connectivity. The effectiveness of Cloud AI is heavily reliant on consistent and reliable internet access, which can be a significant limitation in remote or unstable network areas.
The fantastic features of Cloud AI are significant for managing large-scale AI tasks. Still, they must be carefully analyzed to ensure compliance with the deployment environmentâs requirements and limits. In the following section, we will compare Edge AI with Cloud AI, looking at real-world applications to help you decide which is best for your needs.
How to Choose Between Edge AI and Cloud AI: Situational Suitability and Real-World Applications
Understanding when and when to use Edge AI versus Cloud AI is crucial for improving the performance and usability of artificial intelligence technologies in a variety of settings. This comparative analysis offers advice on situational suitability based on unique operational requirements and demonstrates practical applications using real-world case studies.
Situational Suitability
- When to Use Edge AI:
- Real–timeProcessing. Edge AI is ideal for scenarios requiring immediate data processing without latency, such as autonomous driving and manufacturing automation.
- Limited Connectivity. In environments where internet access is unreliable or unavailable, Edge AI operates effectively as it processes data locally.
- When to Use Cloud AI:
- Large–scaleData Processing. Cloud AI is better suited for applications that need to handle large volumes of data that require significant computational power, such as training complex machine learning models.
- Complex Model Requirements. When the AI application demands extensive computational resources and advanced model capabilities, Cloud AI provides the necessary infrastructure to support these needs.
Real-World Applications
- Industrial IoT and Healthcare (Edge AI):
- Industrial IoT. Edge AI enables real-time monitoring and response systems within industrial settings, improving efficiency and safety by processing data directly from IoT devices without the need for cloud transmission.
- Healthcare. In medical devices and monitoring systems, Edge AI provides immediate processing capabilities essential for patient care, where quick decision-making is crucial.
- Big Data Analytics and Machine Learning (Cloud AI):
- Big Data Analytics. Cloud AI leverages the power of cloud computing to analyze vast datasets quickly and efficiently, enabling businesses to gain insights from big data which can inform strategic decisions.
- Machine Learning. With access to expansive computational resources, Cloud AI supports the development and deployment of complex machine learning models that learn from large and diverse datasets to improve over time.
Use Case | Technology | Advantages | Key Applications |
Real-time Processing | Edge AI | Immediate data processing, operates offline | Autonomous vehicles, manufacturing sensors |
Limited Connectivity | Edge AI | Functional without internet connectivity | Remote monitoring, field operations |
Large-scale Data Processing | Cloud AI | Handles vast amounts of information | Data analytics, large-scale simulations |
Complex Model Requirements | Cloud AI | Supports advanced computational capabilities | AI model training, deep learning |
This analysis underscores the complementary nature of Edge and Cloud AI technologies, highlighting how each can be utilized to fulfill specific technological and operational needs within diverse sectors such as industrial automation, healthcare, and data-driven decision-making processes.
Integrating Edge and Cloud AI
Integrating Edge and Cloud AI can create a more robust AI infrastructure that leverages the strengths of both approaches. This section explores the benefits of a hybrid model, as well as the challenges and considerations involved in implementing such a system.
Benefits of a Hybrid Approach
Balancing Real-Time Processing with Deep Analytics
- Real-Time Processing. Edge AI can process data locally, reducing latency and allowing for immediate responses necessary in scenarios like autonomous driving or emergency medical services.
- Deep Analytics. Cloud AI can handle complex analyses and manage large datasets that provide deeper insights, which can be used to enhance operational strategies and long-term planning.
Aspect | Edge AI Capabilities | Cloud AI Capabilities |
Data Processing | Immediate, at the source | Complex, involving large datasets |
Primary Benefit | Reduces latency | Enhances depth of analysis |
Enhancing Model Performance Through Continuous Learning
- Edge AI can make immediate adjustments based on real-time data, while Cloud AI can update and refine models based on accumulated data over time, leading to progressively better decision-making and predictive capabilities.
Model Learning | Edge AI Approach | Cloud AI Approach |
Immediate Adjustments | Adjusts operations in real-time | Uses historical data for model refinement |
Continuous Improvement | Limited by local data | Benefits from vast, diverse data sources |
Challenges and Considerations
- Architectural Complexities
- Integrating Edge and Cloud AI requires careful design to ensure compatibility and effective communication between disparate systems. This includes choosing the right hardware and software that can seamlessly interact within a hybrid framework.
Challenge | Description |
System Compatibility | Ensuring edge devices and cloud platforms can communicate effectively |
Design and Implementation | Complex setup involving multiple components and stakeholders |
- Coordinating Data Flow and Processing Between Edge and Cloud
- Effective data management strategies must be developed to handle the flow of data from edge devices to the cloud and vice versa. This includes managing bandwidth, storage, and ensuring data integrity and security throughout the process.
Data Management Aspect | Edge AI Considerations | Cloud AI Considerations |
Bandwidth and Storage | Efficient data transmission | Adequate storage for incoming data |
Security and Integrity | Secure data at source | Protect data in transit and at rest |
Implementing a hybrid Edge and Cloud AI system can significantly enhance an organizationâs AI capabilities by leveraging the immediate responsiveness of Edge AI with the powerful processing and analytical capabilities of Cloud AI. However, the integration requires addressing significant architectural and data management challenges to ensure a smooth and efficient operation.
Conclusion
As AI continues to evolve, selecting the right infrastructureâbe it Edge AI, Cloud AI, or a hybrid approachâis crucial for optimizing scalability, efficiency, and flexibility in AI applications. Carefully assessing your specific business needs and future technological trends will enable informed decisions that enhance your AI capabilities and align with your strategic objectives.
If youâre looking to take your AI projects to the next level, Gcoreâs AI Infrastructure could be just what you need. Our approach, designed exclusively for AI and compute-intensive workloads, takes advantage of GPUsâ hundreds of cores to accelerate AI training and manage the high demands of deep learning models.