High Availability Kubernetes Monitoring using Prometheus and Thanos
- By Gcore
- March 31, 2023
- 13 min read
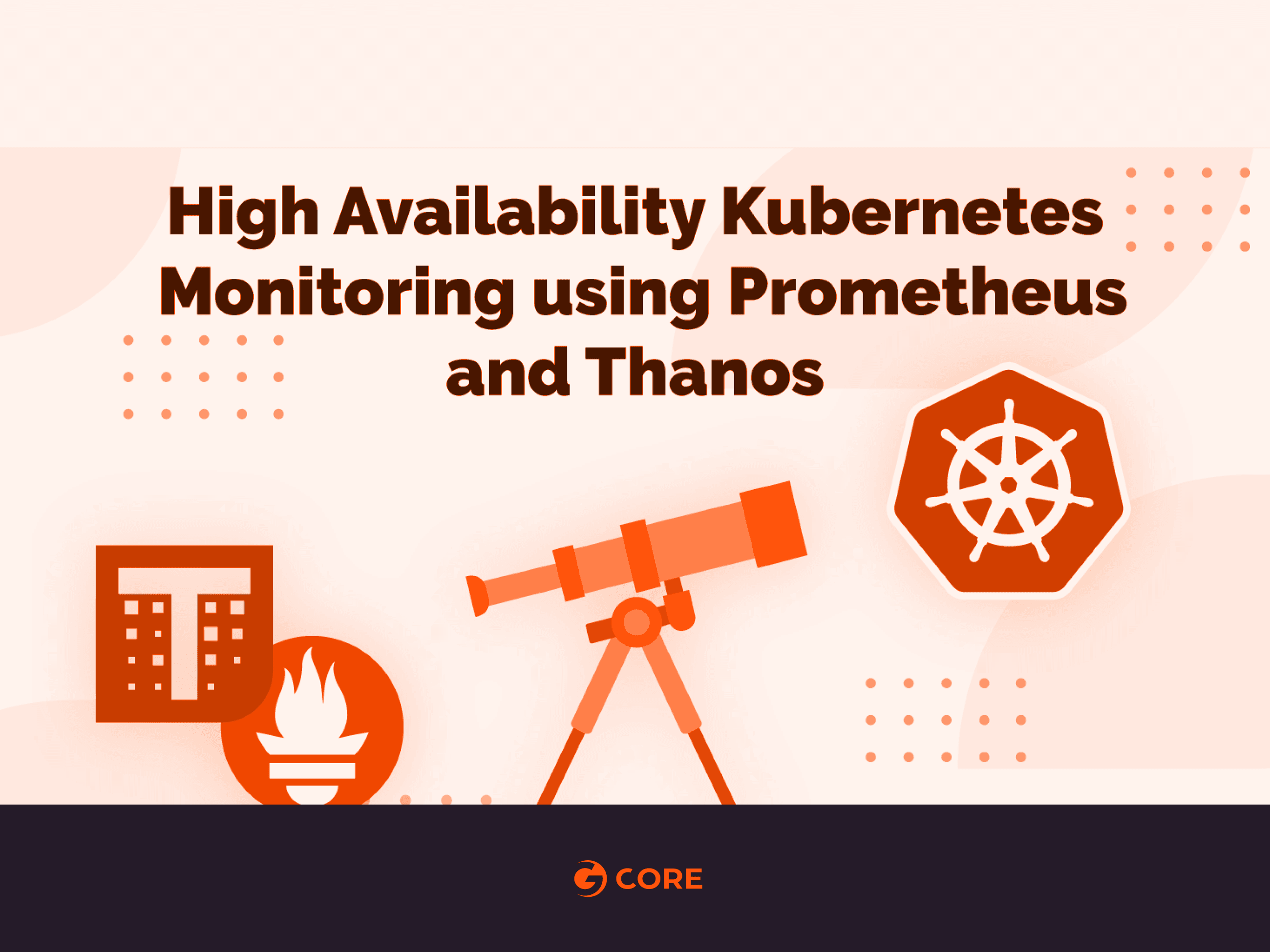
Introduction
The need for Prometheus High Availability
Kubernetes adoption has grown multifold in the past few months and it is now clear that Kubernetes is the defacto for container orchestration. That being said, Prometheus is also considered an excellent choice for monitoring both containerized and non-containerized workloads. Monitoring is an essential aspect of any infrastructure, and we should make sure that our monitoring set-up is highly-available and highly-scalable in order to match the needs of an ever growing infrastructure, especially in the case of Kubernetes.
Therefore, today we will deploy a clustered Prometheus set-up which is not only resilient to node failures, but also ensures appropriate data archiving for future references. Our set-up is also very scalable, to the extent that we can span multiple Kubernetes clusters under the same monitoring umbrella.
Present scenario
Majority of Prometheus deployments use persistent volume for pods, while Prometheus is scaled using a federated set-up. However, not all data can be aggregated using a federated mechanism, where you often need a mechanism to manage Prometheus configuration when you add additional servers.
The Solution
Thanos aims at solving the above problems. With the help of Thanos, we can not only multiply instances of Prometheus and de-duplicate data across them, but also archive data in a long term storage such as GCS or S3.
Implementation
Thanos Architecture
Image Source: https://thanos.io/quick-tutorial.md/
Thanos consists of the following components:
- Thanos Sidecar: This is the main component that runs along Prometheus. It reads and archives data on the object store. Moreover, it manages Prometheus’ configuration and lifecycle. To distinguish each Prometheus instance, the sidecar component injects external labels into the Prometheus configuration. This component is capable of running queries on Prometheus servers’ PromQL interface. Sidecar components also listen on Thanos gRPC protocol and translate queries between gRPC and REST.
- Thanos Store: This component implements the Store API on top of historical data in an object storage bucket. It acts primarily as an API gateway and therefore does not need significant amounts of local disk space. It joins a Thanos cluster on startup and advertises the data it can access. It keeps a small amount of information about all remote blocks on local disk and keeps it in-sync with the bucket. This data is generally safe to delete across restarts at the cost of increased startup times.
- Thanos Query: The Query component listens on HTTP and translates queries to Thanos gRPC format. It aggregates the query result from different sources, and can read data from Sidecar and Store. In a HA setup, it even deduplicates the result.
Run-time deduplication of HA groups
Prometheus is stateful and does not allow replicating its database. This means that increasing high-availability by running multiple Prometheus replicas are not very easy to use. Simple load balancing will not work, as for example after some crash, a replica might be up but querying such replica will result in a small gap during the period it was down. You have a second replica that maybe was up, but it could be down in another moment (e.g rolling restart), so load balancing on top of those will not work well.
- Thanos Querier instead pulls data from both replicas, and deduplicate those signals, filling the gaps if any, transparently to the Querier consumer.
- Thanos Compact: The compactor component of Thanos applies the compaction procedure of the Prometheus 2.0 storage engine to block data stored in object storage. It is generally not semantically concurrency safe and must be deployed as a singleton against a bucket.
It is also responsible for downsampling of data – performing 5m downsampling after 40 hours and 1h downsampling after 10 days. - Thanos Ruler: It basically does the same thing as Prometheus’ rules. The only difference is that it can communicate with Thanos components.
Configuration
Prerequisite
In order to completely understand this tutorial, the following are needed:
- Working knowledge of Kubernetes and using kubectl
- A running Kubernetes cluster with at least 3 nodes
- Implementing Ingress Controller and ingress objects (for the purpose of this demo Nginx Ingress Controller is being used). Although this is not mandatory but it is highly recommended inorder to decrease the number of external endpoints created.
- Creating credentials to be used by Thanos components to access object store (in this case GCS bucket)
- Create 2 GCS buckets and name them as prometheus-long-term and thanos-ruler
- Create a service account with the role as Storage Object Admin
- Download the key file as json credentials and name it as thanos-gcs-credentials.json
- Create kubernetes secret using the credentials
kubectl create secret generic thanos-gcs-credentials --from-file=thanos-gcs-credentials.json -n monitoring
Deploying various components
Deploying Prometheus Services Accounts, Clusterrole and Clusterrolebinding
apiVersion: v1kind: Namespacemetadata: name: monitoring---apiVersion: v1kind: ServiceAccountmetadata: name: monitoring namespace: monitoring---apiVersion: rbac.authorization.k8s.io/v1beta1kind: ClusterRolemetadata: name: monitoring namespace: monitoringrules:- apiGroups: [""] resources: - nodes - nodes/proxy - services - endpoints - pods verbs: ["get", "list", "watch"]- apiGroups: [""] resources: - configmaps verbs: ["get"]- nonResourceURLs: ["/metrics"] verbs: ["get"]---apiVersion: rbac.authorization.k8s.io/v1beta1kind: ClusterRoleBindingmetadata: name: monitoringsubjects: - kind: ServiceAccount name: monitoring namespace: monitoringroleRef: kind: ClusterRole Name: monitoring apiGroup: rbac.authorization.k8s.io---
The above manifest creates the monitoring namespace and service accounts, clusterrole
and clusterrolebinding
needed by Prometheus.
Deploying Prometheus Configuration configmap
apiVersion: v1kind: ConfigMapmetadata: name: prometheus-server-conf labels: name: prometheus-server-conf namespace: monitoringdata: prometheus.yaml.tmpl: |- global: scrape_interval: 5s evaluation_interval: 5s external_labels: cluster: prometheus-ha # Each Prometheus has to have unique labels. replica: $(POD_NAME) rule_files: - /etc/prometheus/rules/*rules.yaml alerting: # We want our alerts to be deduplicated # from different replicas. alert_relabel_configs: - regex: replica action: labeldrop alertmanagers: - scheme: http path_prefix: / static_configs: - targets: ['alertmanager:9093'] scrape_configs: - job_name: kubernetes-nodes-cadvisor scrape_interval: 10s scrape_timeout: 10s scheme: https tls_config: ca_file: /var/run/secrets/kubernetes.io/serviceaccount/ca.crt bearer_token_file: /var/run/secrets/kubernetes.io/serviceaccount/token kubernetes_sd_configs: - role: node relabel_configs: - action: labelmap regex: __meta_kubernetes_node_label_(.+) # Only for Kubernetes ^1.7.3. # See: https://github.com/prometheus/prometheus/issues/2916 - target_label: __address__ replacement: kubernetes.default.svc:443 - source_labels: [__meta_kubernetes_node_name] regex: (.+) target_label: __metrics_path__ replacement: /api/v1/nodes/${1}/proxy/metrics/cadvisor metric_relabel_configs: - action: replace source_labels: [id] regex: '^/machine\.slice/machine-rkt\\x2d([^\\]+)\\.+/([^/]+)\.service$' target_label: rkt_container_name replacement: '${2}-${1}' - action: replace source_labels: [id] regex: '^/system\.slice/(.+)\.service$' target_label: systemd_service_name replacement: '${1}' - job_name: 'kubernetes-pods' kubernetes_sd_configs: - role: pod relabel_configs: - action: labelmap regex: __meta_kubernetes_pod_label_(.+) - source_labels: [__meta_kubernetes_namespace] action: replace target_label: kubernetes_namespace - source_labels: [__meta_kubernetes_pod_name] action: replace target_label: kubernetes_pod_name - source_labels: [__meta_kubernetes_pod_annotation_prometheus_io_scrape] action: keep regex: true - source_labels: [__meta_kubernetes_pod_annotation_prometheus_io_scheme] action: replace target_label: __scheme__ regex: (https?) - source_labels: [__meta_kubernetes_pod_annotation_prometheus_io_path] action: replace target_label: __metrics_path__ regex: (.+) - source_labels: [__address__, __meta_kubernetes_pod_prometheus_io_port] action: replace target_label: __address__ regex: ([^:]+)(?::\d+)?;(\d+) replacement: $1:$2 - job_name: 'kubernetes-apiservers' kubernetes_sd_configs: - role: endpoints scheme: https tls_config: ca_file: /var/run/secrets/kubernetes.io/serviceaccount/ca.crt bearer_token_file: /var/run/secrets/kubernetes.io/serviceaccount/token relabel_configs: - source_labels: [__meta_kubernetes_namespace, __meta_kubernetes_service_name, __meta_kubernetes_endpoint_port_name] action: keep regex: default;kubernetes;https - job_name: 'kubernetes-service-endpoints' kubernetes_sd_configs: - role: endpoints relabel_configs: - action: labelmap regex: __meta_kubernetes_service_label_(.+) - source_labels: [__meta_kubernetes_namespace] action: replace target_label: kubernetes_namespace - source_labels: [__meta_kubernetes_service_name] action: replace target_label: kubernetes_name - source_labels: [__meta_kubernetes_service_annotation_prometheus_io_scrape] action: keep regex: true - source_labels: [__meta_kubernetes_service_annotation_prometheus_io_scheme] action: replace target_label: __scheme__ regex: (https?) - source_labels: [__meta_kubernetes_service_annotation_prometheus_io_path] action: replace target_label: __metrics_path__ regex: (.+) - source_labels: [__address__, __meta_kubernetes_service_annotation_prometheus_io_port] action: replace target_label: __address__ regex: (.+)(?::\d+);(\d+) replacement: $1:$2
The above Configmap creates Prometheus configuration file template. This configuration file template will be read by the Thanos sidecar component and it will generate the actual configuration file, which will in turn be consumed by the Prometheus container running in the same pod. It is extremely important to add the external_labels section in the config file so that the Querier can deduplicate data based on that.
Deploying Prometheus Rules configmap
This will create our alert rules which will be relayed to alertmanager for delivery
apiVersion: v1kind: ConfigMapmetadata: name: prometheus-rules labels: name: prometheus-rules namespace: monitoringdata: alert-rules.yaml: |- groups: - name: Deployment rules: - alert: Deployment at 0 Replicas annotations: summary: Deployment {{$labels.deployment}} in {{$labels.namespace}} is currently having no pods running expr: | sum(kube_deployment_status_replicas{pod_template_hash=""}) by (deployment,namespace) < 1 for: 1m labels: team: devops - alert: HPA Scaling Limited annotations: summary: HPA named {{$labels.hpa}} in {{$labels.namespace}} namespace has reached scaling limited state expr: | (sum(kube_hpa_status_condition{condition="ScalingLimited",status="true"}) by (hpa,namespace)) == 1 for: 1m labels: team: devops - alert: HPA at MaxCapacity annotations: summary: HPA named {{$labels.hpa}} in {{$labels.namespace}} namespace is running at Max Capacity expr: | ((sum(kube_hpa_spec_max_replicas) by (hpa,namespace)) - (sum(kube_hpa_status_current_replicas) by (hpa,namespace))) == 0 for: 1m labels: team: devops - name: Pods rules: - alert: Container restarted annotations: summary: Container named {{$labels.container}} in {{$labels.pod}} in {{$labels.namespace}} was restarted expr: | sum(increase(kube_pod_container_status_restarts_total{namespace!="kube-system",pod_template_hash=""}[1m])) by (pod,namespace,container) > 0 for: 0m labels: team: dev - alert: High Memory Usage of Container annotations: summary: Container named {{$labels.container}} in {{$labels.pod}} in {{$labels.namespace}} is using more than 75% of Memory Limit expr: | ((( sum(container_memory_usage_bytes{image!="",container_name!="POD", namespace!="kube-system"}) by (namespace,container_name,pod_name) / sum(container_spec_memory_limit_bytes{image!="",container_name!="POD",namespace!="kube-system"}) by (namespace,container_name,pod_name) ) * 100 ) < +Inf ) > 75 for: 5m labels: team: dev - alert: High CPU Usage of Container annotations: summary: Container named {{$labels.container}} in {{$labels.pod}} in {{$labels.namespace}} is using more than 75% of CPU Limit expr: | ((sum(irate(container_cpu_usage_seconds_total{image!="",container_name!="POD", namespace!="kube-system"}[30s])) by (namespace,container_name,pod_name) / sum(container_spec_cpu_quota{image!="",container_name!="POD", namespace!="kube-system"} / container_spec_cpu_period{image!="",container_name!="POD", namespace!="kube-system"}) by (namespace,container_name,pod_name) ) * 100) > 75 for: 5m labels: team: dev - name: Nodes rules: - alert: High Node Memory Usage annotations: summary: Node {{$labels.kubernetes_io_hostname}} has more than 80% memory used. Plan Capcity expr: | (sum (container_memory_working_set_bytes{id="/",container_name!="POD"}) by (kubernetes_io_hostname) / sum (machine_memory_bytes{}) by (kubernetes_io_hostname) * 100) > 80 for: 5m labels: team: devops - alert: High Node CPU Usage annotations: summary: Node {{$labels.kubernetes_io_hostname}} has more than 80% allocatable cpu used. Plan Capacity. expr: | (sum(rate(container_cpu_usage_seconds_total{id="/", container_name!="POD"}[1m])) by (kubernetes_io_hostname) / sum(machine_cpu_cores) by (kubernetes_io_hostname) * 100) > 80 for: 5m labels: team: devops - alert: High Node Disk Usage annotations: summary: Node {{$labels.kubernetes_io_hostname}} has more than 85% disk used. Plan Capacity. expr: | (sum(container_fs_usage_bytes{device=~"^/dev/[sv]d[a-z][1-9]$",id="/",container_name!="POD"}) by (kubernetes_io_hostname) / sum(container_fs_limit_bytes{container_name!="POD",device=~"^/dev/[sv]d[a-z][1-9]$",id="/"}) by (kubernetes_io_hostname)) * 100 > 85 for: 5m labels: team: devops
Deploying Prometheus Stateful Set
apiVersion: storage.k8s.io/v1beta1kind: StorageClassmetadata: name: fast namespace: monitoringprovisioner: kubernetes.io/gce-pdallowVolumeExpansion: true---apiVersion: apps/v1beta1kind: StatefulSetmetadata: name: prometheus namespace: monitoringspec: replicas: 3 serviceName: prometheus-service template: metadata: labels: app: prometheus thanos-store-api: "true" spec: serviceAccountName: monitoring containers: - name: prometheus image: prom/prometheus:v2.4.3 args: - "--config.file=/etc/prometheus-shared/prometheus.yaml" - "--storage.tsdb.path=/prometheus/" - "--web.enable-lifecycle" - "--storage.tsdb.no-lockfile" - "--storage.tsdb.min-block-duration=2h" - "--storage.tsdb.max-block-duration=2h" ports: - name: prometheus containerPort: 9090 volumeMounts: - name: prometheus-storage mountPath: /prometheus/ - name: prometheus-config-shared mountPath: /etc/prometheus-shared/ - name: prometheus-rules mountPath: /etc/prometheus/rules - name: thanos image: quay.io/thanos/thanos:v0.8.0 args: - "sidecar" - "--log.level=debug" - "--tsdb.path=/prometheus" - "--prometheus.url=http://127.0.0.1:9090" - "--objstore.config={type: GCS, config: {bucket: prometheus-long-term}}" - "--reloader.config-file=/etc/prometheus/prometheus.yaml.tmpl" - "--reloader.config-envsubst-file=/etc/prometheus-shared/prometheus.yaml" - "--reloader.rule-dir=/etc/prometheus/rules/" env: - name: POD_NAME valueFrom: fieldRef: fieldPath: metadata.name - name : GOOGLE_APPLICATION_CREDENTIALS value: /etc/secret/thanos-gcs-credentials.json ports: - name: http-sidecar containerPort: 10902 - name: grpc containerPort: 10901 livenessProbe: httpGet: port: 10902 path: /-/healthy readinessProbe: httpGet: port: 10902 path: /-/ready volumeMounts: - name: prometheus-storage mountPath: /prometheus - name: prometheus-config-shared mountPath: /etc/prometheus-shared/ - name: prometheus-config mountPath: /etc/prometheus - name: prometheus-rules mountPath: /etc/prometheus/rules - name: thanos-gcs-credentials mountPath: /etc/secret readOnly: false securityContext: fsGroup: 2000 runAsNonRoot: true runAsUser: 1000 volumes: - name: prometheus-config configMap: defaultMode: 420 name: prometheus-server-conf - name: prometheus-config-shared emptyDir: {} - name: prometheus-rules configMap: name: prometheus-rules - name: thanos-gcs-credentials secret: secretName: thanos-gcs-credentials volumeClaimTemplates: - metadata: name: prometheus-storage namespace: monitoring spec: accessModes: [ "ReadWriteOnce" ] storageClassName: fast resources: requests: storage: 20Gi
It is important to understand the following about the manifest provided above:
- Prometheus is deployed as a stateful set with 3 replicas and each replica provisions its own persistent volume dynamically.
- Prometheus configuration is generated by the Thanos sidecar container using the template file we created above.
- Thanos handles data compaction and therefore we need to set –storage.tsdb.min-block-duration=2h and –storage.tsdb.max-block-duration=2h
- Prometheus stateful set is labelled as thanos-store-api: true so that each pod gets discovered by the headless service, which we will create next. It is this headless service which will be used by the Thanos Querier to query data across all Prometheus instances. We also apply the same label to the Thanos Store and Thanos Ruler component so that they are also discovered by the Querier and can be used for querying metrics.
- GCS bucket credentials path is provided using the GOOGLE_APPLICATION_CREDENTIALS environment variable, and the configuration file is mounted to it from the secret which we created as a part of prerequisites.
Deploying Prometheus Services
apiVersion: v1kind: Servicemetadata: name: prometheus-0-service annotations: prometheus.io/scrape: "true" prometheus.io/port: "9090" namespace: monitoring labels: name: prometheusspec: selector: statefulset.kubernetes.io/pod-name: prometheus-0 ports: - name: prometheus port: 8080 targetPort: prometheus---apiVersion: v1kind: Servicemetadata: name: prometheus-1-service annotations: prometheus.io/scrape: "true" prometheus.io/port: "9090" namespace: monitoring labels: name: prometheusspec: selector: statefulset.kubernetes.io/pod-name: prometheus-1 ports: - name: prometheus port: 8080 targetPort: prometheus---apiVersion: v1kind: Servicemetadata: name: prometheus-2-service annotations: prometheus.io/scrape: "true" prometheus.io/port: "9090" namespace: monitoring labels: name: prometheusspec: selector: statefulset.kubernetes.io/pod-name: prometheus-2 ports: - name: prometheus port: 8080 targetPort: prometheus---#This service creates a srv record for querier to find about store-api'sapiVersion: v1kind: Servicemetadata: name: thanos-store-gateway namespace: monitoringspec: type: ClusterIP clusterIP: None ports: - name: grpc port: 10901 targetPort: grpc selector: thanos-store-api: "true"
We create different services for each Prometheus pod in the stateful set, although it is not needed. These are created only for debugging purposes. The purpose of thanos-store-gateway headless service has been explained above. We will later expose Prometheus services using an ingress object.
Deploying Thanos Querier
apiVersion: v1kind: Namespacemetadata: name: monitoring---apiVersion: apps/v1kind: Deploymentmetadata: name: thanos-querier namespace: monitoring labels: app: thanos-querierspec: replicas: 1 selector: matchLabels: app: thanos-querier template: metadata: labels: app: thanos-querier spec: containers: - name: thanos image: quay.io/thanos/thanos:v0.8.0 args: - query - --log.level=debug - --query.replica-label=replica - --store=dnssrv+thanos-store-gateway:10901 ports: - name: http containerPort: 10902 - name: grpc containerPort: 10901 livenessProbe: httpGet: port: http path: /-/healthy readinessProbe: httpGet: port: http path: /-/ready---apiVersion: v1kind: Servicemetadata: labels: app: thanos-querier name: thanos-querier namespace: monitoringspec: ports: - port: 9090 protocol: TCP targetPort: http name: http selector: app: thanos-querier
This is one of the main components of Thanos deployment. Note the following:
- The container argument –store=dnssrv+thanos-store-gateway:10901 helps to discover all components from which metric data should be queried.
- The service thanos-querier provided a web interface to run PromQL queries. It also has the option to de-duplicate data across various Prometheus clusters.
- This is the end point where we provide Grafana as a datasource for all dashboards.
Deploying Thanos Store Gateway
apiVersion: v1kind: Namespacemetadata: name: monitoring---apiVersion: apps/v1beta1kind: StatefulSetmetadata: name: thanos-store-gateway namespace: monitoring labels: app: thanos-store-gatewayspec: replicas: 1 selector: matchLabels: app: thanos-store-gateway serviceName: thanos-store-gateway template: metadata: labels: app: thanos-store-gateway thanos-store-api: "true" spec: containers: - name: thanos image: quay.io/thanos/thanos:v0.8.0 args: - "store" - "--log.level=debug" - "--data-dir=/data" - "--objstore.config={type: GCS, config: {bucket: prometheus-long-term}}" - "--index-cache-size=500MB" - "--chunk-pool-size=500MB" env: - name : GOOGLE_APPLICATION_CREDENTIALS value: /etc/secret/thanos-gcs-credentials.json ports: - name: http containerPort: 10902 - name: grpc containerPort: 10901 livenessProbe: httpGet: port: 10902 path: /-/healthy readinessProbe: httpGet: port: 10902 path: /-/ready volumeMounts: - name: thanos-gcs-credentials mountPath: /etc/secret readOnly: false volumes: - name: thanos-gcs-credentials secret: secretName: thanos-gcs-credentials---
This will create the store component which serves metrics from object storage to the Querier.
Deploying Thanos Ruler
apiVersion: v1kind: Namespacemetadata: name: monitoring---apiVersion: v1kind: ConfigMapmetadata: name: thanos-ruler-rules namespace: monitoringdata: alert_down_services.rules.yaml: | groups: - name: metamonitoring rules: - alert: PrometheusReplicaDown annotations: message: Prometheus replica in cluster {{$labels.cluster}} has disappeared from Prometheus target discovery. expr: | sum(up{cluster="prometheus-ha", instance=~".*:9090", job="kubernetes-service-endpoints"}) by (job,cluster) < 3 for: 15s labels: severity: critical---apiVersion: apps/v1beta1kind: StatefulSetmetadata: labels: app: thanos-ruler name: thanos-ruler namespace: monitoringspec: replicas: 1 selector: matchLabels: app: thanos-ruler serviceName: thanos-ruler template: metadata: labels: app: thanos-ruler thanos-store-api: "true" spec: containers: - name: thanos image: quay.io/thanos/thanos:v0.8.0 args: - rule - --log.level=debug - --data-dir=/data - --eval-interval=15s - --rule-file=/etc/thanos-ruler/*.rules.yaml - --alertmanagers.url=http://alertmanager:9093 - --query=thanos-querier:9090 - "--objstore.config={type: GCS, config: {bucket: thanos-ruler}}" - --label=ruler_cluster="prometheus-ha" - --label=replica="$(POD_NAME)" env: - name : GOOGLE_APPLICATION_CREDENTIALS value: /etc/secret/thanos-gcs-credentials.json - name: POD_NAME valueFrom: fieldRef: fieldPath: metadata.name ports: - name: http containerPort: 10902 - name: grpc containerPort: 10901 livenessProbe: httpGet: port: http path: /-/healthy readinessProbe: httpGet: port: http path: /-/ready volumeMounts: - mountPath: /etc/thanos-ruler name: config - name: thanos-gcs-credentials mountPath: /etc/secret readOnly: false volumes: - configMap: name: thanos-ruler-rules name: config - name: thanos-gcs-credentials secret: secretName: thanos-gcs-credentials---apiVersion: v1kind: Servicemetadata: labels: app: thanos-ruler name: thanos-ruler namespace: monitoringspec: ports: - port: 9090 protocol: TCP targetPort: http name: http selector: app: thanos-ruler
Now if you fire-up on interactive shell in the same namespace as our workloads, and try to see to which all pods does our thanos-store-gateway resolves, you will see something like this:
root@my-shell-95cb5df57-4q6w8:/# nslookup thanos-store-gatewayServer: 10.63.240.10Address: 10.63.240.10#53Name: thanos-store-gateway.monitoring.svc.cluster.localAddress: 10.60.25.2Name: thanos-store-gateway.monitoring.svc.cluster.localAddress: 10.60.25.4Name: thanos-store-gateway.monitoring.svc.cluster.localAddress: 10.60.30.2Name: thanos-store-gateway.monitoring.svc.cluster.localAddress: 10.60.30.8Name: thanos-store-gateway.monitoring.svc.cluster.localAddress: 10.60.31.2root@my-shell-95cb5df57-4q6w8:/# exit
The IP’s returned above correspond to our Prometheus pods, thanos-store
and thanos-ruler
. This can be verified as
$ kubectl get pods -o wide -l thanos-store-api="true"NAME READY STATUS RESTARTS AGE IP NODE NOMINATED NODE READINESS GATESprometheus-0 2/2 Running 0 100m 10.60.31.2 gke-demo-1-pool-1-649cbe02-jdnv <none> <none>prometheus-1 2/2 Running 0 14h 10.60.30.2 gke-demo-1-pool-1-7533d618-kxkd <none> <none>prometheus-2 2/2 Running 0 31h 10.60.25.2 gke-demo-1-pool-1-4e9889dd-27gc <none> <none>thanos-ruler-0 1/1 Running 0 100m 10.60.30.8 gke-demo-1-pool-1-7533d618-kxkd <none> <none>thanos-store-gateway-0 1/1 Running 0 14h 10.60.25.4 gke-demo-1-pool-1-4e9889dd-27gc <none> <none>
Deploying Alertmanager
apiVersion: v1kind: Namespacemetadata: name: monitoring---kind: ConfigMapapiVersion: v1metadata: name: alertmanager namespace: monitoringdata: config.yml: |- global: resolve_timeout: 5m slack_api_url: "<your_slack_hook>" victorops_api_url: "<your_victorops_hook>" templates: - '/etc/alertmanager-templates/*.tmpl' route: group_by: ['alertname', 'cluster', 'service'] group_wait: 10s group_interval: 1m repeat_interval: 5m receiver: default routes: - match: team: devops receiver: devops continue: true - match: team: dev receiver: dev continue: true receivers: - name: 'default' - name: 'devops' victorops_configs: - api_key: '<YOUR_API_KEY>' routing_key: 'devops' message_type: 'CRITICAL' entity_display_name: '{{ .CommonLabels.alertname }}' state_message: 'Alert: {{ .CommonLabels.alertname }}. Summary:{{ .CommonAnnotations.summary }}. RawData: {{ .CommonLabels }}' slack_configs: - channel: '#k8-alerts' send_resolved: true - name: 'dev' victorops_configs: - api_key: '<YOUR_API_KEY>' routing_key: 'dev' message_type: 'CRITICAL' entity_display_name: '{{ .CommonLabels.alertname }}' state_message: 'Alert: {{ .CommonLabels.alertname }}. Summary:{{ .CommonAnnotations.summary }}. RawData: {{ .CommonLabels }}' slack_configs: - channel: '#k8-alerts' send_resolved: true---apiVersion: extensions/v1beta1kind: Deploymentmetadata: name: alertmanager namespace: monitoringspec: replicas: 1 selector: matchLabels: app: alertmanager template: metadata: name: alertmanager labels: app: alertmanager spec: containers: - name: alertmanager image: prom/alertmanager:v0.15.3 args: - '--config.file=/etc/alertmanager/config.yml' - '--storage.path=/alertmanager' ports: - name: alertmanager containerPort: 9093 volumeMounts: - name: config-volume mountPath: /etc/alertmanager - name: alertmanager mountPath: /alertmanager volumes: - name: config-volume configMap: name: alertmanager - name: alertmanager emptyDir: {}---apiVersion: v1kind: Servicemetadata: annotations: prometheus.io/scrape: 'true' prometheus.io/path: '/metrics' labels: name: alertmanager name: alertmanager namespace: monitoringspec: selector: app: alertmanager ports: - name: alertmanager protocol: TCP port: 9093 targetPort: 9093
This will create our alertmanager deployment which will deliver all alerts generated as per Prometheus rules.
Deploying Kubestate Metrics
apiVersion: v1kind: Namespacemetadata: name: monitoring---apiVersion: rbac.authorization.k8s.io/v1 # kubernetes versions before 1.8.0 should use rbac.authorization.k8s.io/v1beta1kind: ClusterRoleBindingmetadata: name: kube-state-metricsroleRef: apiGroup: rbac.authorization.k8s.io kind: ClusterRole name: kube-state-metricssubjects:- kind: ServiceAccount name: kube-state-metrics namespace: monitoring---apiVersion: rbac.authorization.k8s.io/v1# kubernetes versions before 1.8.0 should use rbac.authorization.k8s.io/v1beta1kind: ClusterRolemetadata: name: kube-state-metricsrules:- apiGroups: [""] resources: - configmaps - secrets - nodes - pods - services - resourcequotas - replicationcontrollers - limitranges - persistentvolumeclaims - persistentvolumes - namespaces - endpoints verbs: ["list", "watch"]- apiGroups: ["extensions"] resources: - daemonsets - deployments - replicasets verbs: ["list", "watch"]- apiGroups: ["apps"] resources: - statefulsets verbs: ["list", "watch"]- apiGroups: ["batch"] resources: - cronjobs - jobs verbs: ["list", "watch"]- apiGroups: ["autoscaling"] resources: - horizontalpodautoscalers verbs: ["list", "watch"]---apiVersion: rbac.authorization.k8s.io/v1# kubernetes versions before 1.8.0 should use rbac.authorization.k8s.io/v1beta1kind: RoleBindingmetadata: name: kube-state-metrics namespace: monitoringroleRef: apiGroup: rbac.authorization.k8s.io kind: Role name: kube-state-metrics-resizersubjects:- kind: ServiceAccount name: kube-state-metrics namespace: monitoring---apiVersion: rbac.authorization.k8s.io/v1# kubernetes versions before 1.8.0 should use rbac.authorization.k8s.io/v1beta1kind: Rolemetadata: namespace: monitoring name: kube-state-metrics-resizerrules:- apiGroups: [""] resources: - pods verbs: ["get"]- apiGroups: ["extensions"] resources: - deployments resourceNames: ["kube-state-metrics"] verbs: ["get", "update"]---apiVersion: v1kind: ServiceAccountmetadata: name: kube-state-metrics namespace: monitoring---apiVersion: apps/v1kind: Deploymentmetadata: name: kube-state-metrics namespace: monitoringspec: selector: matchLabels: k8s-app: kube-state-metrics replicas: 1 template: metadata: labels: k8s-app: kube-state-metrics spec: serviceAccountName: kube-state-metrics containers: - name: kube-state-metrics image: quay.io/mxinden/kube-state-metrics:v1.4.0-gzip.3 ports: - name: http-metrics containerPort: 8080 - name: telemetry containerPort: 8081 readinessProbe: httpGet: path: /healthz port: 8080 initialDelaySeconds: 5 timeoutSeconds: 5 - name: addon-resizer image: k8s.gcr.io/addon-resizer:1.8.3 resources: limits: cpu: 150m memory: 50Mi requests: cpu: 150m memory: 50Mi env: - name: MY_POD_NAME valueFrom: fieldRef: fieldPath: metadata.name - name: MY_POD_NAMESPACE valueFrom: fieldRef: fieldPath: metadata.namespace command: - /pod_nanny - --container=kube-state-metrics - --cpu=100m - --extra-cpu=1m - --memory=100Mi - --extra-memory=2Mi - --threshold=5 - --deployment=kube-state-metrics---apiVersion: v1kind: Servicemetadata: name: kube-state-metrics namespace: monitoring labels: k8s-app: kube-state-metrics annotations: prometheus.io/scrape: 'true'spec: ports: - name: http-metrics port: 8080 targetPort: http-metrics protocol: TCP - name: telemetry port: 8081 targetPort: telemetry protocol: TCP selector: k8s-app: kube-state-metrics
Kubestate metrics deployment is needed to relay some important container metrics which are not natively exposed by the kubelet and hence are not directly available to Prometheus.
Deploying Node-Exporter Daemonset
apiVersion: v1kind: Namespacemetadata: name: monitoring---apiVersion: extensions/v1beta1kind: DaemonSetmetadata: name: node-exporter namespace: monitoring labels: name: node-exporterspec: template: metadata: labels: name: node-exporter annotations: prometheus.io/scrape: "true" prometheus.io/port: "9100" spec: hostPID: true hostIPC: true hostNetwork: true containers: - name: node-exporter image: prom/node-exporter:v0.16.0 securityContext: privileged: true args: - --path.procfs=/host/proc - --path.sysfs=/host/sys ports: - containerPort: 9100 protocol: TCP resources: limits: cpu: 100m memory: 100Mi requests: cpu: 10m memory: 100Mi volumeMounts: - name: dev mountPath: /host/dev - name: proc mountPath: /host/proc - name: sys mountPath: /host/sys - name: rootfs mountPath: /rootfs volumes: - name: proc hostPath: path: /proc - name: dev hostPath: path: /dev - name: sys hostPath: path: /sys - name: rootfs hostPath: path: /
Node-Exporter daemonset runs a pod of node-exporter on each node and exposes very important node related metrics which can be pulled by Prometheus instances.
Deploying Grafana
apiVersion: v1kind: Namespacemetadata: name: monitoring---apiVersion: storage.k8s.io/v1beta1kind: StorageClassmetadata: name: fast namespace: monitoringprovisioner: kubernetes.io/gce-pdallowVolumeExpansion: true---apiVersion: apps/v1beta1kind: StatefulSetmetadata: name: grafana namespace: monitoringspec: replicas: 1 serviceName: grafana template: metadata: labels: task: monitoring k8s-app: grafana spec: containers: - name: grafana image: k8s.gcr.io/heapster-grafana-amd64:v5.0.4 ports: - containerPort: 3000 protocol: TCP volumeMounts: - mountPath: /etc/ssl/certs name: ca-certificates readOnly: true - mountPath: /var name: grafana-storage env: - name: GF_SERVER_HTTP_PORT value: "3000" # The following env variables are required to make Grafana accessible via # the kubernetes api-server proxy. On production clusters, we recommend # removing these env variables, setup auth for grafana, and expose the grafana # service using a LoadBalancer or a public IP. - name: GF_AUTH_BASIC_ENABLED value: "false" - name: GF_AUTH_ANONYMOUS_ENABLED value: "true" - name: GF_AUTH_ANONYMOUS_ORG_ROLE value: Admin - name: GF_SERVER_ROOT_URL # If you're only using the API Server proxy, set this value instead: # value: /api/v1/namespaces/kube-system/services/monitoring-grafana/proxy value: / volumes: - name: ca-certificates hostPath: path: /etc/ssl/certs volumeClaimTemplates: - metadata: name: grafana-storage namespace: monitoring spec: accessModes: [ "ReadWriteOnce" ] storageClassName: fast resources: requests: storage: 5Gi---apiVersion: v1kind: Servicemetadata: labels: kubernetes.io/cluster-service: 'true' kubernetes.io/name: grafana name: grafana namespace: monitoringspec: ports: - port: 3000 targetPort: 3000 selector: k8s-app: grafana
This will create our Grafana Deployment and Service which will be exposed using our Ingress Object. We should add Thanos-Querier as the datasource for our Grafana deployment. In order to do so:
- Click on Add DataSource
- Set Name: DS_PROMETHEUS
- Set Type: Prometheus
- Set URL: http://thanos-querier:9090
- Save and Test. You can now build your custom dashboards or simply import dashboards from grafana.net. Dashboard #315 and #1471 are good to start with.
Deploying the Ingress Object
apiVersion: extensions/v1beta1kind: Ingressmetadata: name: monitoring-ingress namespace: monitoring annotations: kubernetes.io/ingress.class: "nginx"spec: rules: - host: grafana.<yourdomain>.com http: paths: - path: / backend: serviceName: grafana servicePort: 3000 - host: prometheus-0.<yourdomain>.com http: paths: - path: / backend: serviceName: prometheus-0-service servicePort: 8080 - host: prometheus-1.<yourdomain>.com http: paths: - path: / backend: serviceName: prometheus-1-service servicePort: 8080 - host: prometheus-2.<yourdomain>.com http: paths: - path: / backend: serviceName: prometheus-2-service servicePort: 8080 - host: alertmanager.<yourdomain>.com http: paths: - path: / backend: serviceName: alertmanager servicePort: 9093 - host: thanos-querier.<yourdomain>.com http: paths: - path: / backend: serviceName: thanos-querier servicePort: 9090 - host: thanos-ruler.<yourdomain>.com http: paths: - path: / backend: serviceName: thanos-ruler servicePort: 9090
This is the final piece in the puzzle. This will help expose all our services outside the Kubernetes cluster and help us access them. Make sure you replace <yourdomain> with a domain name which is accessible to you and you can point the Ingress-Controller’s service to.
You should now be able to access Thanos Querier at http://thanos-querier.<yourdomain>.com . It will look something like this:
Make sure deduplication is selected.
If you click on Stores all the active endpoints discovered by thanos-store-gateway service can be seen
Now you add Thanos Querier as the datasource in Grafana and start creating dashboards
Kubernetes Cluster Monitoring Dashboard
Kubernetes Node Monitoring Dashboard
Conclusion
Integrating Thanos with Prometheus definitely provides the ability to scale Prometheus horizontally, and also since Thanos-Querier is able to pull metrics from other querier instances, you can practically pull metrics across clusters visualize them in a single dashboard.
We are also able to archive metric data in an object store that provides infinite storage to our monitoring system along with serving metrics from the object storage itself. A major part of cost for this set-up can be attributed to the object storage (S3 or GCS). This can be further reduced if we apply appropriate retention policies to them.
However, achieving all this requires quite a bit of configuration on your part. The manifests provided above have been tested in a production environment. Feel free to reach out should you have any questions around them.
Related articles
Subscribe to our newsletter
Get the latest industry trends, exclusive insights, and Gcore updates delivered straight to your inbox.