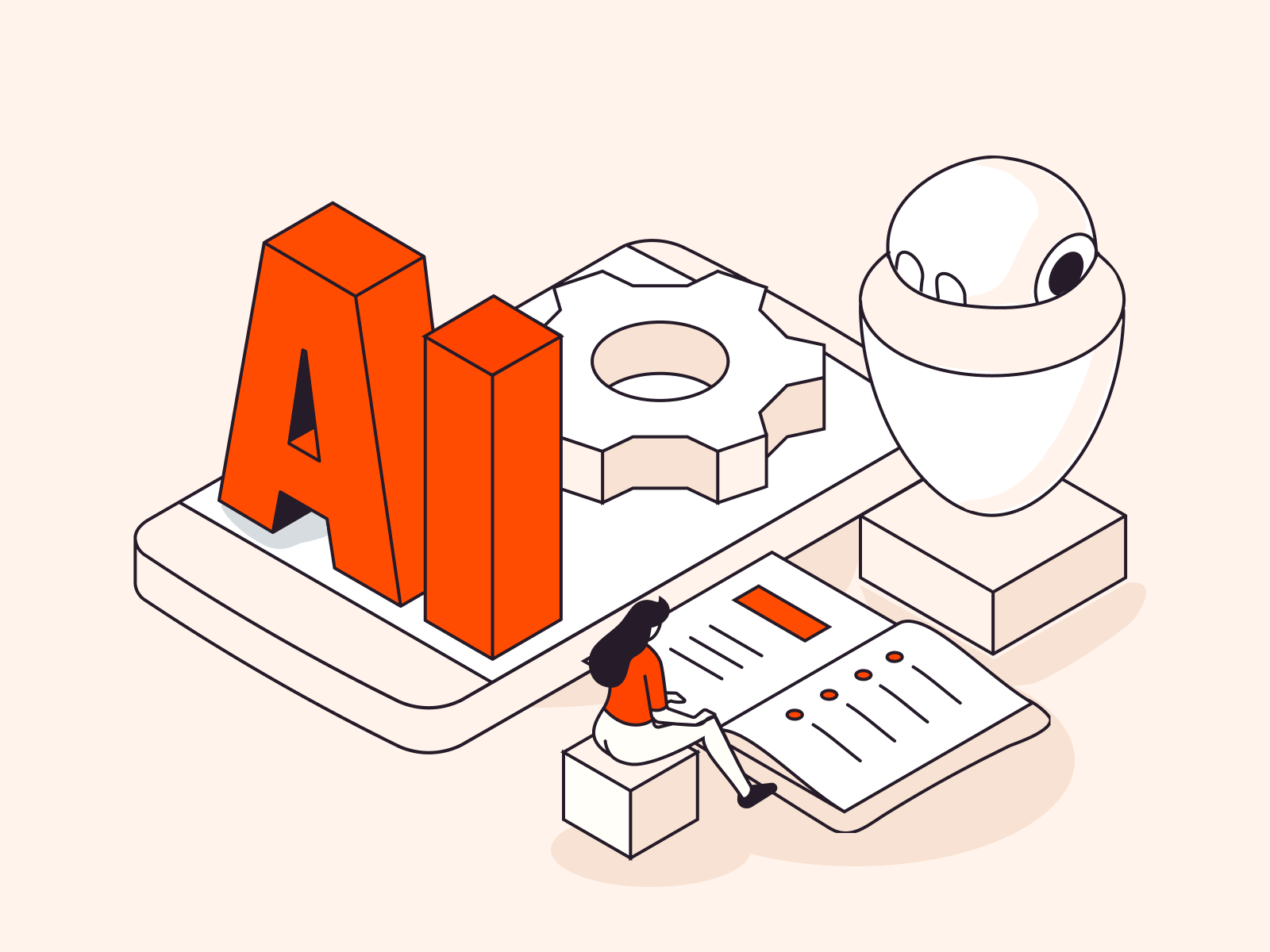
AI frameworks offer a streamlined way to efficiently implement AI algorithms. These frameworks automate many complex tasks required to process and analyze large datasets, facilitating rapid inference processes that enable real-time decision-making. This capability allows companies to respond to market changes with unprecedented speed and accuracy. This article will detail what AI frameworks are, how they work, and how to choose the right AI framework for your needs.
What Is an AI Framework?
AI frameworks are essential tools comprising comprehensive suites of libraries and utilities that support the creation, deployment, and management of artificial intelligence algorithms. These frameworks provide pre-configured functions and modules, allowing developers to focus more on customizing AI models for specific tasks rather than building from scratch.
How AI Frameworks Work
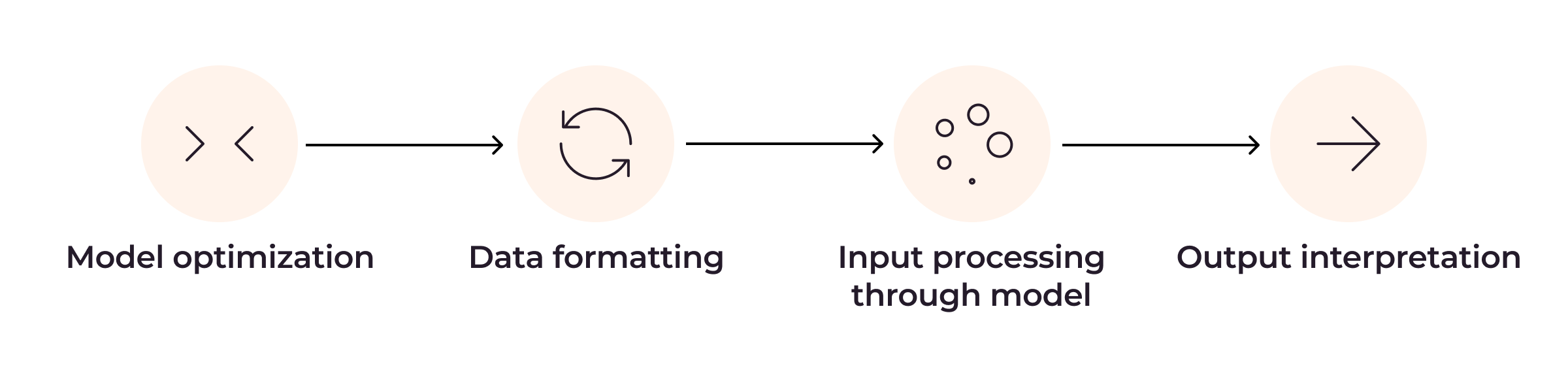
In the inference process, AI frameworks link together several key components: the model, input data, hardware, and the inference engine:
- The framework prepares the trained model for inference, ensuring it’s optimized for the specific type of hardware, whether it’s CPUs (central processing units), GPUs (graphics processing units), TPUs (tensor processing units), or IPUs from Graphcore. This optimization involves adjusting the model’s computational demands to align with the hardware’s capabilities, ensuring efficient processing and reduced latency during inference tasks.
- Before data can be analyzed, the framework formats it to ensure compatibility with the model. This can include normalizing scales, which means adjusting the range of data values to a standard scale to ensure consistency; encoding categorical data, which involves converting text data into a numerical format the model can process; or reshaping input arrays, which means adjusting the data shape to meet the model’s expected input format. Doing so helps maintain accuracy and efficiency in the model’s predictions.
- The framework directs the preprocessed input through the model using the inference engine. For more information, read Gcore’s comprehensive guide to AI inference and how it works.
- Finally, the framework interprets the raw output and translates it into a format that is understandable and actionable. This may include converting logits (the model’s raw output scores) into probabilities, which quantify the likelihood of different outcomes in tasks like image recognition or text analysis. It may also apply thresholding, which sets specific limits to determine the conditions under which certain actions are triggered based on the predictions.
How to Choose the Right AI Framework for Your Inference Needs
The AI framework your organization uses for inference will directly influence the efficiency and effectiveness of its AI initiatives. To make sure that the framework you ultimately select aligns with your organization’s technical needs and business goals, consider several factors, including performance, flexibility, ease of adoption, integration capabilities, cost, and support, in the context of your specific industry and organizational needs.
Performance
In the context of AI frameworks, performance primarily refers to how effectively the framework can manage data and execute tasks, which directly impacts training and inference speeds. High-performance AI frameworks minimize latency, imperative for time-sensitive applications such as automotive AI, where rapid responses to changing road conditions can be a matter of life and death.
That said, different organizations have varying performance requirements and high-performance capabilities can sometimes compromise other features. For example, a framework that prioritizes speed and efficiency might have less flexibility or be harder to use. Additionally, high-performance frameworks may require advanced GPUs and extensive memory allocation, potentially increasing operating costs. As such, make sure to consider the trade-offs between performance and resource consumption; while a high-performance framework like TensorFlow excels in speed and efficiency, its resource demands might not suit all budgets or infrastructure capabilities. Conversely, lighter frameworks like PyTorch might offer less raw speed but greater flexibility and lower resource needs.
Flexibility
Flexibility in an AI framework refers to its capability to test different types of algorithms, adapt to different data types including text, images, and audio, and integrate seamlessly with other technologies. As such, consider whether the framework supports the range of AI methodologies your organization seeks to implement. What types of AI applications do you intend to develop? Can the framework you’re considering grow with your organization’s evolving needs?
In retail, AI frameworks facilitate advanced applications such as smart grocery systems that integrate self-checkout and merchandising. These systems utilize image recognition to accurately identify a wide variety of products and their packaging, which demands a framework that can quickly adapt to different product types without extensive reconfiguration.
Retail environments also benefit from AI frameworks that can process, analyze, and infer from large volumes of consumer data in real time. This capability supports applications that analyze shopper behaviors to generate personalized content, predictions, and recommendations, and use customer service bots integrated with natural language processing to enhance the customer experience and improve operational efficiency.
Ease of Adoption
Ease of AI framework adoption refers to how straightforward it is to implement and use the framework for building AI models. Easy-to-adopt frameworks save valuable development time and resources, making them attractive to startups or teams with limited AI expertise. To assess a particular AI framework’s ease of adoption, determine whether the framework has comprehensive documentation and developer tools. How easily can you learn to use the AI framework for inference?
Renowned for their extensive resources, frameworks like TensorFlow and PyTorch are ideal for implementing AI applications such as generative AI, chatbots, virtual assistants, and data augmentation, where AI is used to create new training examples. Software engineers who use AI tools within a framework that is easy to adopt can save a lot of time and refocus their efforts on building robust, efficient code. Conversely, frameworks like Caffe, although powerful, might pose challenges in adoption due to less extensive documentation and a steeper learning curve.
Integration Capabilities
Integration capabilities refer to the ability of an AI framework to connect seamlessly with a company’s existing databases, software systems, and cloud services. This ensures that AI applications enhance and extend the functionalities of existing systems without causing disruptions, aligning with your chosen provider’s technological ecosystem.
In gaming, AI inference is used in content and map generation, AI bot customization and conversation, and real-time player analytics. In each of these areas, the AI framework needs to integrate smoothly with the existing game software and databases. For content and map generation, the AI needs to work with the game’s design software. For AI bot customization, it needs to connect with the bot’s programming. And for player analytics, it needs to access and analyze data from the game’s database. Prime examples of frameworks that work well for gaming include Unity Machine Learning Agents (ML-Agents), TensorFlow, and Apache MXNet. A well-integrated AI framework will streamline these processes, making sure everything runs smoothly.
Cost
Cost can be a make-or-break factor in the selection process. Evaluate whether the framework offers a cost structure that aligns with your budget and financial goals. It’s also worth considering whether the framework can reduce costs in other areas, such as by minimizing the need for additional hardware or reducing the workload on data scientists through automation. Here, Amazon SageMaker Neo is an excellent choice for organizations already invested in AWS. For those that aren’t, KServe and TensorFlow are good options, due to their open-source nature.
Manufacturing companies often use AI for real-time defect detection in production pipelines. This requires strong AI infrastructure to process and analyze data in real-time, providing rapid response feedback to prevent production bottlenecks.
However, implementing such a system can be expensive. There are costs associated with purchasing the necessary hardware and software, setting up the system, and training employees to use it. Over time, there may be additional costs related to scaling the system as the company grows, maintaining the system to ensure it continues to run efficiently, and upgrading the system to take advantage of new AI developments. Manufacturing companies need to carefully consider whether the long-term cost savings, through improved efficiency and reduced production downtime, outweigh the initial and ongoing costs of the AI infrastructure. The goal is to find a balance that fits within the company’s budget and financial goals.
Support
The level of support provided by the framework vendor can significantly impact your experience and success. Good support includes timely technical assistance, regular updates, and security patches from the selected vendor. You want to make sure that your system stays up-to-date and protected against potential threats. And if an issue arises, you want to know that a responsive support team can help you troubleshoot.
In the hospitality industry, AI frameworks play a key role in enabling services like personalized destination and accommodation recommendations, smart inventory management, and efficiency improvements, important for providing high-quality service and ensuring smooth operations. If an issue arises within the AI framework, it could disrupt the functioning of the recommendation engine or inventory management system, leading to customer dissatisfaction or operational inefficiencies. This is why hospitality businesses need to consider the support provided by the AI framework vendor. A reliable, responsive support team can quickly help resolve any issues, minimizing downtime and maintaining the excellent service quality that guests expect.
How Gcore Inference at the Edge Supports AI Frameworks
Gcore Inference at the Edge is specifically designed to support AI frameworks such as TensorFlow, Keras, PyTorch, PaddlePaddle, ONNX, and Hugging Face, facilitating their deployment across various industries and ensuring efficient inference processes:
- Performance: Gcore Inference at the Edge utilizes high-performance computing resources, including the latest A100 and H100 SXM GPUs. This setup achieves an average latency of just 30 ms through a combination of CDN and Edge Inference technologies, enabling rapid and efficient inference across Gcore’s global network of over 160 locations.
- Flexibility: Gcore supports a variety of AI frameworks, providing the necessary infrastructure to run diverse AI applications. This includes specialized support for Graphcore IPUs and NVIDIA GPUs, allowing organizations to select the most suitable frameworks and hardware based on their computational needs.
- Ease of adoption: With tools like Terraform Provider and REST APIs, Gcore simplifies the integration and management of AI frameworks into existing systems. These features make it easier for companies to adopt and scale their AI solutions without extensive system overhauls.
- Integration capabilities: Gcore’s infrastructure is designed to seamlessly integrate with a broad range of AI models and frameworks, ensuring that organizations can easily embed Gcore solutions into their existing tech stacks.
- Cost: Gcore’s flexible pricing structure helps organizations choose a model that suits their budget and scaling requirements.
- Support: Gcore’s commitment to support encompasses technical assistance, as well as extensive resources and documentation to help users maximize the utility of their AI frameworks. This ensures that users have the help they need to troubleshoot, optimize, and advance their AI implementations.
Gcore Support for TensorFlow vs. Keras vs. PyTorch vs. PaddlePaddle vs. ONNX vs. Hugging Face
As an Inference at the Edge service provider, Gcore integrates with leading AI frameworks for inference. To help you make an informed choice about which AI inference framework best meets your project’s needs, here’s a detailed comparison of features offered by TensorFlow, Keras, PyTorch, PaddlePaddle, ONNX, and Hugging Face, all of which can be used with Gcore Inference at the Edge support.
Parameters | TensorFlow | Keras | PyTorch | PaddlePaddle | ONNX | Hugging Face |
Developer | Google Brain Team | François Chollet (Google) | Facebook’s AI Research lab | Baidu | Facebook and Microsoft | Hugging Face Inc. |
Release Year | 2015 | 2015 | 2016 | 2016 | 2017 | 2016 |
Primary Language | Python, C++ | Python | Python, C++ | Python, C++ | Python, C++ | Python |
Design Philosophy | Large-scale machine learning; high performance; flexibility | User-friendliness; modularity and composability | Flexibility and fluidity for research and development | Industrial-level large-scale application; ease of use | Interoperability; shared optimization | Democratizing AI; NLP |
Core Features | High-performance computation; strong support for large-scale ML | Modular; easy to understand and use to create deep learning models | Dynamic computation graph; native support for Python | Easy to use; support for large-scale applications | Standard format for AI models; supports a wide range of platforms | State-of-the-art NLP models; large-scale model training |
Community Support | Very large | Large | Large | Growing | Growing | Growing |
Documentation | Excellent | Excellent | Good | Good | Good | Good |
Use Case | Research, production | Prototyping, research | Research, production | Industrial level applications | Model sharing, production | NLP research, production |
Model Deployment | TensorFlow Serving, TensorFlow Lite, TensorFlow.js | Keras.js, TensorFlow.js | TorchServe, ONNX | Paddle Serving, Paddle Lite, Paddle.js | ONNX Runtime | Transformers Library |
Pre-Trained Models | Available | Available | Available | Available | Available | Available |
Scalability | Excellent | Good | Excellent | Excellent | Good | Good |
Hardware Support | CPUs, GPUs, TPUs | CPUs, GPUs (via TensorFlow or Theano) | CPUs, GPUs | CPUs, GPUs, FPGA, NPU | CPUs, GPUs (via ONNX Runtime) | CPUs, GPUs |
Performance | High | Moderate | High | High | Moderate to High (depends on runtime environment) | High |
Ease of Learning | Moderate | High | High | High | Moderate | Moderate |
Conclusion
Since 2020, businesses have secured over 4.1 million AI-related patents, highlighting the importance of optimizing AI applications. Driven by the need to enhance performance and reduce latency, companies are actively pursuing the most suitable AI frameworks to maximize inference efficiency and meet specific organizational needs. Understanding the features and benefits of various AI frameworks while considering your business’s specific needs and future growth plans will allow you to make a well-informed decision that optimizes your AI capabilities and supports your long-term goals.
If you’re looking to support your AI inference framework with minimal latency and maximized performance, consider Gcore Inference at the Edge. This solution offers the latest NVIDIA L40S GPUs for superior model performance, a low-latency global network to minimize response times, and scalable cloud storage that adapts to your needs. Additionally, Gcore ensures data privacy and security with GDPR, PCI DSS, and ISO/IEC 27001 compliance, alongside DDoS protection for ML endpoints.