GPU Acceleration in AI: How Graphics Processing Units Drive Deep Learning
- By Gcore
- September 19, 2023
- 9 min read
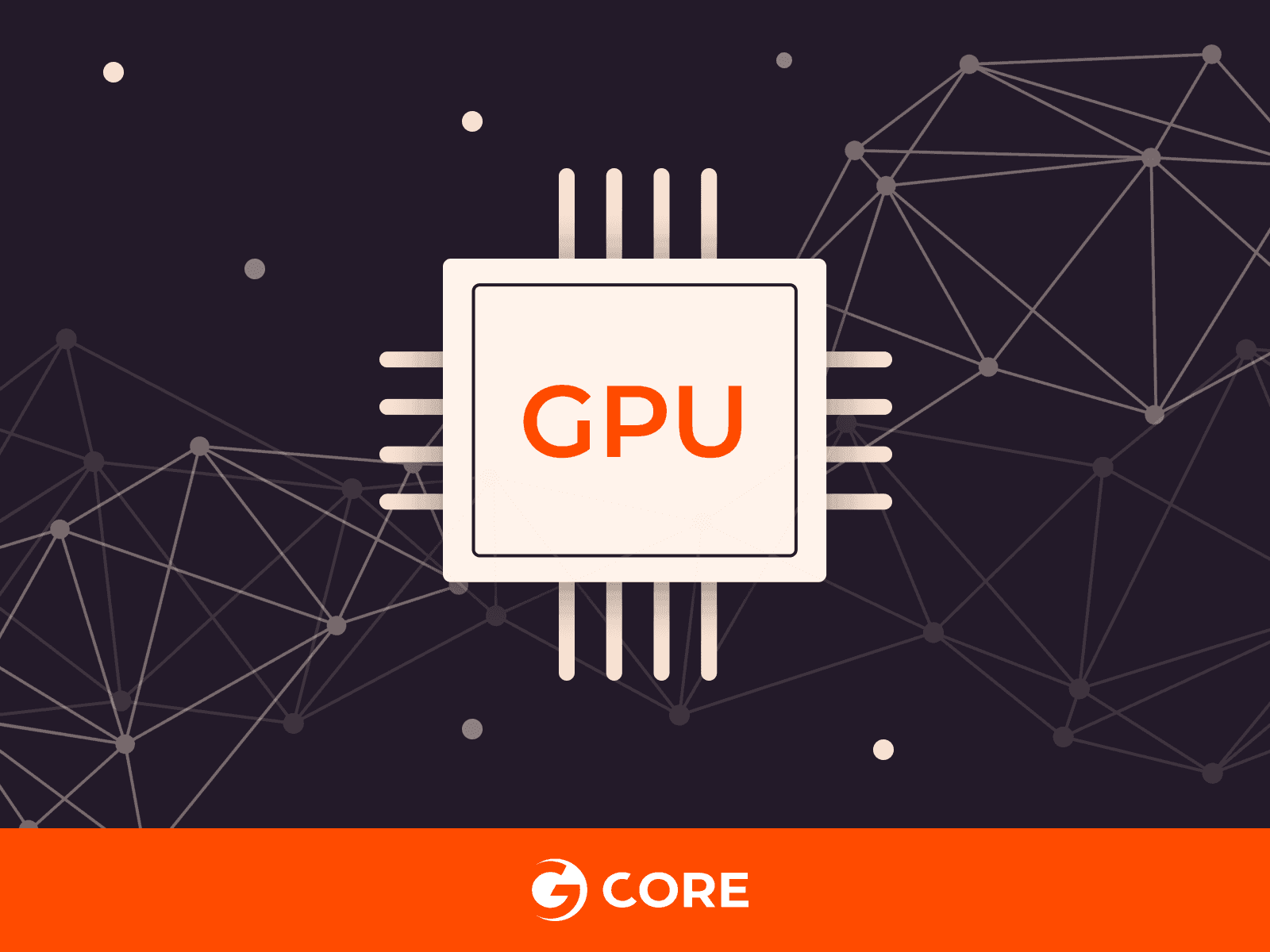
This article discusses how GPUs are shaping a new reality in the hottest subset of AI training: deep learning. We’ll explain the GPU architecture and how it fits with AI workloads, why GPUs are better than CPUs for training deep learning models, and how to choose an optimal GPU configuration.
How GPUs Drive Deep Learning
The key GPU features that power deep learning are its parallel processing capability and, at the foundation of this capability, its core (processor) architecture.
Parallel Processing
Deep learning (DL) relies on matrix calculations, which are performed effectively using the parallel computing that GPUs provide. To understand this interrelationship better, let’s consider a simplified training process of a deep learning model. The model takes the input data, such as images, and has to recognize a specific object in these images using a correlation matrix. The matrix summarizes a data set, identifies patterns, and returns results accordingly: If the object is recognized, the model labels it “true”, otherwise it is labeled “false.” Below is a simplified illustration of this process.
An average DL model has billions of parameters, each of which contributes to the size of the matrix weights used in the matrix calculations. Each of the billion parameters must be taken into account, that’s why the true/false recognition process requires running billions of iterations of the same matrix calculations. The iterations are not linked to each other, they are executed in parallel. GPUs are perfect for handling these types of operations because of their parallel processing capabilities. This is enabled by devoting more transistors to data processing.
Core Architecture: Tensor Cores
NVIDIA tensor cores are an example of how hardware architecture can effectively adapt to DL and AI. Tensor cores—special kinds of processors—were designed specifically for the mathematical calculations needed for deep learning, while earlier cores were also used for video rendering and 3D graphics. “Tensor” refers to tensor calculations, which are matrix calculations. A tensor is a mathematical object; if a tensor has two dimensions, it is a matrix. Below is a visualization of how a Tensor core calculates matrices.
NVIDIA Volta-based chips, like Tesla V100 with 640 tensor cores, became the first fully AI-focused GPUs, and they significantly influenced and accelerated the DL development industry. NVIDIA added tensor cores to its GPU chips in 2017, based on the Volta architecture.
Multi-GPU Clusters
Another GPU feature that drives DL training is the ability to increase throughput by building multi-GPU clusters, where many GPUs work simultaneously. This is especially useful when training large, scalable DL models with billions and trillions of parameters. The most effective approach for such training is to scale GPUs horizontally using interfaces such as NVLink and InfiniBand. These high-speed interfaces allow GPUs to exchange data directly, bypassing CPU bottlenecks.
For example, with the NVLink switch system, you can connect 256 NVIDIA GPUs in a cluster and get 57.6 Tbps of bandwidth. A cluster of that size can significantly reduce the time needed to train large DL models. Though there are several AI-focused GPU vendors on the market, NVIDIA is the undisputed leader, and makes the greatest contribution to DL. This is one of the reasons why Gcore uses NVIDIA chips for its AI GPU infrastructure.
GPU vs. CPU Comparison
A CPU executes tasks serially. Instructions are completed on a first-in, first-out (FIFO) basis. CPUs are better suited for serial task processing because they can use a single core to execute one task after another. CPUs also have a wider range of possible instructions than GPUs and can perform more tasks. They interact with more computer components such as ROM, RAM, BIOS, and input/output ports.
A GPU performs parallel processing, which means it processes tasks by dividing them between multiple cores. The GPU is a kind of advanced calculator: it can only receive a limited set of instructions and execute only graphics- and AI-related tasks, such as matrix multiplication (CPU can execute them too.) GPUs only need to interact with the display and memory. In the context of parallel computing, this is actually a benefit, as it allows for a greater number of cores devoted solely to these operations. This specialization enhances the GPU’s efficiency in parallel task execution.
An average consumer-grade GPU has hundreds of cores adapted to perform simple operations quickly and in parallel, while an average consumer-grade CPU has 2–16 cores adapted to complex sequential operations. Thus, the GPU is better suited for DL because it provides many more cores to perform the necessary computations faster than the CPU.
The parallel processing capabilities of the GPU are made possible by dedicating a larger number of transistors to data processing. Rather than relying on large data caches and complex flow control, GPUs can reduce memory access latencies with computation compared to CPUs. This helps avoid long memory access latencies, frees up more transistors for data processing rather than data caching, and, ultimately, benefits highly parallel computations.
GPUs also use video DRAM: GDDR5 and GDDR6. These are much faster than CPU DRAM: DDR3 and DDR4.
How GPU Outperforms CPU in DL Training
DL requires a lot of data to be transferred between memory and cores. To handle this, GPUs have a specially optimized memory architecture which allows for higher memory bandwidth than CPUs, even when GPUs technically have the same or less memory capacity. For example, a GPU with just 32 GB of HBM (high bandwidth memory) can deliver up to 1.2 Tbps of memory bandwidth and 14 TFLOPS of computing. In contrast, a CPU can have hundreds of GB of HBM, yet deliver only 100 Gbps bandwidth and 1 TFLOPS of computing.
Since GPUs are faster in most DL cases, they can also be cheaper when renting. If you know the approximate time you spend on DL training, you can simply check the prices of cloud providers to estimate how much money you will save by using GPUs instead of CPUs.
Depending on the configuration, models, and frameworks, GPUs often provide better performance than CPUs in DL training. Here are some direct comparisons:
- Azure tested various cloud CPU and GPU clusters using the TensorFlow and Keras frameworks for five DL models of different sizes. In all cases, GPU cluster throughput consistently outperformed CPU cluster throughput, with improvements ranging from 186% to 804%.
- Deci compared the NVIDIA Tesla T4 GPU and the Intel Cascade Lake CPU using the EfficientNet-B2 model. They found that the GPU was 3 times faster than the CPU.
- IEEE published the results of a survey about running different types of neural networks on an Intel i5 9th generation CPU and an NVIDIA GeForce GTX 1650 GPU. When testing CNN (convolutional neural networks,) which are better suited to parallel computation, the GPU was between 4.9 and 8.8 times faster than the CPU. But when testing ANN (artificial neural networks,) the execution time of CPUs was 1.2 times faster than that of GPUs. However, GPUs outperformed CPUs as the data size increased, regardless of the NN architecture.
Using CPU for DL Training
The last comparison case shows that CPUs can sometimes be used for DL training. Here are a few more examples of this:
- There are CPUs with 128 cores that can process some AI workloads faster than consumer GPUs.
- Some algorithms allow the optimization DL model to perform better training on CPUs. For instance, Rice’s Brown School of Engineering has introduced an algorithm that makes CPUs 15 times faster than GPUs for some AI tasks.
- There are cases where the precision of a DL model is not critical, like speech recognition under near-ideal conditions without any noise and interference. In such situations, you can train a DL model using floating-point weights (FP16, FP32) and then round them to integers. Because CPUs work better with integers than GPUs, they can be faster, although the results will not be as accurate.
However, using CPUs for DL training is still an unusual practice. Most DL models are adapted for parallel computing, i.e., for GPU hardware. Thus, building a CPU-based DL platform is a task that may be both difficult and unnecessary. It can take an unpredictable amount of time to select a multi-core CPU instance and then configure a CPU-adapted algorithm to train your model. By selecting a GPU instance, you get a platform that’s ready to build, train, and run your DL model.
How to Choose an Optimal GPU Configuration for Deep Learning
Choosing the optimal GPU configuration is basically a two-step process:
- Determine the stage of deep learning you need to execute.
- Choose a GPU server specification to match.
Note: We’ll only consider specification criteria for DL training, because DL inference (execution of a trained DL model,) as you’ll see, is not such a big deal as training.
1. Determine Which Stage of Deep Learning You Need
To choose an optimal GPU configuration, first you must understand which of two main stages of DL you will execute on GPUs: DL training, or DL inference. Training is the main challenge of DL, because you have to adjust the huge number (up to trillions) of matrix coefficients (weights.) The process is close to a brute-force search for the best combinations to give the best results (though some techniques help to reduce the number of computations, for example, the stochastic gradient descent algorithm.) Therefore, you need maximum hardware performance for training, and vendors make GPUs specifically designed for this. For example, the NVIDIA A100 and H100 GPUs are positioned as devices for DL training, not for inference.
Once you have calculated all the necessary matrix coefficients, the model is trained and ready for inference. At this stage, a DL model only needs to multiply the input data and the matrix coefficients once to produce a single result—for example, when a text-to-image AI generator generates an image according to a user’s prompt. Therefore, inference is always simpler than training in terms of math computations and required computational resources. In some cases, DL inference can be run on desktop GPUs, CPUs, and smartphones. An example is an iPhone with face recognition: the relatively modest GPU with 4–5 cores is sufficient for DL inference.
2. Choose the GPU Specification for DL Training
When choosing a GPU server or virtual GPU instance for DL training, it’s important to understand what training time is appropriate for you: hours, days, months, etc. To achieve this, you can count operations in the model or use information about reported training time and GPU model performance. Then, decide on the resources you need:
- Memory size is a key feature. You need to specify at least as much GPU RAM as your DL model size. This is sufficient if you are not pressed for time to market, but if you’re under time pressure then it’s better to specify sufficient memory plus extra in reserve.
- The number of tensor cores is less critical than the size of the GPU memory, since it only affects the computation speed. However, if you need to train a model faster, then the more cores the better.
- Memory bandwidth is critical if you need to scale GPUs horizontally, for example, when the training time is too long, the dataset is huge, or the model is highly complex. In such cases, check whether the GPU instances support interconnects, such as NVLink or InfiniBand.
So, memory size is the most important thing when training a DL model: if you don’t have enough memory, you won’t be able to run the training. For example, to run the LLaMA model with 7 billion parameters at full precision, the Hugging Face technical team suggests using 28 GB of GPU RAM. This is the result of multiplying 7×4, where 7 is the tensor size (7B), and 4 is four bits for FP32 (the full-precision format.) For FP16 (half-precision), 14 GB is enough (7×2.) The full-precision format provides greater accuracy. The half-precision format provides less accuracy but makes training faster and more memory efficient.
Kubernetes as a Tool for Improving DL Inference
To improve DL inference, you can containerize your model and use a managed Kubernetes service with GPU instances as worker nodes. This will help you achieve greater scalability, resiliency, and cost savings. With Kubernetes, you can automatically scale resources as needed. For example, if the number of user prompts to your model spikes, you will need more compute resources for inference. In that case, more GPUs are allocated for DL inference only when needed, meaning you have no idle resources and no monetary waste.
Managed Kubernetes also reduces operational overhead and helps to automate cluster maintenance. A provider manages master nodes (the control plane.) You manage only the worker nodes on which you deploy your model, instead focusing on its development.
AI Frameworks that Power Deep Learning on GPUs
Various free, open-source AI frameworks help to train deep neural networks and are specifically designed to be run on GPU instances. All of the following frameworks also support NVIDIA’s Compute Unified Device Architecture (CUDA.) This is a parallel computing platform and API that enables the development of GPU-accelerated applications, including DL models. CUDA can significantly improve their performance.
TensorFlow is a library for ML and AI focused on deep learning model training and inference. With TensorFlow, developers can create dataflow graphs. Each graph node represents a matrix operation, and each connection between nodes is a matrix (tensor.) TensorFlow can be used with several programming languages, including Python, C++, JavaScript, and Java.
PyTorch is a machine-learning framework based on the Torch library. It provides two high-level features: tensor computing with strong acceleration via GPUs, and deep neural networks built on a tape-based auto-differentiation system. PyTorch is considered more flexible than TensorFlow because it gives developers more control over the model architecture.
MXNet is a portable and lightweight DL framework that can be used for DL training and inference not only on GPUs, but also on CPUs and TPUs (Tensor Processing Units.) MXNet supports Python, C++, Scala, R, and Julia.
PaddlePaddle is a powerful, scalable, and flexible framework that, like MXNet, can be used to train and deploy deep neural networks on a variety of devices. PaddlePaddle provides over 500 algorithms and pretrained models to facilitate rapid DL development.
Gcore’s Cloud GPU Infrastructure
As a cloud provider, Gcore offers AI GPU Infrastructure powered by NVIDIA chips:
- Virtual machines and bare metal servers with consumer- and enterprise-grade GPUs
- AI clusters based on servers with A100 and H100 GPUs
- Managed Kubernetes with virtual and physical GPU instances that can be used as worker nodes
With Gcore’s GPU infrastructure, you can train and deploy DL models of any type and size. To learn more about our cloud services and how they can help in your AI journey, contact our team.
Conclusion
The unique design of GPUs, focused on parallelism and efficient matrix operations, makes them the perfect companion for the AI challenges of today and tomorrow, including deep learning. Their profound advantages over CPUs are underscored by their computational efficiency, memory bandwidth, and throughput capabilities.
When seeking a GPU, consider your specific deep learning goals, time, and budget. These help you to choose an optimal GPU configuration.
Related articles
Subscribe to our newsletter
Get the latest industry trends, exclusive insights, and Gcore updates delivered straight to your inbox.