Stable Diffusion: Open-source AI for image generation and the amazing apps based on this tech
- By Gcore
- February 2, 2023
- 4 min read
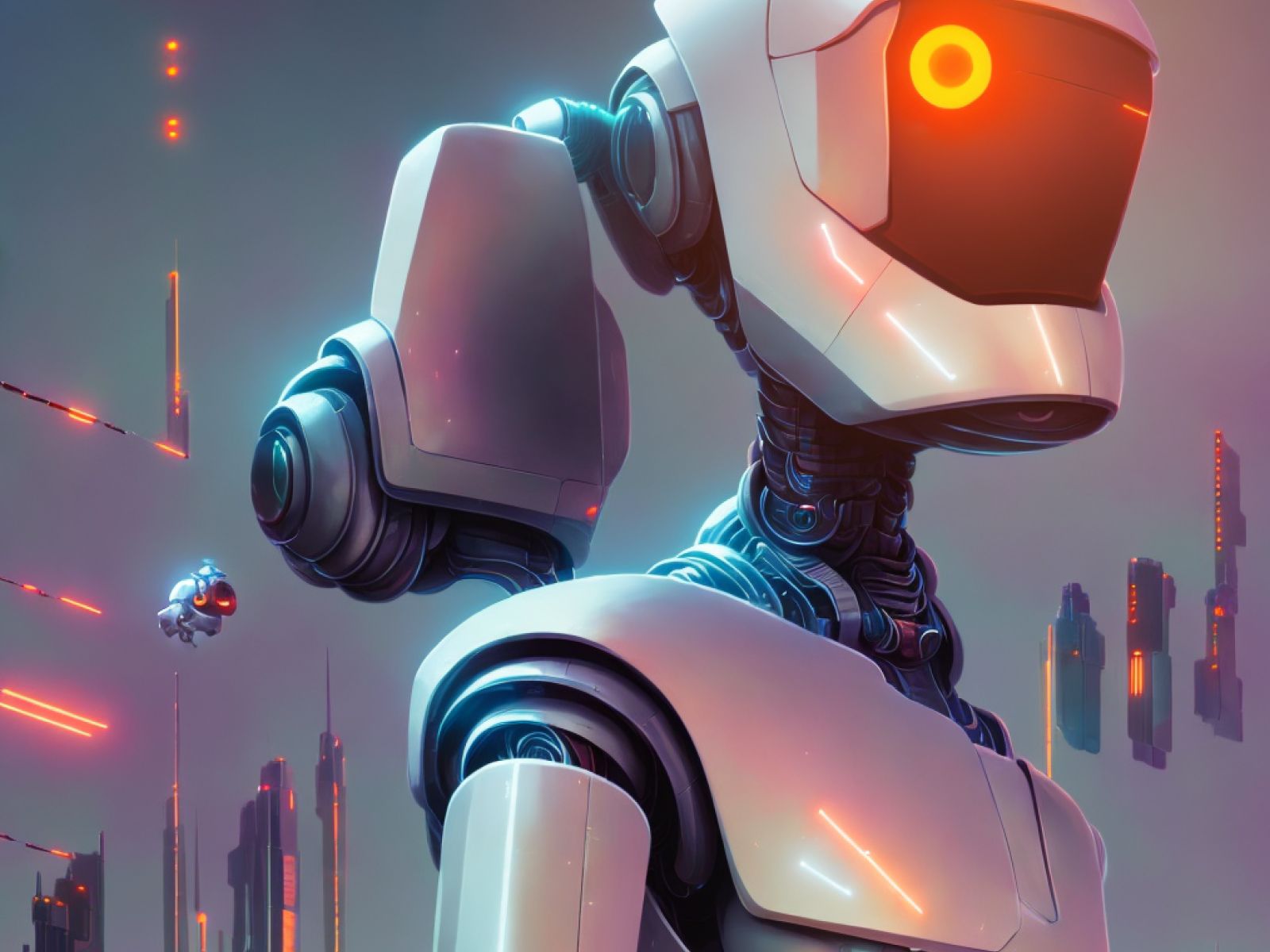
In 2022, image generation technology truly reached new heights. Tools like Open AI’s DALL·E 2 achieved a level that could seriously compete with humans—which even led to artists’ mass protest against AI-generated artworks. That year, we saw groundbreaking advancements in the field, and it’s clear that the future of image generation is looking brighter than ever before.
In this article, we’ll talk about the lesser-known model for image generation—Stable Diffusion. This is a customizable open-source tool. We will tell you how this model favorably differs from DALL·E 2 and show some of the amazing applications created based on its capabilities.
DALL·E 2: Opportunities and drawbacks
When it comes to neural networks that have made a significant impact, one that certainly comes to mind is DALL·E 2. This AI has achieved stunning results and gained wide popularity: by September of 2022, it had reached 1.5M users creating over 2M images per day.
DALL·E 2 generates images based on a text description. This opens up a whole world of possibilities, from creating fantastical creatures to more realistic objects with subtle variations.
You can request what you want to see and get the response in a few seconds. For example, let’s try inputting “portrait of Thor from Avengers, slight smile, diffuse natural sunlight, autumn lights, highly detailed, digital painting, artstation, concept art, sharp focus, illustration.” Here is one result DALL·E 2 will generate:
The AI is also able to perform image inpainting and outpainting. Inpainting is the process of filling in missing or corrupted parts of an image, while outpainting is the process of extending an image beyond its original boundaries. Here is an example of what outpainting looks like:
DALL·E 2 looks like a tool that opens up unlimited potential. However, it actually has a significant drawback—DALL·E 2 is restricted to the specific dataset it’s been trained on. This greatly limits the application of the AI. DALL·E 2 will never create a portrait of you or generate a design for your apartment since it can’t add photos of you or the apartment into the dataset. It is also worth noting that DALL·E 2 is a “black box,” meaning that we can’t modify its internal mechanisms.
How Stable Diffusion & DreamBooth solve the DALL·E 2 problem
The problem with customization was solved by Stability AI’s Stable Diffusion in conjunction with Google’s DreamBooth method. Stable Diffusion is an image generation neural network that is similar to DALL·E 2 but open-source. It can be easily trained and fine-tuned.
About Stable Diffusion
Stable Diffusion accepts text descriptions, also known as “prompts,” as input. You have the ability to specify a specific location, weather conditions, and time of day. Additionally, you can even specify the style of a renowned artist or a person’s hairstyle, among other details. In short, the possibilities are endless with the level of customization you can include in your request.
Stable Diffusion produces results as good as DALL·E 2. Here is its version of Thor:
The request to Stable Diffusion: “portrait of Thor from Avengers, slight smile, diffuse natural sunlight, autumn lights, highly detailed, digital painting, artstation, concept art, sharp focus, illustration.”
Just like DALL·E 2, Stable Diffusion is trained on generic datasets. This means that, in the base version, the AI cannot know your face as a specific person or some specific object styles, etc., which are not provided to the base dataset.
Stable Diffusion fine-tuning
DreamBooth is a technique that helps to customize text-to-image models like Stable Diffusion by enriching the model’s dataset with new data. Here is how it works:
- You upload new images and keywords attached to them.
- DreamBooth helps to train the model on the uploaded data.
- The model creates associations between the new keywords and images.
- The model can now create images based on your new data.
For example, you can upload 20 photos of a person and add one keyword to all of them. Let’s say the person is John Smith and you use his name as a keyword. As a result of additional training, Stable Diffusion learns how John Smith looks and can now create images built around him. If you request “John Smith in a sports car” or “John Smith as Spider-Man,” the model will understand what you mean and create the image.
DreamBooth helps to get a personalized ML model trained on your data. It can be any type of image: photos, game assets, logos, paintings, and many more.
Use cases: Amazing apps based on Stable Diffusion
To give you an idea of just how cool the combination of Stable Diffusion and DreamBooth is, we will present a few examples of amazing tools based on the tech that are already available.
AI avatar generation
portret.ai generates realistic avatars based on photos uploaded by you. AI will create an image of you according to the text description—for instance, it can portray you in the desert or make you an astronaut.
2D game assets
Scenario helps generate hundreds of variations of game assets. You just need to upload your own training data: characters, props, vehicles, weapons, skins, buildings, concept art, pixel art, sketches, etc. Scenario will create assets in the style of your game.
AI photo stock
StockAI creates realistic images generated by AI. It uses a trained version of Stable Diffusion which focuses on realistic photo photos of people and real-world objects.
Hairstyle selection
HairstyleAI will create dozens of hairstyle options matched with your face. All you need to do is upload photos and vòila!—you will be able to choose a new hairstyle.
AI interior
InteriorAI makes design variations for the inside of your home in no time. It just needs photos of your house or apartment to generate fresh ideas for you to consider.
NSFW content generation
SimpMaker3K1 is a public version of Stable Diffusion trained to generate nude content. It creates images of naked people from scratch (we won’t show examples of this, but they are available on the developer’s website). And can also produce anime/fantasy images with a hint of realism.
Conclusion and perspectives
For years, image generators have been part of the technological landscape. But only recently has this technology become sufficiently mature to have an impact on our world. Today we can generate high-quality images from scratch just by typing out text. This will revolutionize industries such as fashion, gaming, design, and many others. In addition, we can generate synthetic data to train other neural networks—this opens up new possibilities for improving AI models.
It’s important to note that any advanced technology can be used for both good and bad purposes. And this technology is no exception—it will facilitate a new generation of fake images, there is no escaping that fact. Nevertheless, we hope that this will not damage the reputation of AI tools and that most people will choose to use them for good.
Related articles
Subscribe to our newsletter
Get the latest industry trends, exclusive insights, and Gcore updates delivered straight to your inbox.