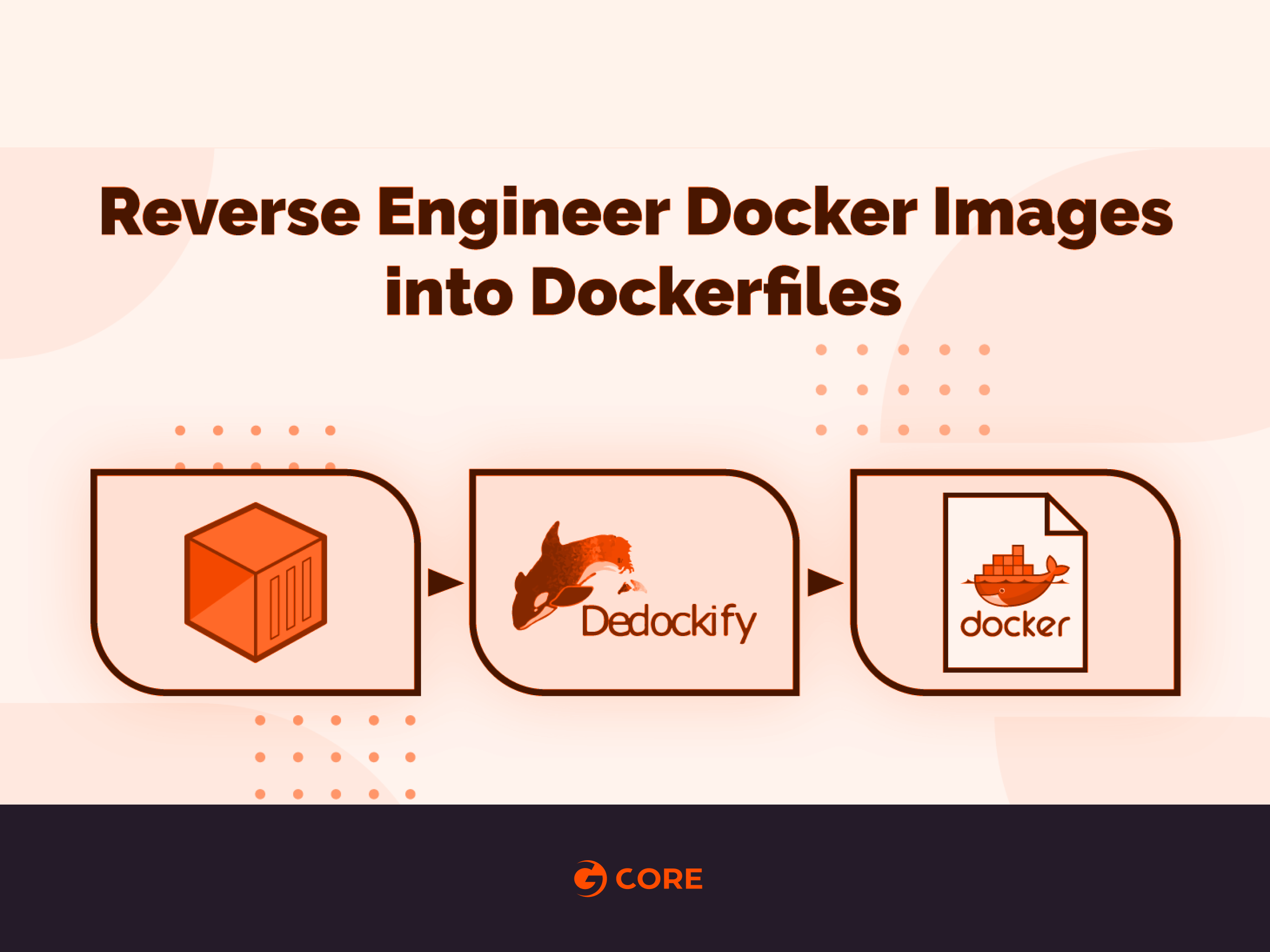
This article explores how we can reverse engineer Docker images by examining the internals of how Docker images store data, how to use tools to examine the different aspects of the image, and how we can create tools like Dedockify to leverage the Python Docker API to create Dockerfiles from source images.
Introduction
As public Docker registries like Docker Hub and TreeScale increase in popularity, except for the most restrictive environments, it has become common for admins and developers to casually download an image built by an unknown entity. It often comes down to the convivence outweighing the perceived risk. When a Docker image is made publicly available, the Dockerfile is sometimes also provided, either directly in the listing, in a git repository, or through an associated link, but sometimes this is not the case. Even if the Dockerfile was made available, we don’t have many assurances that the published image is safe to use.
Maybe security vulnerabilities aren’t your concern. Perhaps one of your favorite images is no longer being maintained, and you would like to update it so that it runs on the latest version of Ubuntu. Or perhaps a compiler for another distribution has an exclusive feature that makes it better optimized to produce binaries during compile time, and you have an uncontrollable compulsion to release a similar image that’s just a little more optimized.
Whatever the reason, if you wish to recover a Dockerfile from an image, there are options. Docker images aren’t a black box. Often, you can retrieve most of the information you need to reconstruct a Dockerfile. In this article, we will explore exactly how to do that by looking inside a Docker image so that we can very closely reconstruct the Dockerfile that built it.
In this article, we will show how it’s possible to reconstruct a Dockerfile from an image using two tools, Dedockify
, a customized Python script provided for this article, and dive
. The basic process flow used will be as follows.

Using dive
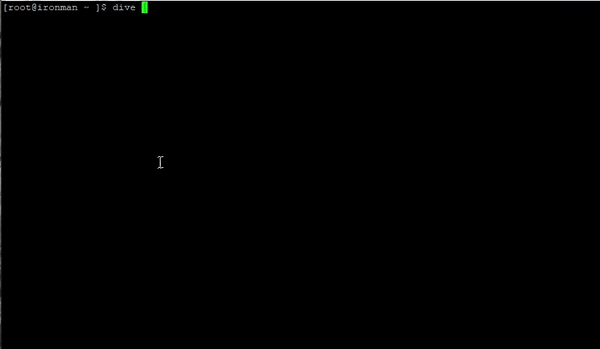
To get some quick, minimal-effort intuition regarding how images are composed, we will introduce ourselves to various advanced and potentially unfamiliar Docker concepts using Dive. Dive is an image exploration tool that allows examination of each layer of a Docker image.
First, let us create a simple, easy to follow Dockerfile that we can explore for testing purposes.
In an empty directory, enter the following snippet directly into the command line:
cat > Dockerfile << EOF ; touch testfile1 testfile2 testfile3 FROM scratch COPY testfile1 / COPY testfile2 / COPY testfile3 / EOF
By entering the above and pressing enter, we’ve just created a new Dockerfile
and populated three zero-byte test files in the same directory.
$ ls Dockerfile testfile1 testfile2 testfile3
So now, lets build an image using this Dockerfile and tag it as example1
.
docker build . -t example1
Building the example1
image should produce the following output:
Sending build context to Docker daemon 3.584kB Step 1/4 : FROM scratch ---> Step 2/4 : COPY testfile1 / ---> a9cc49948e40 Step 3/4 : COPY testfile2 / ---> 84acff3a5554 Step 4/4 : COPY testfile3 / ---> 374e0127c1bc Successfully built 374e0127c1bc Successfully tagged example1:latest
The following zero-byte example1
image should now be available:
$ docker images REPOSITORY TAG IMAGE ID CREATED SIZE example1 latest 374e0127c1bc 31 seconds ago 0B
Note that since there’s no binary data, this image won’t be functional. We are only using it as a simplified example of how layers can be viewed in Docker images.
We can see here by the size of the image that there is no source image. Instead of a source image, we used scratch
which instructed Docker to use a zero-byte blank image as the source image. We then modified the blank image by copying three additional zero-byte test files onto it, and then tagged the changes as example1
.
Now, let us explore our new image with Dive.
docker run --rm -it \ -v /var/run/docker.sock:/var/run/docker.sock \ wagoodman/dive:latest example1
Executing the above command should automatically pull wagoodman/dive
from Docker Hub, and produce the output of Dive’s polished interface.
Unable to find image 'wagoodman/dive:latest' locally latest: Pulling from wagoodman/dive 89d9c30c1d48: Pull complete 5ac8ae86f99b: Pull complete f10575f61141: Pull complete Digest: sha256:2d3be9e9362ecdcb04bf3afdd402a785b877e3bcca3d2fc6e10a83d99ce0955f Status: Downloaded newer image for wagoodman/dive:latest Image Source: docker://example-image Fetching image... (this can take a while for large images) Analyzing image... Building cache...
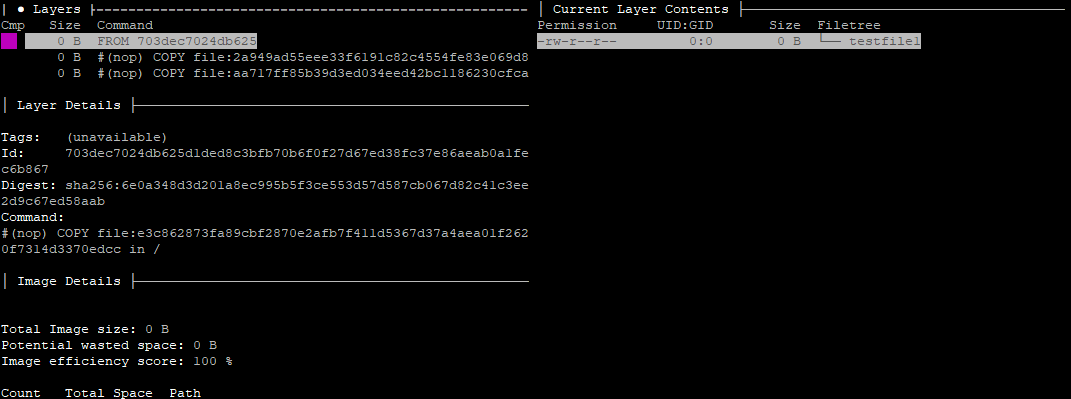
Scroll through the three layers of the image in the list to find the three files in the tree displayed on the right.
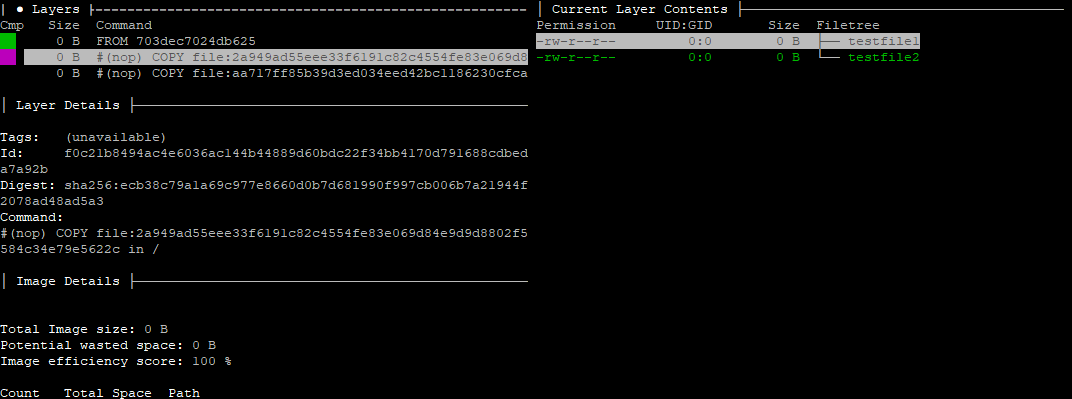
We can see the contents on the right change as we scroll through each layer. As each file was copied to a blank Docker scratch
image, it was recorded as a new layer.
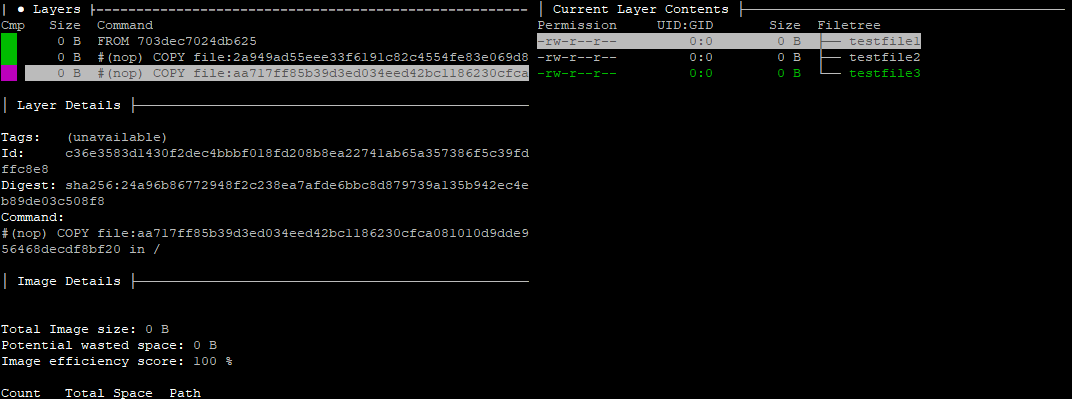
Notice also that we can see the commands that were used to produced each layer. We can also see the hash value of the source file and the file that was updated.
If we take note of the items in the Command:
section, we should see the following:
#(nop) COPY file:e3c862873fa89cbf2870e2afb7f411d5367d37a4aea01f2620f7314d3370edcc in / #(nop) COPY file:2a949ad55eee33f6191c82c4554fe83e069d84e9d9d8802f5584c34e79e5622c in / #(nop) COPY file:aa717ff85b39d3ed034eed42bc1186230cfca081010d9dde956468decdf8bf20 in /
Each command provides solid insight into the original command used in the Dockerfile to produce the image. However, the original filename is lost. It appears that the only way to recover this information is to make observations about the changes to the target filesystem, or perhaps to infer based on other details. More on this later.
docker history
Aside from third-party tools like dive
, the tool we have immediately available is docker history
. If we use the docker history
command on our example1
image, we can view the entries we used in the Dockerfile to create that image.
docker history example1
We should, therefore, get the following result:
IMAGE CREATED CREATED BY SIZE COMMENT 374e0127c1bc 25 minutes ago /bin/sh -c #(nop) COPY file:aa717ff85b39d3ed… 0B 84acff3a5554 25 minutes ago /bin/sh -c #(nop) COPY file:2a949ad55eee33f6… 0B a9cc49948e40 25 minutes ago /bin/sh -c #(nop) COPY file:e3c862873fa89cbf… 0B
Notice that everything in the CREATED BY
column is truncated. These are Dockerfile directives passed through Bourne shell. This information could be useful for recreating our Dockerfile, and although it is truncated here, we can view all of it by also using the --no-trunc
option:
$ docker history example1 --no-trunc IMAGE CREATED CREATED BY SIZE COMMENT sha256:374e0127c1bc51bca9330c01a9956be163850162f3c9f3be0340bb142bc57d81 29 minutes ago /bin/sh -c #(nop) COPY file:aa717ff85b39d3ed034eed42bc1186230cfca081010d9dde956468decdf8bf20 in / 0B sha256:84acff3a5554aea9a3a98549286347dd466d46db6aa7c2e13bb77f0012490cef 29 minutes ago /bin/sh -c #(nop) COPY file:2a949ad55eee33f6191c82c4554fe83e069d84e9d9d8802f5584c34e79e5622c in / 0B sha256:a9cc49948e40d15166b06dab42ea0e388f9905dfdddee7092f9f291d481467fc 29 minutes ago /bin/sh -c #(nop) COPY file:e3c862873fa89cbf2870e2afb7f411d5367d37a4aea01f2620f7314d3370edcc in / 0B
While this has some useful data, it could be a challenge to parse from the command line. We could also use docker inspect
. However, in this article, we will focus on using the Docker Engine API for Python.
Using Docker Engine API for Python
Docker released a Python library for the Docker Engine API, which allows full control of Docker from within Python. In the following example, we can recover similar information we did using docker history
by running the following Python 3 code:
#!/usr/bin/python3 import docker cli = docker.APIClient(base_url='unix://var/run/docker.sock') print (cli.history('example1'))
This should result in output much like the following:
[{'Comment': '', 'Created': 1583008507, 'CreatedBy': '/bin/sh -c #(nop) COPY file:aa717ff85b39d3ed034eed42bc1186230cfca081010d9dde956468decdf8bf20 in / ', 'Id': 'sha256:374e0127c1bc51bca9330c01a9956be163850162f3c9f3be0340bb142bc57d81', 'Size': 0, 'Tags': ['example:latest']}, {'Comment': '', 'Created': 1583008507, 'CreatedBy': '/bin/sh -c #(nop) COPY file:2a949ad55eee33f6191c82c4554fe83e069d84e9d9d8802f5584c34e79e5622c in / ', 'Id': 'sha256:84acff3a5554aea9a3a98549286347dd466d46db6aa7c2e13bb77f0012490cef', 'Size': 0, 'Tags': None}, {'Comment': '', 'Created': 1583008507, 'CreatedBy': '/bin/sh -c #(nop) COPY file:e3c862873fa89cbf2870e2afb7f411d5367d37a4aea01f2620f7314d3370edcc in / ', 'Id': 'sha256:a9cc49948e40d15166b06dab42ea0e388f9905dfdddee7092f9f291d481467fc', 'Size': 0, 'Tags': None}]
Looking at the output, we can see that reconstructing much of the Dockerfile is just a matter of parsing all the relevant data and reversing the entries. But as we saw earlier, we also notice that there are a few hashed entries in the COPY
directives. As previously mentioned, the hashed entries here represent filenames used from outside the layer. This information isn’t directly recoverable. However, just as we saw in dive
, we can infer these names when we search for changes made to the layer. It’s also sometimes possible to infer in cases where the original copy directive included the target filename as the destination. In other cases, the filenames may not be critical, allowing us to use arbitrary filenames. And still in other cases, while more difficult to assess, we can infer filenames that are back-referenced elsewhere in the system, such as in supporting dependencies like scripts or configuration files. But in any case, searching for all changes between layers is the most reliable.
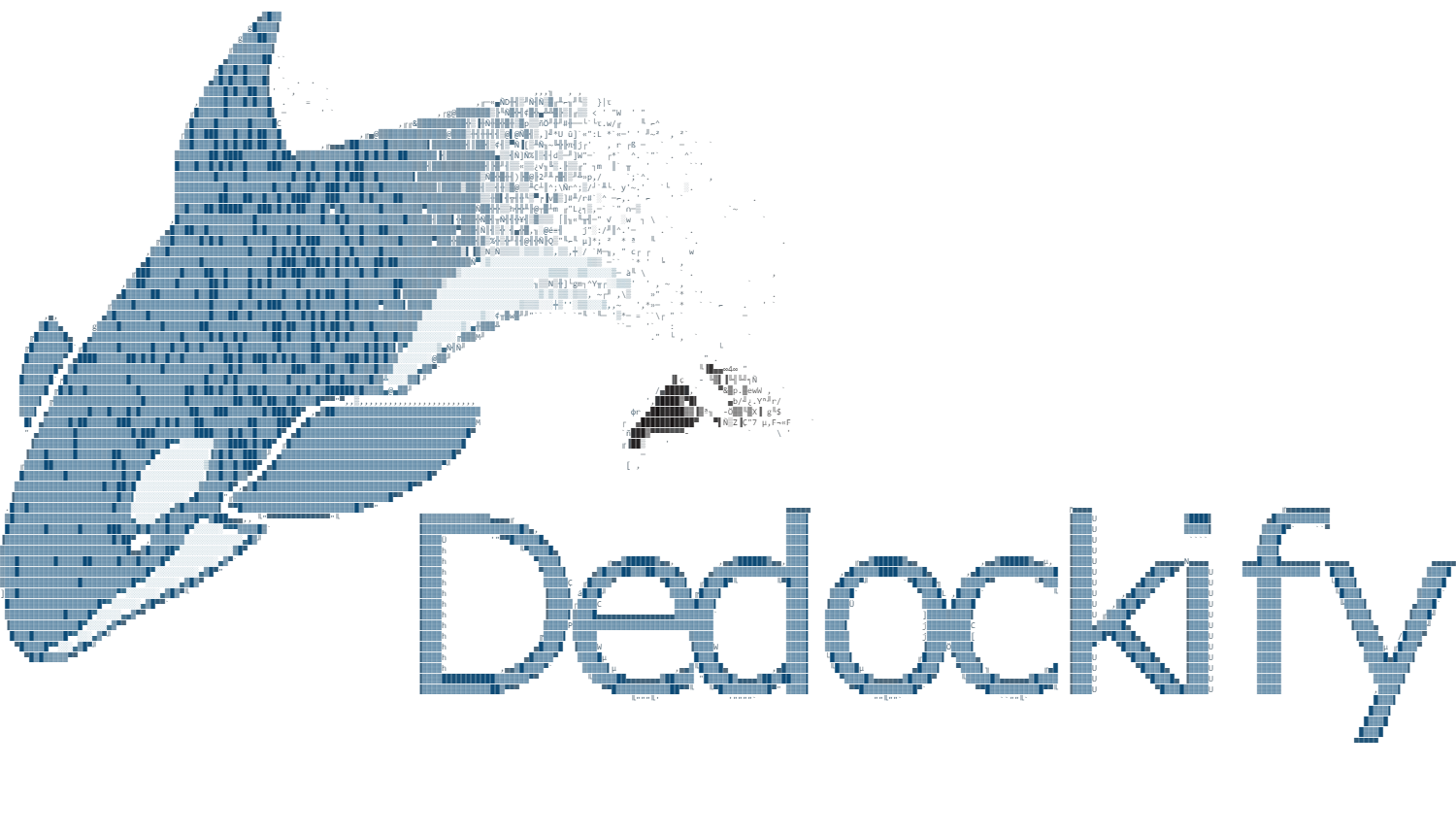
Dedockify
Let’s take this a few steps further. In order to help reverse engineer this image into a Dockerfile, we will need to parse everything and reformat it into a form that is readable. Please note that for the purposes of this article, the following Python 3 code has been made available and can be obtained from the Dedockify repository on GitHub. Thanks goes to LanikSJ for all prior work.
from sys import argv import docker class ImageNotFound(Exception): pass class MainObj: def __init__(self): super(MainObj, self).__init__() self.commands = [] self.cli = docker.APIClient(base_url='unix://var/run/docker.sock') self._get_image(argv[-1]) self.hist = self.cli.history(self.img['RepoTags'][0]) self._parse_history() self.commands.reverse() self._print_commands() def _print_commands(self): for i in self.commands: print(i) def _get_image(self, img_hash): images = self.cli.images() for i in images: if img_hash in i['Id']: self.img = i return raise ImageNotFound("Image {} not found\n".format(img_hash)) def _insert_step(self, step): if "#(nop)" in step: to_add = step.split("#(nop) ")[1] else: to_add = ("RUN {}".format(step)) to_add = to_add.replace("&&", "\\\n &&") self.commands.append(to_add.strip(' ')) def _parse_history(self, rec=False): first_tag = False actual_tag = False for i in self.hist: if i['Tags']: actual_tag = i['Tags'][0] if first_tag and not rec: break first_tag = True self._insert_step(i['CreatedBy']) if not rec: self.commands.append("FROM {}".format(actual_tag)) __main__ = MainObj()
Initial Dockerfile Generation
If you’ve made it this far, then you should have two images: wagoodman/dive
and our custom example1
image.
$ docker images REPOSITORY TAG IMAGE ID CREATED SIZE example1 latest 374e0127c1bc 42 minutes ago 0B wagoodman/dive latest 4d9ce0be7689 2 weeks ago 83.6MB
Running this code against our example1
image will finally produce the following:
$ python3 dedockify.py 374e0127c1bc FROM example1:latest COPY file:e3c862873fa89cbf2870e2afb7f411d5367d37a4aea01f2620f7314d3370edcc in / COPY file:2a949ad55eee33f6191c82c4554fe83e069d84e9d9d8802f5584c34e79e5622c in / COPY file:aa717ff85b39d3ed034eed42bc1186230cfca081010d9dde956468decdf8bf20 in /
We’ve extracted nearly the same information that we observed when we explored the image with dive
earlier. Notice the FROM
directive shows us example1:latest
instead of scratch
. Our code is making an assumption about the base image that is technically incorrect in this case.
As a comparison, let us do the same thing with our wagoodman/dive
image.
$ python3 dedockify.py 4d9ce0be7689 FROM wagoodman/dive:latest ADD file:fe1f09249227e2da2089afb4d07e16cbf832eeb804120074acd2b8192876cd28 in / CMD ["/bin/sh"] ARG DOCKER_CLI_VERSION= RUN |1 DOCKER_CLI_VERSION=19.03.1 /bin/sh -c wget -O- https://download.docker.com/linux/static/stable/x86_64/docker-${DOCKER_CLI_VERSION}.tgz | tar -xzf - docker/docker --strip-component=1 \ && mv docker /usr/local/bin COPY file:8385774b036879eb290175cc42a388877142f8abf1342382c4d0496b6a659034 in /usr/local/bin/ ENTRYPOINT ["/usr/local/bin/dive"]
This shows a lot more diversity compared to our example1
image. We notice the ADD
directive just before the FROM
directive. Our code is making the wrong assumption again. We don’t know what the ADD
directive is adding. We can intuitively make the assumption, however, that we don’t know for sure what the base image is. The ADD
directive could have been used to extract a local tar
file into the root directory. It’s possible that it was using this method to load another base image.
Dedockify Limitation Testing
Let’s experiment by creating an example Dockerfile
where we explicitly define the base image. As we did earlier, in an empty directory, run the following snippet directly from the command line.
cat > Dockerfile << EOF ; touch testfile1 testfile2 testfile3 FROM ubuntu:latest RUN mkdir testdir1 COPY testfile1 /testdir1 RUN mkdir testdir2 COPY testfile2 /testdir2 RUN mkdir testdir3 COPY testfile3 /testdir3 EOF
Now, perform a build that tags our new image as example2
. This will create a similar image as before, except instead of using scratch
it will use ubuntu:latest
as the base image.
$ docker build . -t example2 Sending build context to Docker daemon 3.584kB Step 1/7 : FROM ubuntu:latest ---> 72300a873c2c Step 2/7 : RUN mkdir testdir1 ---> Using cache ---> 4110037ae26d Step 3/7 : COPY testfile1 /testdir1 ---> Using cache ---> e4adf6dc5677 Step 4/7 : RUN mkdir testdir2 ---> Using cache ---> 22d301b39a57 Step 5/7 : COPY testfile2 /testdir2 ---> Using cache ---> f60e5f378e13 Step 6/7 : RUN mkdir testdir3 ---> Using cache ---> cec486378382 Step 7/7 : COPY testfile3 /testdir3 ---> Using cache ---> 05651f084d67 Successfully built 05651f084d67 Successfully tagged example2:latest
Since we now have a slightly more complex Dockerfile
to reconstruct, and we have the exact Dockerfile
we used to generate this image, we can make a comparison. Let us generate the output from our Python script.
$ docker images REPOSITORY TAG IMAGE ID CREATED SIZE example2 latest 05651f084d67 2 minutes ago 64.2MB example1 latest 374e0127c1bc 1 hour ago 0B ubuntu latest 72300a873c2c 9 days ago 64.2MB wagoodman/dive latest 4d9ce0be7689 3 weeks ago 83.6MB
$ python3 dedockify.py 05651f084d67 FROM ubuntu:latest RUN /bin/sh -c mkdir testdir1 COPY file:cc4f6e89a1bc3e3c361a1c6de5acc64d3bac297f0b99aa75af737981a19bc9d6 in /testdir1 RUN /bin/sh -c mkdir testdir2 COPY file:a04cdcdf5fd077a994fe5427a04f6b9a52288af02dad44bb1f8025ecf209b339 in /testdir2 RUN /bin/sh -c mkdir testdir3 COPY file:2ed8ccde7cd97bc95ca15f0ec24ec447484a8761fa901df6032742e8f1a2a191 in /testdir3
This correlates well with the original Dockerfile
. There’s no ADD
directive this time, and the FROM
directive is correct. Provided that our base image is defined in the original Dockerfile
, and it avoids using scratch
or avoids using the ADD
directive to create a base image from a tar
file, we should be able to reconstruct the Dockerfile
with some accuracy. We still don’t know the names of the original files that were copied, however.
Blind Freestyle Dockerfile Reconstruction
Now, let us try reverse engineering a Docker container the proper way using the tools that we’ve already discussed. The container we will use has been modified from the above examples. Our earlier Dockerfile has been modified to create example3
. The image has been made functional by adding a small binary. The assembly source code is available here in the Dedockify GitHub repository. Since this image is so small, we won’t need to build or pull it. We can just copy and paste the entire container right into our Docker environment with the snippet below.
uudecode << EOF | zcat | docker load begin-base64 600 - H4sICMicXV4AA2V4YW1wbGUzLnRhcgDtXVtvG8cVVnp56UN/QJ/YDQokgETN zJkrgTykjgsbDSzDURMkshDM5YzFhiJVkkpiCELzH/pP+tYfkf/UsxRNXdxI spe7lqv5IJF7PTM7Z87MmY9nZxgL2qG1DkN2nkXmtTecQwYrMMfsBHgXgFuV eXLCI5c26sxdNHQsie2Nm8GYZEYp+l7g6vdim4MWBrgyBjaY4MbIjZ66hezG OJ7N/ZSyMp1M5tddd9P5qw/3noA11f+XD5998XjnybVpcMa0lNfoH67oHxiI jV4nhXjP9c/7701WC1pAY/v/+2wyvimNG+zfgLpi/0KbYv+d4KQapmpQNa0G 1WZ15Kc4npMsr7JUjGGMyLUzPEXJc1RCWqFkTtoEL5TgEaLSKlmOiXHnUSlK jYsQSFacop9jnTHuDNtinP52GRss/r6pL5iM5344xum3tJWHL6rBSfVoMpuP /SHSXXTFZ5NDuuB8/28znJ5tfTqf+3jwxTwNx9Ug+9EMLxybHM9fP4jT6erg 7vzlanvnCMeX5Sz2dsYRV0cejr+vBuPj0WizenCYXm0+PvQvlhn7cjI6PsTZ qzNfTabfDccvPhsuc/twPJ++PJoM66I9u2Jn/Ofj4Wgl6nMfcLS8/XSzmtBm NRqOj3+sTm+h/8b2P/IvcdqvbeiX07je/uXr/h9oZor9dwF/dHQbF74R3sz/ F1RfuKbLi//fAWr993846C/+J0f/aCONRR9/g/4vbXNQShb7LygoKGgTTDkP 1tiEUvkgJajgnTZRA0pAplFqZ1m02hqXjc4+aaVTztx4pzywfvPxH7X1V/0/ oZgu7X8XOKn8NB4M5xjnx9N6ROIPk5ZnI6y7P67aq55+uvvok+3j2XR7NIl+ tD0Lw/Hgwv5q9/zEYuNslz6q/f85MJsd0CBVD4SHEDhGkM4LDIqHQN4JjU5s 5NqiJguRAr3nKpgICoRhyLNiCgHQOLxhfLdN7teVMd7e4uD2AY5Gkzpv14/2 VuPgegyvDA3aOATr5cJkJUs0tMsRacytE6MsGZnp/piCEMaiijHmJJOIihvN bh5WX0zh/7kqkA5od3t2QI+yFenjw4/Gk6OPe7Wqnuw++/rpzuMnu7295xdU 9bzar29/X6rPyenpRZZFMMG2GGwxsStgoPhAQF8KrZ1grqZb0iR+R5Xie5zO hpPxgpbpM+hrOnUwnM0nU1LY3sm1AnnfOXCgDDPfnDM834aX9XOclXZvK/aW Jf3VzrO/fvb4WW97jjPS95RXp5vXyxd9Qd0MSCnsLeQ/2Hn6dS8PRzgAIbLL TiBEJ9Ej8hRZEmCURW6CcTaD8+h18BhtdsrJIFDGmBigS6w3HPfqTNbCeO8W 2SQjVBK4lW9RDOIW8hUZv+PG8XdWDOI2xWA4mYGkKvYWxQC3kO+YdMYxod5Z McDNxQB9Zpkg38iJNymG2uxvFi005+RJwRsVQAAIkmURmaF+gwUXvAZjApis tWaSJyWFoAaKOS1DFJrKR0omQSSNVvO6ABaNz20e/mITc0MOe9c1vJsVHh7N X3674CKrwXx6jKf7l6jQzar233Ld8lXzl0d1E724eFY3bsOcvx2mWd14Lttt riUXQXJAZFJn5MLriMHJTA6yT44zHZjHQJ2qZM5watCpBeeevFdMdANJXUkC 7ZMxjCq7TI6cbW+zUkEpqj/RMrBeWInCUuFqj3QZTxays9RrBNT+XBJDh2Qw wQkfqXcAqjZK5RA5SNIJzywKSTKTMGiYjkoknwJjzgVg1vBwLilYbbwhFzsz LYTTwqNgRiMEZhMYK7zztZ9gmHUZap8/0WOROCXRenUhT1Q8IlhltBbOihhR ZhOtoucgwVRolD3DkqprS6bHZzlbmxMNOBJGRYVxLsmDcSGZJBkTjkdlEtW3 IKj4nA0oUJHDYgP5MZQ3qjHKZCrDqJSylvwZhAtPF5PRMfJEt4I0kjvms/Qq U4VVnp6O6jD3PJpaujKcLqJqaNF5TlrR5lxS5jQ2IfVRv22MAhbInjAaiNIp zkSGCAacVmAj0IPWDVoKPFCjQMXi0VX7p7eh4N8phI70kElxeg7vhJIKZYpa qOy9J9Vpck3Qkx2I2qOMIUrSQ46GKrzkqHRb8R+cF/63CzTWfyvxH8LI8vtP JyjxH/cbje1/DfEfWvDX4j8YL/bfBZbxH02rQYnZ6DBmY51obP/txH8oUX7/ 7QSv+LU2g0DezP/nVF+EBij+fxdY6b/FIJC6PN4s/kOqWv/F/gsKCgrag2Jg EIxFl3RyIWQB2bjkDIiYFeTIUuLJy6SNNUG4wKPl3GeXEgSfWuL/BNTxv6X/ bx+N9d/O+18gVen/u0Dh/+43Gtt/K+9/gWDF/jvBkv9rWg0uvf/lBSZQEqIi YUoB50E7iU4JFD4ESbcok5Kq6SWbIWjFpbFSCOmUYaxwiR1yiY3tvxX+T3JR 3v/sBK8Cy+4O/yfO+L/y/lcnWOn/rvF/hf8vKCgoaBXGQgwYvEKWwNmY0euo As/cks/mFGMgBI+BfD7jaJtrHaMnhx1ZMAF9a/M/6dL/d4HG+m9r/qfS/3eC wv/dbzS2/7bmfyr23wmW/F/TanCR/7PKBZAyeI7SuGDqd0Y9ahVYZj6nJFFq pZS2UieBDAC05HRxNBKE06nwfx3yf43tv6X5n3iJ/+0Er96ovHP8X/H/O8FK /3eN/yu//xUUFBS0iqbOekv8nzSs9P9doLH+23n/V4ky/1cnKPzf/UZj+2+H /wNd7L8TLPm/NXB2K/5vDbGEhf/riv9rbP/t8H/Ayvt/naDE/xX9L/S/mrxv /Wnc7P9f1P+C/5OyxP8WFBQUtIqmizW1Ff9X+v9u0Fj/Lc3/Z4r/3wkK/3e/ 0dj+W4r/K/P/dIMl/7eGNRtX/N8aYgkL/9cV/9fY/lua/0+xYv9doMT/Ff0v 9L9atWL9abwV/1fi/wsKCgpaRdPJetqK/6vX/y39f/torP+W4v94+f2vExT+ 736jsf23FP+niv13glf8X/M5+1b8X1lL5H3i/5rafzv8n9Bl/a9OcPfW/1jy f8X/7wQr/a/WMF5/Gm/F/xX+v6CgoKBVJPK8tUkQIUjIXCXMBoJj0oPO3Btu jJc86SyRWaFFDOhQ8QxKJ8uya2n9D6bK+h+doLH+25n/j/My/0cnKPzf/UZj +2/O/wmQr63/a0r8TzdY8n9Nq8FF/o+xoB1a6zBk51lkXpMADhmswByzE+Bd AG5V5skJj1zaSKm4aOhYEpf4P8EE22KwxcSugIHiAwF9KbR2grlLRGCdsKrf XuUQrJe25hYlS5SRHDFpoxNDACOzDxhToCpmUcUYc5JJRMWNZjfzihdTuGsc 41719NPdR59sH8+m26NJ9KPtWRiOBxf2V7vnJxYbZ7v0Ue0vScq9qt7dnh3Q o2xF+vjwo/Hk6ONezUc+2X329dOdx092e3vPq21/dLR9gKPR5Hm1X9/+itic HXih9EB4CIFjBOm8wKB4CIxMnRkbubaokRoGgd5zFUwEBcIw5FkxRbpC4/AG frRO/gpHunchT3WGfoEtPTk93azSJH5Hqv4ep7PhZLxgm/sM+vqsItx9Wnmd Kr/MS9919S3Jbj+NB8M5xvnxtM61P0y6tst3uJ72+4bG/X9z/l+9Pv4DXd7/ 6Qa1sbWdxhuP/znXqsT/doJVY9tiGrX9X6t/Y67w/9SPlPF/J/jnw8//8sFq 78Fvf7Xx640/0Hf9/xs68h9OG//+/c8//am38cHGv37+6VFdWTZ7P0ymo/TH 3727jBcUFBQUNMKhHw8zzub9W/B4b4sb/H8O0lzyBemIEuX9n06wd1I9WFId FVPOgzU2oVQ+SAkqeKdN1IASaKiPUrsFr2aNy0Znn8hNTzlz453ywBZ1iIbf z/BosutfzOrRPP7oD49GCIORryNM6iH95/WIcXGyaaTg+eCTUl1fJBsJW9+y uCRsfXPskbD1LdhRl9na3v4lYU1530vC1sdLVPun+8VP/SVMyVZnw/lkOsRZ W2nc9PsvB7ja/pNBlPa/C5ysWuia61420mv4Pej0tNhcQUFBwV3GfwHMszUX AMIAAA== ==== EOF
Running everything directly from the command line will load example3:latest
. Now, let us try to recreate the Dockerfile
.
$ docker images REPOSITORY TAG IMAGE ID CREATED SIZE example3 latest 059a3878de45 5 minutes ago 63B
$ python3 dedockify.py 059a3878de45 FROM example3:latest WORKDIR /testdir1 COPY file:322f9f92e3c94eaee1dc0d23758e17b798f39aea6baec8f9594b2e4ccd03e9d0 in testfile1 WORKDIR /testdir2 COPY file:322f9f92e3c94eaee1dc0d23758e17b798f39aea6baec8f9594b2e4ccd03e9d0 in testfile2 WORKDIR /testdir3 COPY file:322f9f92e3c94eaee1dc0d23758e17b798f39aea6baec8f9594b2e4ccd03e9d0 in testfile3 WORKDIR /app COPY file:b33b40f2c07ced0b9ba6377b37f666041d542205e0964bc26dc0440432d6e861 in hello ENTRYPOINT ["/app/hello"]
This gives us a base Dockerfile
to work from. Since example3:latest
is the name of this image, we can assume from the context that it’s using scratch
. Now, we need to see what files were copied into /testdir1
, /testdir2
, /testdir3
, and /app
. Let us run this image against dive
to see how we will recover the missing data.
docker run --rm -it \ -v /var/run/docker.sock:/var/run/docker.sock \ wagoodman/dive:latest example3:latest
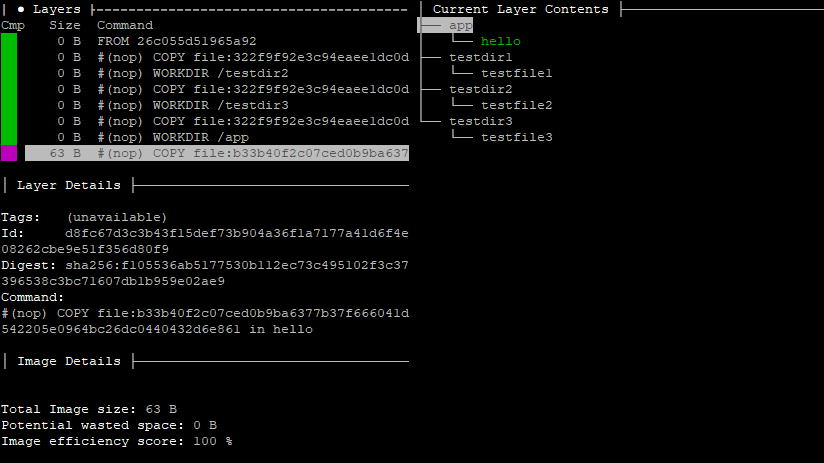
If you scroll down to the last layer, you’ll be able to see all of the missing data populate the tree on the right. Each of the directories had zero-byte files named testfile1
, testfile2
, and testfile3
copied to it. And in the last later, a 63-byte file was copied called hello
to the /app
directory.
Now, let us recover those files! There doesn’t appear to be a way to copy the files directly from the image, so we will need to create a container first.
$ docker run -td example3:latest 6fdca182a128df7a76e618931c85a67e14a73adc69ad23782bc9a5dc29420a27
Now, let us copy the files we need from the container to the host using the path and filenames we recovered from Dive
below.
/testdir1/testfile1 /testdir2/testfile2 /testdir3/testfile3 /app/hello
We might first check to see if our container is still running.
$ docker ps CONTAINER ID IMAGE COMMAND CREATED STATUS PORTS NAMES 6fdca182a128 example3:latest "/app/hello" 2 minutes ago Up 2 minutes wizardly_lamport
If a container isn’t running for some reason, that’s fine. We can verify its status to see that it’s stopped.
$ docker container ls -a
We can also check the logs.
$ docker logs 6fdca182a128 Hello, world!
It appears to be running a persistent Hello, world!
program. Actually, in this case, the Hello, world!
program wasn’t designed to be persistent. In Docker version 19.03.6
, there may be a bug that’s preventing the application from terminating normally. This is acceptable for now. The container can be active or stopped; the application doesn’t need to be persistent to recover any of the data we need. A container in any state only needs to be generated from the source image for which we are extracting data.
docker cp 6fdca182a128:/testdir1/testfile1 . docker cp 6fdca182a128:/testdir2/testfile2 . docker cp 6fdca182a128:/testdir3/testfile3 . docker cp 6fdca182a128:/app/hello .
By running the recovered executable to verify its behavior, we should see the following:
$ ./hello Hello, world!
With the Dockerfile
we generated earlier, we can update it to include all the new details. This includes updating the FROM
directive to scratch
, along with all of the discovered filenames we found while exploring with Dive
.
FROM scratch WORKDIR /testdir1 COPY testfile1 . WORKDIR /testdir2 COPY testfile2 . WORKDIR /testdir3 COPY testfile3 . WORKDIR /app COPY hello . ENTRYPOINT ["/app/hello"]
Again, combining all files in a shared folder, we’re ready to run our reverse engineered Dockerfile
.
$ docker build . -t example3:recovered Sending build context to Docker daemon 4.608kB Step 1/10 : FROM scratch ---> Step 2/10 : WORKDIR /testdir1 ---> Running in 5e8e47505ca6 Removing intermediate container 5e8e47505ca6 ---> d30a2f002626 Step 3/10 : COPY testfile1 . ---> 4ac46077a588 Step 4/10 : WORKDIR /testdir2 ---> Running in 8c48189da985 Removing intermediate container 8c48189da985 ---> 7c7d90bc2219 Step 5/10 : COPY testfile2 . ---> 5b40d33100e1 Step 6/10 : WORKDIR /testdir3 ---> Running in 4ccd634a04db Removing intermediate container 4ccd634a04db ---> f89fdda8f059 Step 7/10 : COPY testfile3 . ---> 9542f614200d Step 8/10 : WORKDIR /app ---> Running in 7614b0fdba42 Removing intermediate container 7614b0fdba42 ---> 6d686935a791 Step 9/10 : COPY hello . ---> cd4baca758dd Step 10/10 : ENTRYPOINT ["/app/hello"] ---> Running in 28a1ca58b27f Removing intermediate container 28a1ca58b27f ---> 35dfd9240a2e Successfully built 35dfd9240a2e Successfully tagged example3:recovered
$ docker run --name recovered -dt example3:recovered 0f696bf500267a996339b522cf584e010434103fe82497df2c1fa58a9c548f20 $ docker logs recovered Hello, world!
Now, for further verification, lets check the layers with dive
again.
docker run --rm -it \ -v /var/run/docker.sock:/var/run/docker.sock \ wagoodman/dive:latest example3:recovered
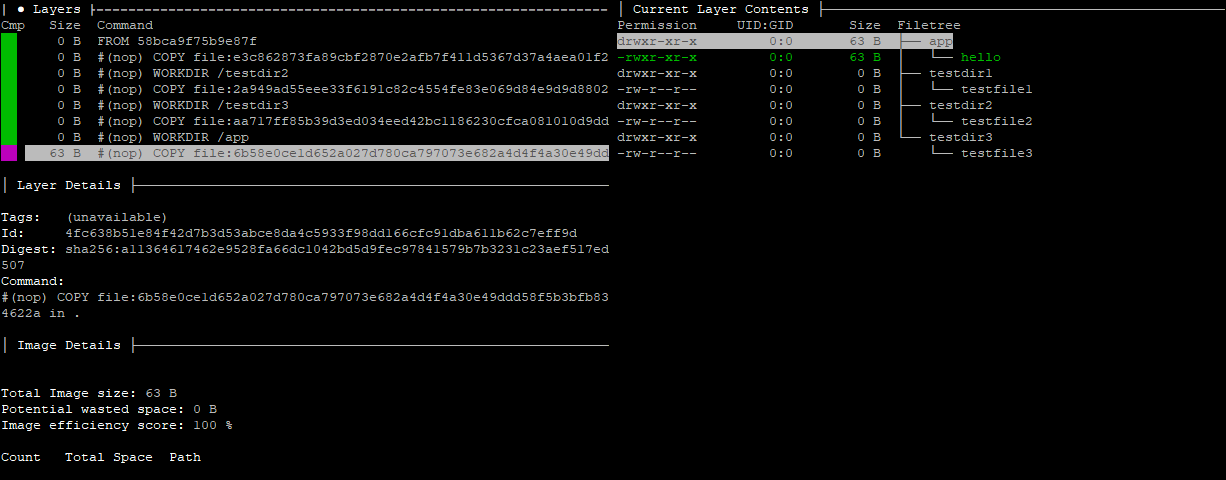
This image shows the same files as the original. Comparing the two images side, by side, they both show that they match. Both show the same file sizes. And both function in exactly the same way.
Here is the original Dockerfile
used to generate the original example3
image.
FROM alpine:3.9.2 RUN apk add --no-cache nasm WORKDIR /app COPY hello.s /app/hello.s RUN touch testfile && nasm -f bin -o hello hello.s && chmod +x hello FROM scratch WORKDIR /testdir1 COPY --from=0 /app/testfile testfile1 WORKDIR /testdir2 COPY --from=0 /app/testfile testfile2 WORKDIR /testdir3 COPY --from=0 /app/testfile testfile3 WORKDIR /app COPY --from=0 /app/hello hello ENTRYPOINT ["/app/hello"]
We can see that, while we weren’t able to reconstruct it perfectly, we were able to reconstruct approximately. There’s no way to reconstruct a Dockerfile that uses a multi-stage build like this one. The information simply isn’t available. Our only option is to reconstruct the Dockerfile of the image we actually have. If we have images from the eariler build stages, we can reproduce a Dockerfile for each of those, but in this case, all we had was the final build. But regardless, we have still successfully reproduced a useful Dockerfile
from a Docker image.
Future Work
By using a similar approach as dive
, we should be able to update the Dedockify
source code to transgress through each of the layers automatically in order to recover all useful file information. Also, the program can be updated to be able to automatically recover files from the container and store them locally, while also automatically making appropriate updates to the Dockerfile
. Finally, the program can also be updated to be able to easily infer if the base layer is using an empty scratch
image, or something else. With some additional changes to the recovered Dockerfile
syntax, Dedockify
can potentially be updated to completely automate the reverse engineering of a Docker image into a functional Dockerfile
in most cases.