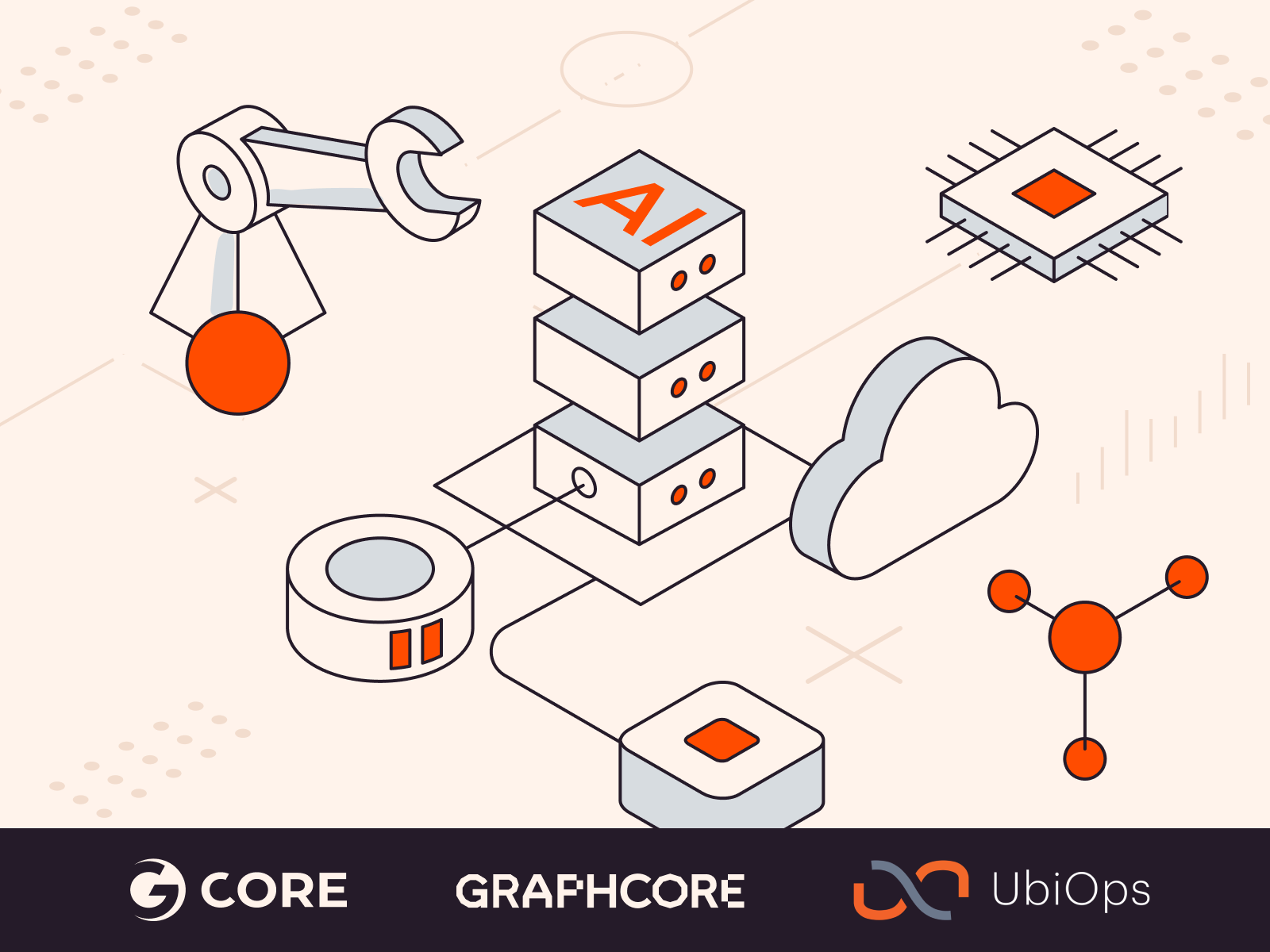
As AI and machine learning advance rapidly, the demand for high-performance computing hardware grows. We are excited to announce a partnership between Gcore, Graphcore, and UbiOps that offers a new, powerful solution in this sphere. Graphcore’s Intelligence Processing Units (IPUs,) UbiOps’ powerful MLOps platform, and Gcore Cloud together offer unmatched efficiency for AI and ML workloads. Let’s give it a closer look.
Unique Service Offering for AI Teams: On-Demand IPUs in the Cloud
By partnering with Graphcore and UbiOps, Gcore Cloud is taking a significant step forward in empowering AI teams. Our unique service offering combines the best IPU hardware, MLOps platform, and cloud infrastructure.
Graphcore is a leading company in developing IPU hardware designed to meet the demanding requirements of modern AI tasks. IPUs primarily leverage model parallelization to speed up computational tasks, compared to data parallelization offered by GPUs.
UbiOps is a powerful machine learning operations (MLOps) platform that simplifies AI model deployment, orchestration, and management. It helps businesses efficiently run AI models and workflows in various cloud computing environments, accelerating their time to market with AI solutions, saving on DevOps and cloud engineering costs, and efficiently using compute resources with on-demand hardware scaling.
Together, Gcore Cloud, Graphcore, and UbiOps are creating a seamless experience for AI teams, enabling them to easily run their workloads on IPUs by making them available in the UbiOps platform. Leveraging UbiOps’ multi-cloud technology, the orchestration layer was connected to the Gcore Cloud infrastructure with Graphcore IPUs, making the IPUs readily available on-demand for UbiOps users to run AI models and training jobs.
This integration allows users to leverage the computational power of IPUs for their specific job requirements, enabling IPU-powered scalable model inference APIs and faster model training jobs in the UbiOps platform. Users can also take advantage of the out-of-the-box MLOps features that UbiOps offers, such as model versioning, governance, and monitoring.
Benchmarking the Benefits of IPUs
To demonstrate the benefits of using IPUs compared to other devices, we benchmarked workloads on three different compute resources: CPU, GPU, and IPU.
Device type | Device | CPU RAM | vCPU |
CPU | CPU | 70GB | 10 |
GPU | A100 (40 GB) | 70GB | 10 |
IPU | POD-4 | 70GB | 10 |
A convolutional neural network (CNN) was trained on the CIFAR-10 dataset on these three different devices, and the training speeds for different effective batch sizes were compared accordingly. (We took the product between the data batch size and the gradient accumulation.)
Type | Effective batch size* | Graph compilation (s) | Training duration (s) | Time per epoch (s) | Unit cost (€/h) |
IPU-POD4 | 50 | ~180 | 472 | 08.1 | From €2.5 |
IPU-POD4 | 8 | ~180 | 1420 | 26.0 | From €2.5 |
GPU | 50 | 0 | 443 | 08.6 | From €4 |
GPU | 8 | 0 | 2616 | 51.7 | From €4 |
CPU | 50 | 0 | ~5 hours | 330 | From €1.3 |
CPU | 4 | 0 | 10+ hours | 10+ minutes | From €1.3 |
The results showed that the training times were already quite lengthy on a CPU, even for a relatively simple CNN and small dataset. Using specialized hardware, a significant improvement in speed was visible on IPU and GPU. With minimal optimization, an even shorter time per epoch could be achieved on IPU versus GPU.
Although the initial cost of an IPU is higher than a CPU, its efficiency more than justifies it. The time savings generated by an IPU can lead to faster results and innovation, contributing to a higher return on investment.
Accelerating AI Innovation
This collaboration between Gcore Cloud, Graphcore, and UbiOps unlocks the potential of IPUs for AI and ML workloads, providing AI teams with accessible, high-performance computing resources. We’re excited about the potential of this partnership to foster success and help more AI projects achieve their goals.
If you want to try out Graphcore IPUs on Gcore Cloud with UbiOps, contact sales@gcore.com.