Managed Kubernetes with GPU Worker Nodes for Faster AI/ML Inference
- By Gcore
- November 23, 2023
- 6 min read
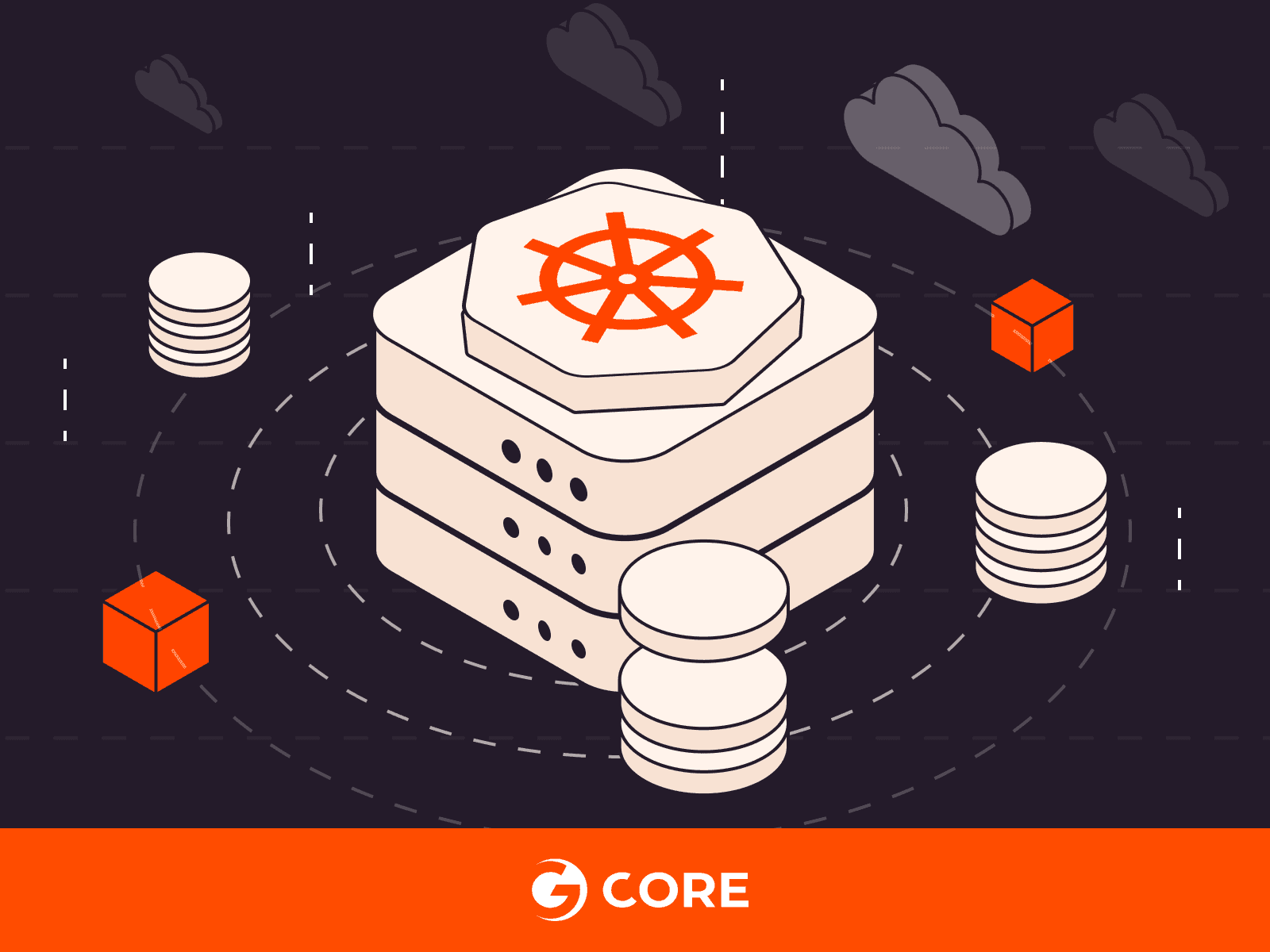
Currently, 48% of organizations use Kubernetes for AI/ML workloads, and the demand for such workloads also drives usage patterns on Kubernetes. Let’s look at the key technical reasons behind this trend, how AI/ML workloads benefit from running on GPU worker nodes in managed K8s clusters, and some considerations regarding GPU vendors and scheduling.
Why Kubernetes is Good for AI/ML
A number of features make Kubernetes popular and effective in the AI/ML realm:
- Scalability. K8s enables seamless, on-demand scalability of AI/ML workloads. This is especially critical for inference workloads because they are more dynamic regarding resource utilization than training workloads, and can be resource-intensive. The latter means they often require frequent scaling up or down based on the volume of data being processed.
- Automated scheduling. The ability to automatically schedule AI/ML workloads reduces the operational overhead for MLOps teams. It also improves the performance of AI/ML applications by ensuring they are scheduled to the nodes that have the required resources.
- Resource utilization. K8s can help to optimize physical resource utilization for AI/ML workloads. It can dynamically and automatically allocate the required amounts of CPU, GPU, and RAM resources. This is critical due to the resource-intensive nature of these workloads and the potential for cost reduction.
- Flexibility. With K8s, you can deploy AI/ML workloads across multiple infrastructures, including on-premises, public cloud, and edge cloud. This feature also makes Kubernetes a good option for organizations that need to deploy AI/ML workloads in hybrid or multicloud environments.
- Portability. You can easily migrate Kubernetes-based AI/ML applications between different environments and installations. This is critical for deploying and managing AI/ML workloads in a hybrid infrastructure.
Use Cases
Here are some examples of how companies have adopted Kubernetes (K8s) for their AI/ML projects:
- OpenAI has been an early adopter of K8s. In 2017, the company was running machine learning experiments on K8s clusters. With the K8s autoscaler, OpenAI could deploy such a project in a few days and scale it up to hundreds of GPUs in a week or two. Without the Kubernetes autoscaler, such a process would take months. As a result, OpenAI increased the number of AI experiments tenfold. In 2021, the company expanded its K8s infrastructure to 7,500 nodes for large ML models such as GPT-3, DALL-E and CLIP.
- Shell uses a K8s-based platform Kubeflow to run tests and quickly experiment with ML models on laptops. Engineers can move these workloads from the test environment to production, and the workloads will function just the same. With Kubernetes, Shell builds thousands of ML models in two hours instead of a month. The time to write the underlying code is reduced from two weeks to four hours.
- IKEA has developed an internal MLOps platform based on K8s to train ML models on-premises and get inference in the cloud. This allows the MLOps team to orchestrate different types of trained models and, ultimately, improve the customer experience.
Of course, these examples are not broadly representative. Most companies are not fully AI-focused like OpenAI and are not as large as IKEA. They can’t afford to train large AI/ML models from scratch, which takes time and money, but instead run pretrained models and integrate them with other internal services. In other words, these companies use AI/ML inference, not training.
Inference workloads tend to be more dynamic regarding resource utilization than training workloads because production clusters are more likely to experience user and traffic spikes. In such cases, the infrastructure needs to scale up and down quickly, whereas AI/ML training typically requires gradual scaling. Therefore, for AI/ML models that are already trained and deployed, the scalability and dynamic resource utilization of K8s are especially beneficial.
Why GPU Is Better than CPU for Worker Nodes
GPU worker nodes are a better fit for containerized AI/ML workloads than CPU worker nodes for the same reasons as for non-containerized workloads: GPU offers parallel processing capabilities and higher performance for AI/ML than CPUs.
Inference for AI/ML workloads running on GPU worker nodes can be faster than those running on CPU worker nodes due to the following factors:
- The GPU’s memory architecture is specifically optimized for AI/ML processing, enabling higher memory bandwidth than CPUs.
- GPUs often provide better computational performance than CPUs for AI/ML training and inference because they have more transistors to process data.
Kubernetes adds its own performance benefits to those of GPUs. In addition to hardware acceleration, AI/ML workloads running on GPU worker nodes get scalability and dynamic resource allocation. Kubernetes also includes plugins for GPU vendor support, making it easy to configure GPU resources for use by AI/ML workloads.
With Kubernetes, you can manage GPU resources across multiple worker nodes. Containers consume GPU resources in essentially the same way as they consume CPU resources.
GPU Vendors Comparison
There are three GPU vendors available for Kubernetes: NVIDIA, AMD, and Intel. When choosing GPU vendors for worker nodes, it’s important to keep in mind that their compatibility with Kubernetes, tool ecosystem, performance, and cost can vary.
NVIDIA GPU worker nodes | AMD GPU worker nodes | Intel GPU worker nodes | |
Compatibility with K8s | Excellent | Good | Good |
Tools ecosystem | Excellent | Good | Fair |
Performance | Excellent | Good | Fair |
Cost | High | Medium | Medium |
Let’s compare the three vendors.
- Compatibility with Kubernetes: NVIDIA is the most compatible with K8s. The company provides CUDA drivers, various container runtimes, and other tools and features that simplify GPU integration and management. AMD and Intel support for K8s is less mature and often requires custom configuration.
- Tools ecosystem: NVIDIA has the best ecosystem of tools for AI/ML, thanks to software such as the GPU Operator and Container Toolkit, and ML frameworks adapted for NVIDIA GPUs, such as TensorFlow, PyTorch, and MXNet. AMD and Intel also have tools for AI/ML, but they are not as comprehensive as NVIDIA’s.
- Performance: NVIDIA GPUs are known for their high performance on AI workloads, outperforming the competition on most MLPerf benchmarks. NVIDIA GPUs are ideal for demanding tasks such as deep learning and high-performance computing.
- Cost: NVIDIA GPUs are the most expensive type of GPU worker node.
- Flexibility: NVIDIA offers several features that make its GPU-based K8s clusters highly flexible in terms of management and resource utilization compared to its competitors:
- Multi-instance GPU (MIG) mechanism for NVIDIA A100 GPU to allow a GPU to be securely partitioned into up to seven separate instances for better GPU utilization
- Multicloud GPU clusters, which can be seamlessly managed and scaled as if deployed in a single cloud
- Heterogeneous GPU and CPU clusters to simplify the training and management of distributed deep learning models
- GPU metrics monitoring with Prometheus and visualization with Grafana
- Support for multiple container runtimes, including Docker, CRI-O, and containers
In summary, NVIDIA GPU worker nodes are the best choice for AI/ML workloads in Kubernetes. They offer the best compatibility with K8s, the best tools ecosystem, and the best performance. That’s why we chose NVIDIA GPUs for Gcore Managed Kubernetes. Our customers get all the benefits of NVIDIA, including the highest performance level for faster training and inference of their AI/ML workloads.
Important Specifics of GPU Scheduling in Kubernetes
To enable GPU scheduling and allow pods to access its resources, you need to install a vendor-specific device plugin from your chosen GPU vendor — NVIDIA, AMD, or Intel.
Pods request GPU resources in the same way they request CPU resources. However, Kubernetes is less flexible with GPU than with CPU when it comes to configuring `limits`
and `requests`
. With `requests`
, you set the amount of resources that a pod is guaranteed to get, such as a minimum quantity. With `limits`
, you set the amount of resources that won’t be exceeded, for instance, a maximum quantity. When configuring a pod manifest for GPU requests, `limits`
and `requests`
should be equal, meaning that a pod won’t get more resources than guaranteed if, for example, the application needs them.
Also, by default, you can’t allocate part of a GPU or multiple GPUs to a container because of the way CPU allocation works. You can only allocate one full GPU per container. This limitation doesn’t help with resource economics. But NVIDIA has managed to overcome this. With its GPU, you can use either use:
- Time-sharing GPUs, which work by sequentially assigning time intervals to shared containers on a physical GPU. This works for all NVIDIA GPUs.
- Multi-instance GPUs, which allow a GPU to be divided into up to seven instances for better GPU utilization. This only works with the NVIDIA A100 GPU.
These two features help you to use NVIDIA GPU resources more efficiently and save money on renting GPU instances in the cloud. This is also a significant advantage over other GPU vendors.
Managed Kubernetes vs. Vanilla Kubernetes with GPU
A managed Kubernetes service can offer several advantages over vanilla (open source) Kubernetes for AI/ML workloads running on GPU worker nodes:
- Flexible choice of GPUs. Managed K8s services typically provide support for GPU instances with various specifications. This makes it easier to choose the appropriate level of GPU acceleration for your AI/ML workloads.
- Reduced operational overhead. Managed Kubernetes handles the everyday responsibilities of overseeing a Kubernetes cluster, like managing the control plane and implementing K8s updates. This enables you to focus on creating, deploying and managing AI/ML applications.
- Scalability and reliability. Managed K8s services are typically designed with a strong focus on scalability and reliability, ensuring that your AI/ML workloads can adeptly handle fluctuating traffic and spikes in resource demand.
Gcore Managed Kubernetes with NVIDIA GPU Workers
Gcore Managed Kubernetes helps you to deploy Kubernetes clusters fast, without the need to maintain the underlying infrastructure and Kubernetes backend. The Gcore team controls the master nodes while you control only the worker nodes, reducing your operational burden. Worker nodes can be Gcore Virtual Machines or Bare Metal servers in various configurations, including those with NVIDIA GPU modules.
Conclusion
Managed Kubernetes with GPU worker nodes is a powerful and flexible combination for accelerating AI/ML inference. By taking advantage of both Kubernetes and GPUs, managed Kubernetes with GPU worker nodes can help you improve the performance and efficiency of your AI/ML workloads. The service also frees you from the need to maintain the underlying GPU infrastructure and most Kubernetes components.
Gcore Managed Kubernetes can boost your AI/ML workloads with GPU worker nodes on Bare Metal for faster inference and operational efficiency. We offer a 99.9% SLA with free production management and free egress traffic—at outstanding value for money.
Related articles
Subscribe to our newsletter
Get the latest industry trends, exclusive insights, and Gcore updates delivered straight to your inbox.