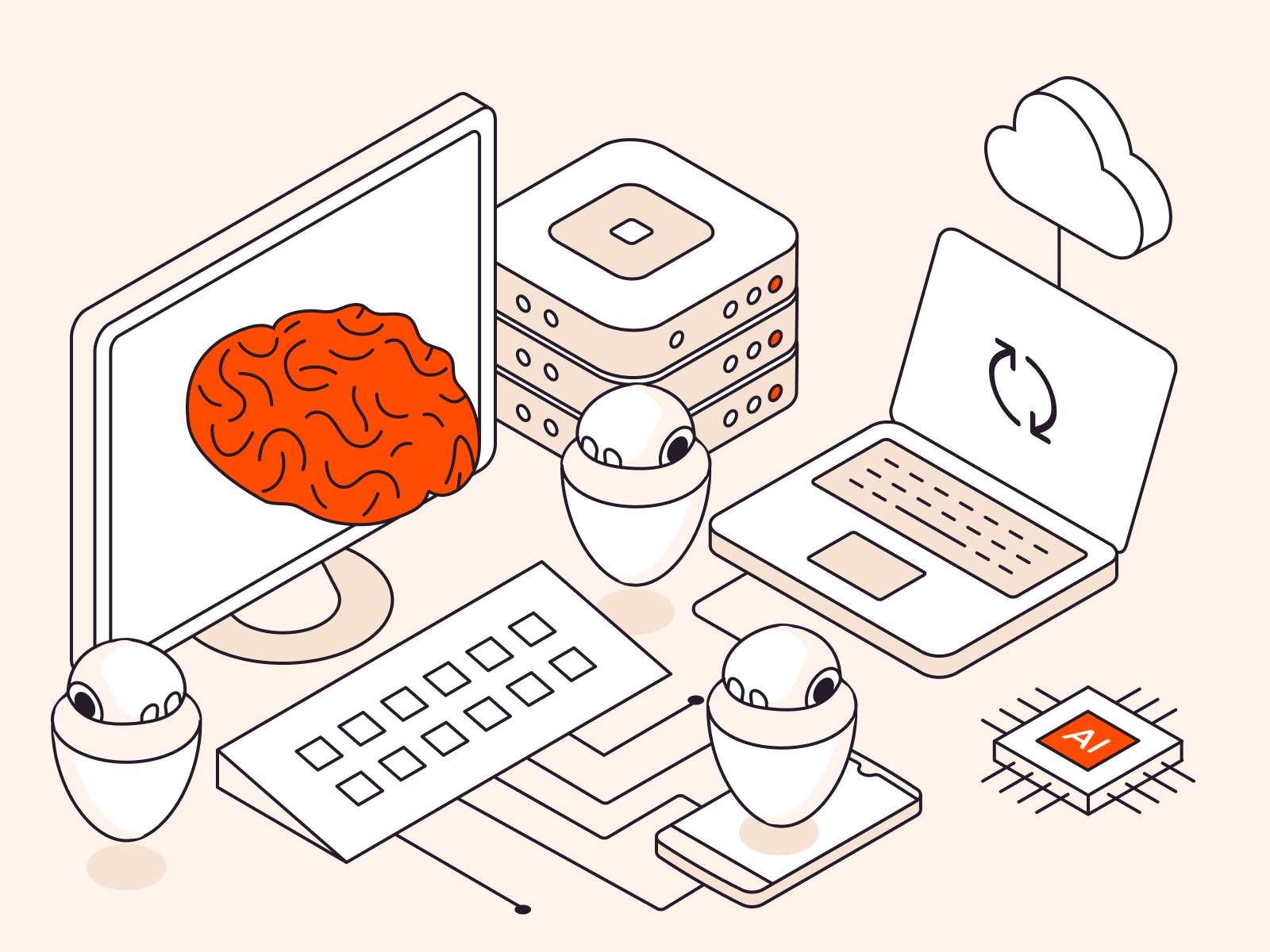
AI workloads are all the computational processes required to run AI behind the scenes, including during training and inference. These intensive workloads require massive computational resources, making them expensive, complex, and slow to run. At least, that’s the case when using a traditional cloud AI infrastructure.
Edge AI is a new technology that solves these challenges during the inference stage—this is called edge inference or inference at the edge. It decentralizes data processing and brings computation closer to end users, offering faster and more secure AI experiences. Read on to discover how edge inference solves six AI workload challenges for businesses.
#1 Challenge: Latency
High latency is a serious annoyance for AI end users. Think about staring at your screen waiting for a ChatGPT response or wasting time on slow customer service chatbots. The problem is even more severe in real-time applications like autonomous vehicles, finance, and video streaming. Slow AI means lost customers and failed apps.
While AI models contribute to lag, 33% of AI/ML latency is due to network slowness. To overcome the problem, AI workloads require low-latency, high-bandwidth connectivity between servers, storage, and the essential CPUs for processing.
Solution: Use an Edge Network
Deploy an edge network for AI inference to bring data processing closer to your end users. In this setup, edge points of presence are equipped with serious computational power and offer impressive connectivity, resulting in reduced latency and faster processing times for AI applications.
#2 Challenge: Bandwidth
Bandwidth is the volume of data that can be transmitted or processed in a given amount of time. It affects the speed, efficiency, and cost of AI operations. Transferring large datasets between data centers or from edge devices to central servers requires high bandwidth, resulting in high costs that are passed on to the business.
Traditional networks often struggle with the high bandwidth demands of AI workloads. As data volumes grow, network congestion can occur, causing delays and reducing processing efficiency.
Solution: Optimize Data Management and Storage
Edge AI localizes data storage, filters relevant data, and compresses it before transmission to optimize bandwidth. It performs real-time analytics and edge caching to minimize data sent to the cloud. The system places critical data closer to the edge through hierarchical storage, and predictive management anticipates data needs. These strategies reduce data transfer, conserve bandwidth, and boost efficiency and reliability.
#3 Challenge: Scalability
Scalability is essential to futureproofing your business’ AI applications. As your customer base grows, your AI app needs to respond to more requests without compromising on speed or incurring disproportionate costs.
Traditional centralized cloud infrastructures can become bottlenecks as the number of AI requests increases because all the data processing has to happen in one location. Imagine your website’s homepage offers AI-powered recommendations. During a Black Friday sale, service could grind to a halt as one server struggles to process the vast number of visitors to your site.
Solution: Implement Scalable Infrastructure
Edge inference distributes your AI workloads across numerous powerful GPUs strategically located close to your customers. On Black Friday, your customers are served AI recommendations from a nearby server, meaning each server gets a small portion of the total workload to process. Gcore Inference at the Edge runs over a 180+ point-of-presence network, meaning the AI workload gets distributed across hundreds of servers. No matter how many customers access your AI service, they still get quick responses. With Smart Routing and pay-as-you-go pricing, you don’t need to lift a finger as your company grows.
#4 Challenge: Infrastructure
AI needs compute power, and that’s supplied by its hardware—servers. GPUs are the most effective option here, as they’re designed with AI in mind. For example, the NVIDIA L40S was created for AI inference and outperforms other NVIDIA GPUs on inference tasks.
The problem is that with the sudden uptake of AI across industries, these GPUs can be hard to come by for businesses looking to buy outright. They’re also extremely costly.
Solution: Assess and Choose Appropriate Hardware
Edge inference can be outsourced to a specialist provider that sources, sets up, and maintains the hardware on your behalf. In this model, you automatically use and pay for the GPU compute you need at any given moment. By sharing GPU compute with other customers, you get all the processing power when you need it but without a huge price tag. Beyond saving you from a huge initial hardware investment, you also save the cost and hassle of hiring specialists to set up and maintain the hardware.
#5 Challenge: Ethics, Security, and Privacy
End users often input sensitive data into AI applications. For example, in the medical industry, a patient MRI may be processed to detect early-stage cancer. In retail, virtual try-ons may involve customers uploading images of themselves. These use cases raise concerns about privacy breaches and misuse of user data. As a result, regulations are being rolled out to protect users of AI apps, in addition to existing data processing requirements.
Solution: Implement Robust Security and Compliance Measures
Robust security measures must be implemented to support data privacy and regulatory compliance. Outsourcing your AI inference to a specialized provider means you don’t need to worry about these issues as long as you confirm that your provider is compliant.
Edge inference streamlines compliance because data is always processed locally. It’s relatively simple for edge inference providers to set rules so that, for example, data from users in Texas is always processed in Texas, even if a user is physically closer to an edge point of presence across the state border in New Mexico. This means your provider can make adhering to local regulations simpler.
Compare that to a traditional cloud model, where all your customers’ data goes to one central cloud location. Complying with increasingly complex requirements becomes difficult, if not impossible.
Next-Gen Edge AI Developments with Gcore
At Gcore, we’re at the forefront of next-generation AI developments. Gcore Inference at the Edge makes the benefits of edge AI accessible to businesses across industries:
- Gaming: Enhance your players’ interactions and game management with real-time analytics and responsive AI-driven content.
- Media and entertainment: Boost your users’ experience with personalized media consumption and real-time enhancements in live broadcasting.
- Technology: Streamline content creation and tech support for an efficient, superior user experience.
- Telecommunications: Improve service reliability and disaster management with real-time insights and low-latency response to keep your customers connected.
Gcore Inference at the Edge makes even the most complex and challenging AI workloads easy to manage and scale. Powered by NVIDIA L40S GPUs and a global network of 180+ PoPs, we ensure quick response times for your customers. You focus on your core business, we’ll handle your AI infrastructure.