AI is becoming increasingly ingrained in every facet of business. But how can organizations unlock its full potential? How can they confidently rely on AI for key business operations? Behind the buzz, a range of strong and silent workhorse technologies, such as Kubernetes, operate in the background to secure AI’s foundations and ensure its future success, longevity, and reliability within your company.
How Kubernetes Powers AI
Kubernetes (also known as K8s) is an open-source platform originally developed by Google and turned over to the open-source community in 2014. It deploys, automates, and manages containerized applications, i.e., it acts as a “data center,” which can eliminate many time-consuming manual processes for developers. You can think of Kubernetes as the conductor of an orchestra, keeping individual components (or sections) working as and when they should be.
When it comes to AI, K8s plays a key role throughout the entire AI lifecycle: development, training, and inference. K8s is particularly important in the latter two phases.
As of 2023, almost half of organizations were using Kubernetes for AI workloads. Reasons for this include scalability, cost-efficiency, and reliability. Let’s explore each in more depth.
1. Scalability
Every business experiences periods of higher and lower demand. For example, a US e-commerce site could get huge traffic volumes of customer service chatbot requests during a Black Friday sale but relatively low volumes during US overnight hours. Kubernetes auto-scales in response to fluctuating traffic, user requests, and volume of data being processed. It can handle complex needs and allow companies to resource accordingly without delay. This benefit is most significant in the inference phase because that’s where the greatest variance in workload is, since demand is user-generated. Training is much more predictable and controllable, so autoscaling isn’t as relevant. Training is also a discrete activity, whereas inference is constant as long as your AI app is available, so the potential long-term benefits of autoscaling for inference are almost infinite!
In addition, the adaptable and automated nature of K8s greatly simplifies workflow processes for engineers. Organizations can easily scale their infrastructure up or down in both the training and inference phases without the need to rely on human intervention to manage the physical hardware. This can reduce human resource costs for businesses.
2. Cost-Efficiency
The flexibility of Kubernetes is also beneficial for companies in financial terms. Training AI models, for example, is expensive and time-consuming, with the largest models predicted to cost over a billion dollars to train by 2027.
Kubernetes offers workload automation and enables you to allocate the computing resources needed dynamically and automatically. This saves you money because you only pay for the resources your AI workload actually uses. So, if your inference processing needs are lower on weekends, Kubernetes will automatically scale resources down in the week and up on weekends as demand fluctuates. Without this autoscaling, you’d have to pay according to weekend demand all week long, throwing away cash and, therefore, profit.
3. Reliability
While the world of AI is not new, the hype around it is. Kubernetes has been around for a decade and is, therefore, a relatively mature technology that companies can trust to manage their AI endeavors. Its stability and dependency enable developers to streamline processes and work more efficiently. In addition, Kubernetes is auto-healing, which means that it actively detects and resolves issues, minimizing downtime. That’s why, for many businesses, Kubernetes is the backbone of their company’s AI computing infrastructure.
What Does the Future Hold for K8s and AI?
There is still room for growth in terms of future functions of Kubernetes. The continued rise of AI means that cloud-based infrastructure will grow and evolve to meet new use cases. In terms of futureproofing your AI workload, integrating Kubernetes now to support the ever-growing range of AI use cases is more important and timely than ever.
Get the Power of K8s for AI with Gcore Managed Kubernetes
At Gcore, we make it simple to take advantage of the benefits Kubernetes provides for AI workloads. Gcore Managed Kubernetes is easy to deploy, manage, and scale based on your needs. Whether you’re looking to train a model with Gcore GPU Cloud or your app is ready to deploy via Gcore Inference at the Edge, we can help.
Our latest ebook, Accelerating AI with Kubernetes, provides a more technical, in-depth look at how Kubernetes benefits AI development, training, and inference.
Related articles
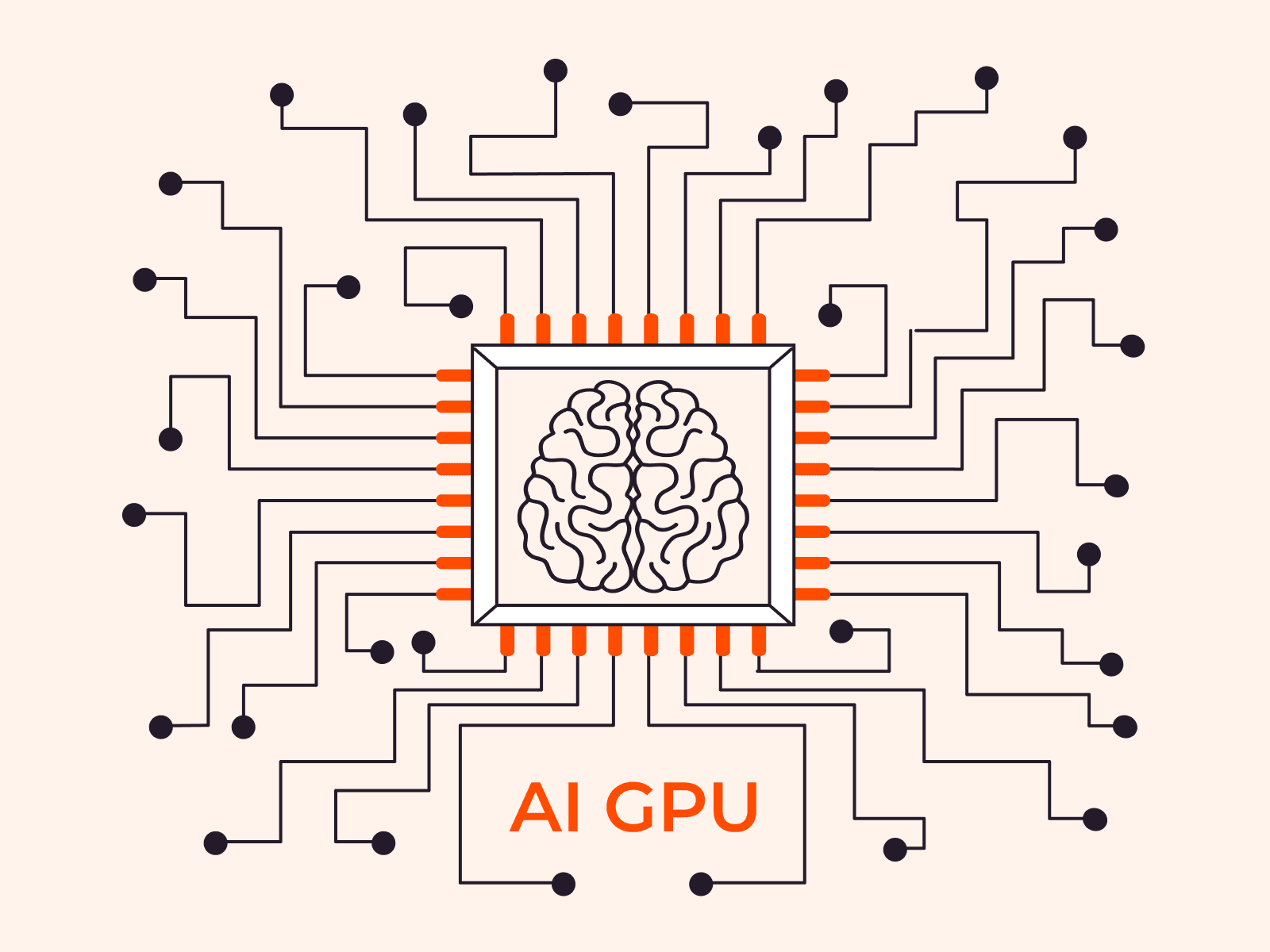
Announcing a new AI-optimized data center in Southern Europe
Good news for businesses operating in Southern Europe! Our newest cloud regions in Sines, Portugal, give you faster, more local access to the infrastructure you need to run advanced AI, ML, and HPC workloads across the Iberian Peninsula and wider region. Sines-2 marks the first region launched in partnership with Northern Data Group, signaling a new chapter in delivering powerful, workload-optimized infrastructure across Europe. And Sines-3 expands capacity and availability for the region.Strategically positioned in Portugal, Sines-2 and Sines-3 enhance coverage in Southern Europe, providing a lower-latency option for customers operating in or targeting this region. With the explosive growth of AI, machine learning, and compute-intensive workloads, these new regions are designed to meet escalating demand with cutting-edge GPU and storage capabilities.You can activate Sines-2 and Sines-3 for GPU Cloud or Everywhere Inference today with just a few clicks.Built for AI, designed to scaleSines-2 and Sines-3 bring with them next-generation infrastructure features, purpose-built for today's most demanding workloads:NVIDIA H100 GPUs: Unlock the full potential of AI/ML training, high-performance computing (HPC), and rendering workloads with access to H100 GPUs.VAST NFS (file sharing protocol) support: Benefit from scalable, high-throughput file storage ideal for data-intensive operations, research, and real-time AI workflows.IaaS portfolio: Deploy Virtual Machines, manage storage, and scale infrastructure with the same consistency and reliability as in our flagship regions.Organizations operating in Portugal, Spain, and nearby regions can now deploy workloads closer to end users, improving application performance. For finance, healthcare, public sector, and other organisations running sensitive workloads that must stay within a country or region, Sines-2 and Sines-3 are easy ways to access state-of-the-art GPUs with simplified compliance. Whether you're building AI models, running simulations, or managing rendering pipelines, Sines-2 and Sines-3 offer the performance, capacity, availability, and proximity you need.And best of all, servers are available and ready to deploy today.Run your AI workloads in Portugal todayWith these new Sines regions and our partnership with Northern Data Group, we're making it easier than ever for you to run AI workloads at scale. If you need speed, flexibility, and global reach, we're ready to power your next AI breakthrough.Unlock the power of Sines-2 and Sines-3 today
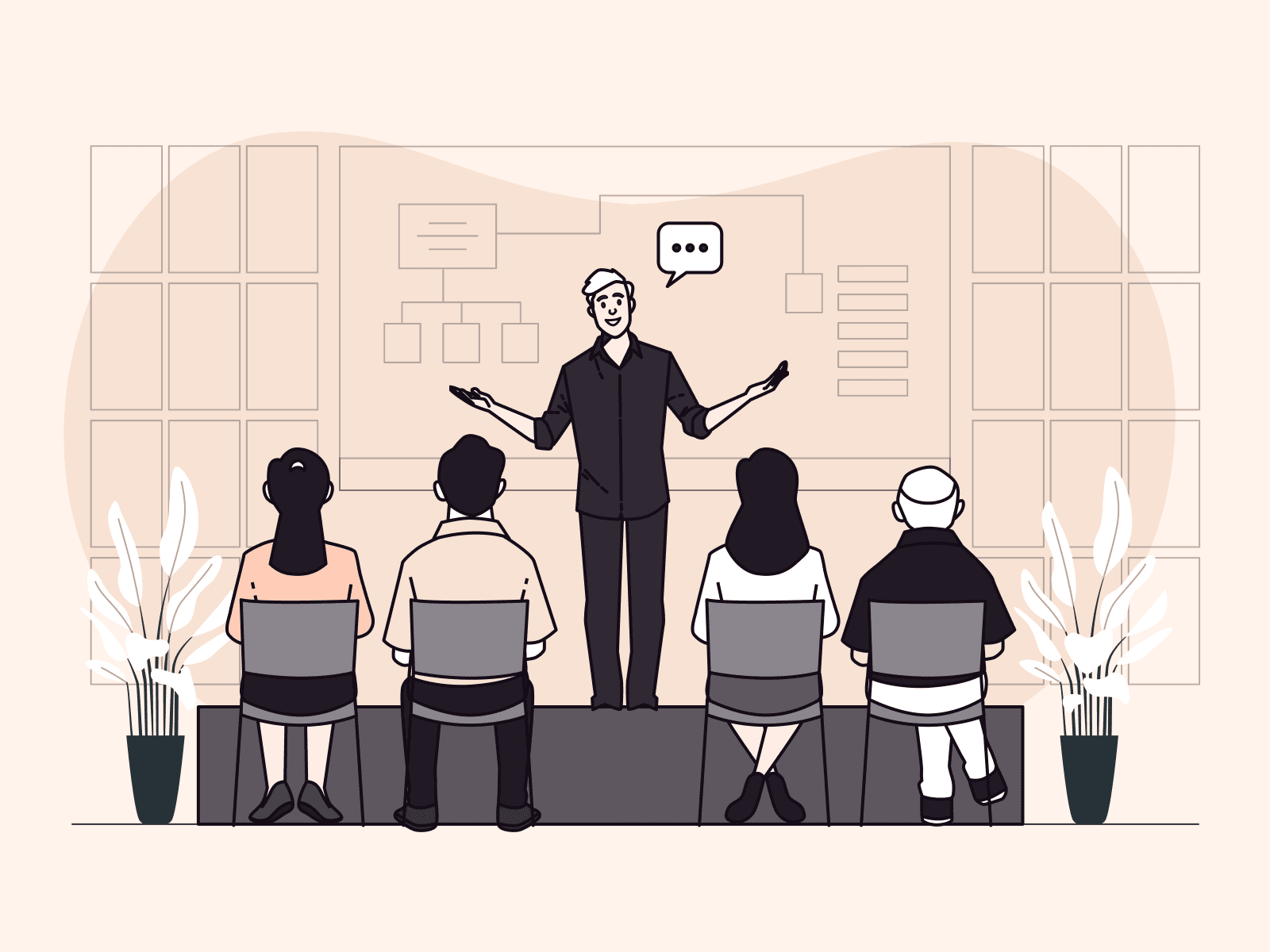
GTC Europe 2025: watch Seva Vayner on European AI trends
Inference is becoming Europe’s core AI workload. Telcos are moving fast on low-latency infrastructure. Data sovereignty is shaping every deployment decision.At GTC Europe, these trends were impossible to miss. The conversation has moved beyond experimentation to execution, with exciting, distinctly European priorities shaping conversations.Gcore’s own Seva Vayner, Product Director of Edge Cloud and AI, shared his take on this year’s event during GTC. He sees a clear shift in what European enterprises are asking for and what the ecosystem is ready to deliver.Scroll on to watch the interview and see where AI in Europe is heading.“It’s really a pleasure to see GTC in Europe”After years of global AI strategy being shaped primarily by the US and China, Europe is carving its own path. Seva notes that this year’s GTC Europe wasn’t just a regional spin-off. it marked the emergence of a distinctly European voice in AI development.“First of all, it's really a pleasure to see that GTC in Europe happened, and that a lot of European companies came together to have the conversation and build the ecosystem.”As Seva notes, the real excitement came from watching European players collaborate. The focus was less on following global trends and more on co-creating the region’s own AI trajectory.“Inference workloads will grow significantly in Europe”Inference was a throughline across nearly every session. As Seva points out, Europe is still at the early stages of adopting inference at scale, but the shift is happening fast.“Europe is only just starting its journey into inference, but we already see the trend. Over the next 5 to 10 years, inference workloads will grow significantly. That’s why GTC Europe is becoming a permanent, yearly event.”This growth won’t just be driven by startups. Enterprises, governments, and infrastructure providers are all waking up to the importance of real-time, regional inference capabilities.“There’s real traction. Companies are more and more interested in how to deliver low-latency inference. In a few years, this will be one of the most crucial workloads for any GPU cloud in Europe.”“Telcos are getting serious about AI”One of the clearest signs of maturity at GTC Europe was that telcos and CSPs are actively looking to deploy AI. And they’re asking the hard questions about how to integrate it into their infrastructure at a vast scale.“One of the most interesting things is how telcos are thinking about adopting AI workloads on their infrastructure to deliver low latency. Sovereignty is crucial, especially for customers looking to serve training or inference workloads inside their region. And also user experience: how can I get GPU capacity in clusters, or deliver inference in just a few clicks?”This theme—fast, sovereign, self-service AI—popped up again and again. Telcos and service providers want frictionless deployment and local control.“Companies are struggling most with data”While model deployment and infrastructure strategy took center stage, Seva reminds us that data processing and storage remains the bottleneck. Enterprises know they need to adopt AI, but they’re still navigating where and how to store and process the data that fuels it.“One of the biggest struggles for end customers is the data: where it’s processed, where it’s stored, and what kind of capabilities are available. From a European perspective, we already see more and more companies looking for sovereign data privacy and simple, mature solutions for end users.”That’s a familiar challenge for enterprises operating under GDPR, NIS2, and other compliance frameworks. The new wave of AI infrastructure has to be built for performance and for trust.AI in Europe: responsible, scalable, and localSeva’s key takeaway is that AI in Europe is no longer about catching up, it’s about doing it differently. The questions have changed from “Should we do AI?” to “How do we scale it responsibly, reliably, and locally?”From sovereign deployment to edge-first infrastructure, GTC Europe 2025 showed that inference is the foundation of how European businesses plan to run AI. “The ecosystem is coming together,” explains Seva. “And the next five years will be crucial for defining how AI will work: not just in the cloud, but everywhere.”If you’re looking to reduce latency, cut costs, and stay compliant while deploying AI in production, we invite you to download our free ebook, The inference optimization playbook.Download our free inference optimization playbook
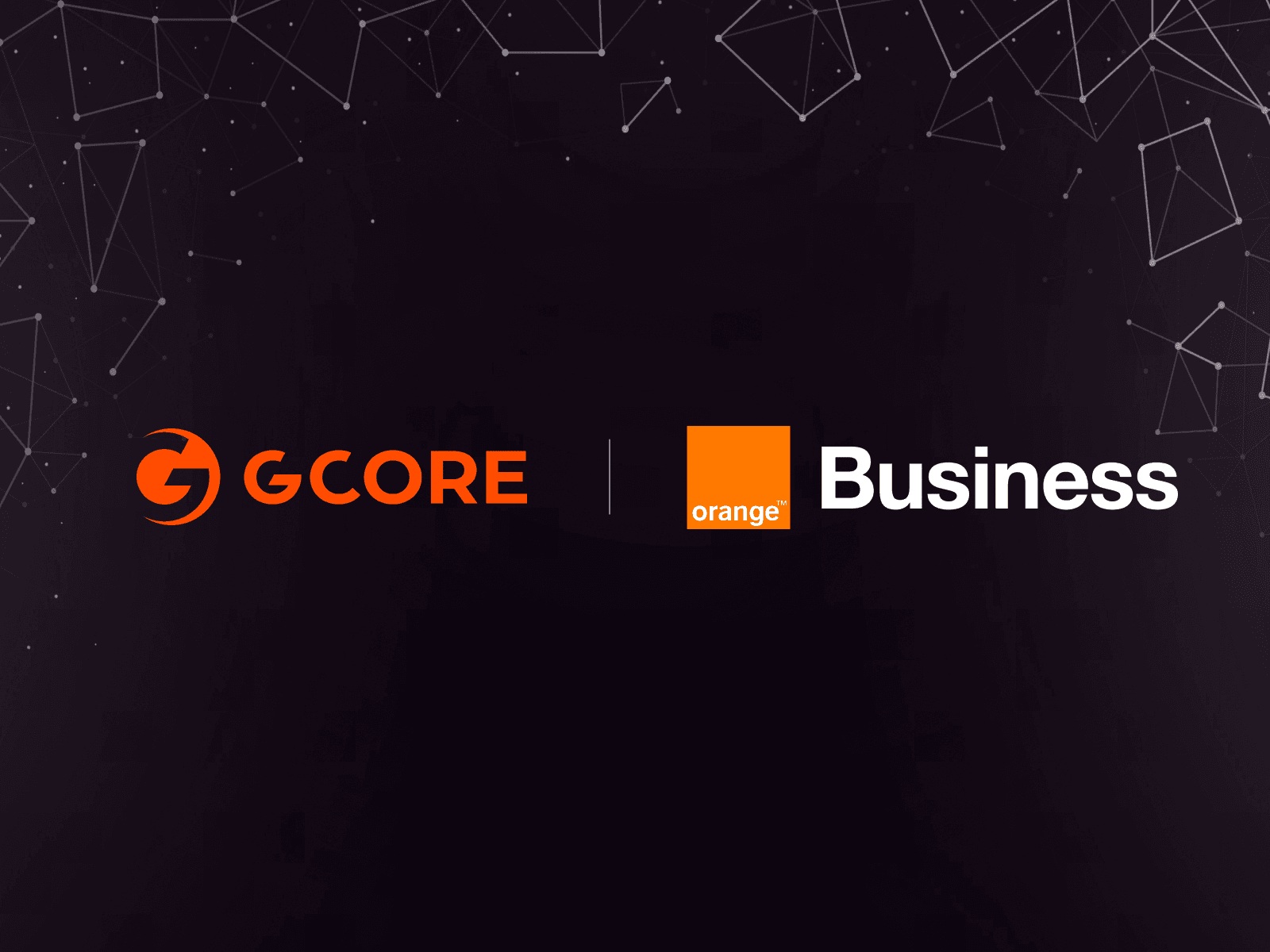
Gcore and Orange Business launch innovation program piloting joint solution to deliver sovereign inference as a service
Gcore and Orange Business have kicked off a strategic co-innovation program with the mission to deliver a scalable, production-grade AI inference service that is sovereign by design. By combining Orange Business’ secure, trusted cloud infrastructure and Gcore’s AI inference private deployment service, the collaboration empowers European enterprises and public sector organizations to run inference workloads at scale, without compromising on latency, control, or compliance.Gcore’s AI inference private deployment service is already live on Orange Business’ Cloud Avenue infrastructure. Selected enterprises across industries are actively testing it in real-world scenarios. These pilot customers are exploring how fast, secure, and compliant inference can accelerate their AI projects, cut deployment times, and reduce infrastructure overhead.The prototype will be demonstrated at NVIDIA GTC Paris, at the Taiga Cloud booth G26. Stop by any time to see it in action.The inference supercycle is underwayBy 2030, inference will comprise 70% of enterprise AI workloads. Telcos are well positioned to lead this shift due to their dense edge presence, licensed national data infrastructure, and long-standing trust relationships.Gcore’s inference solution provides a sovereign, edge-native inference layer. It enables users to serve real-time, GPU-intensive applications like agentic AI, trusted LLMs, computer vision, and predictive analytics, all while staying compliant with Europe’s evolving data and AI governance frameworks.From complexity to three clicksEnterprise AI doesn’t need to be hard. Deploying inference workloads at scale used to demand Kubernetes fluency, large MLOps teams, and costly trial-and-error.Now? It’s just three clicks:Pick a model: Choose from NVIDIA NIMs, open source, or proprietary libraries.Choose a region: Select one of Orange Business’ accredited EU data centers.Deploy: See your workloads go live in under 10 seconds.Enterprises can launch inference projects faster, test ideas more quickly, and deliver production-ready AI services without spending months on ML plumbing.Explore our blog to watch a demo showing how enterprises can deploy inference workloads in just three clicks and ten seconds.Sovereign by designAll model data, logs, and inference results are stored exclusively within Orange Business’ own data centers in France, Germany, Norway, and Sweden. Cross-border data transfer is opt-in only, helping ensure alignment with GDPR, sector-specific regulations, and the forthcoming EU AI Act.This platform is built for trust, transparency, and sovereignty by default. Customers maintain full control over their data, with governance baked into every layer of the deployment.Performance without trade-offsGcore’s AI inference solution avoids the latency spikes, cold starts, and resource waste common in traditional cloud AI setups. Key design features include:Smart GPU routing: Directs each request to the nearest in-region GPU, delivering real-time performance with sub-50ms latency.Pre-loaded models: Reduces cold start delays and improves response times.Secure multi-tenancy: Isolates customer data while maximizing infrastructure efficiency.The result is a production-ready inference platform optimized for both performance and compliance.Powering the future of AI infrastructureThis partnership marks a step forward for Europe’s sovereign AI capabilities. It highlights how telcos can serve as the backbone of next-generation AI infrastructure, hosting, scaling, and securing workloads at the edge.With hundreds of edge POPs, trusted national networks, and deep ties across vertical industries, Orange Business is uniquely positioned to support a broad range of use cases, including real-time customer service AI, fraud detection, healthcare diagnostics, logistics automation, and public sector digital services.What’s next: validating real-world performanceThis phase of the Gcore and Orange Business program is focused on validating the solution through live customer deployments and performance benchmarks. Orange Business will gather feedback from early access customers to shape its future sovereign inference service offering. These insights will drive refinements and shape the roadmap ahead of a full commercial launch planned for later this year.Gcore and Orange Business are committed to delivering a sovereign inference service that meets Europe’s highest standards for speed, simplicity, and trust. This co-innovation program lays the foundation for that future.Ready to discover how Gcore and Orange Business can deliver sovereign inference as a service for your business?Request a preview
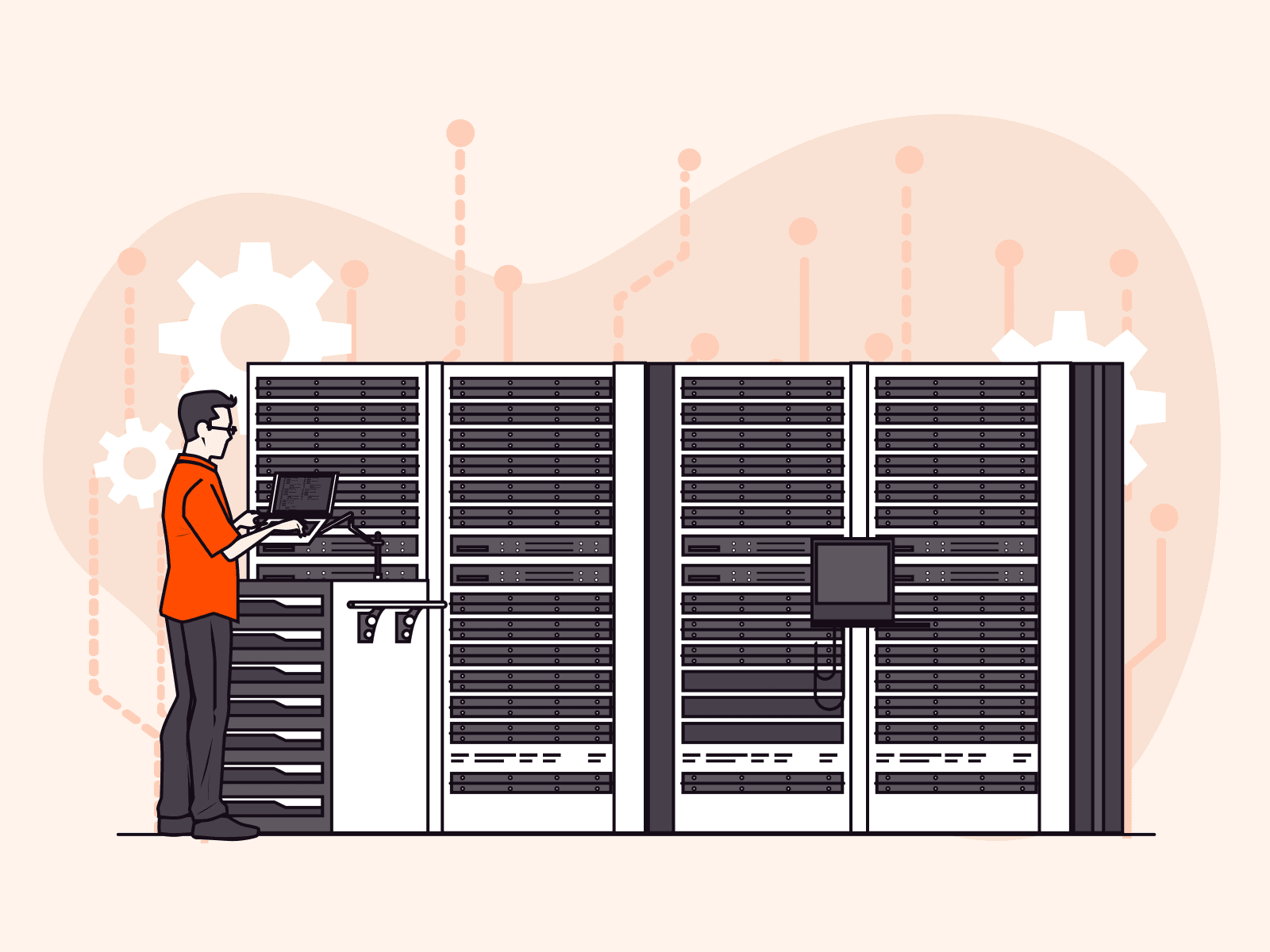
Why on-premises AI is making a comeback
In recent years, cloud AI infrastructure has soared in popularity. With its scalability and ease of deployment, it’s no surprise that organizations rushed to transfer their data to the cloud in a bid to become “cloud-first.”But now, the tide is turning.As AI workloads grow more complex and regulatory pressures increase, many companies are reconsidering their reliance on cloud and turning back toward on-premises AI infrastructure.Rather than doubling down on the cloud, organizations are diversifying—adopting multi-cloud models, sovereign cloud environments, and even hybrid or fully on-prem setups. The era of a single cloud provider handling everything is coming to an end. Why? Control, security, and performance are hard to find in the public cloud.Here’s why more businesses are bringing AI back in-house.#1 Enhanced data security and controlData security remains one of the most urgent concerns driving the return to on-prem infrastructure.For sensitive or high-priority workloads—common in sectors like finance, healthcare, and government—keeping data off the cloud is often non-negotiable. Cloud computing inherently increases risk by exposing data to shared environments, wider attack surfaces, and complex supply chains.Choosing a trusted cloud provider can mitigate some of those risks. But it can’t replace the peace of mind that comes from keeping sensitive data in-house.With on-premises AI, organizations gain fine-grained access control. Encryption keys remain internal and breach exposure shrinks dramatically. It’s also much easier to stay compliant with privacy laws when data never leaves your own secure perimeter.For industries where trust and confidentiality are everything, on-prem solutions offer full visibility into where and how data is stored and processed.#2 Performance enhancement and latency reductionLatency matters—especially in AI.On-premises AI systems excel in environments that require real-time performance and heavy compute loads. Processing data locally avoids the physical delays caused by transferring it across the internet to a cloud data center.By eliminating long-haul network hops, companies get near-instant access to computing resources. They also get to fine-tune their internal networks—using private fiber, low-hop switching, and other low-latency optimizations that cloud customers can’t control.Unlike multi-tenant cloud platforms, on-prem resources aren’t shared. That means consistently low, predictable latency.This is vital for use cases where milliseconds—or even microseconds—make a difference: autonomous vehicles, real-time analytics, robotic control systems, and high-speed trading. Fast feedback loops and localized processing enable better outcomes, tighter control, and faster decision-making at the edge.#3 Regulatory compliance and data sovereigntyAround the world, data privacy regulations are tightening. For most organizations, compliance isn’t optional.On-premises infrastructure helps keep data safely inside the organization’s network. This supports data sovereignty, ensuring that sensitive information remains subject only to local laws—not the policies of another country’s cloud provider.It's also a powerful hedge against geopolitical instability.While hyperscalers operate globally, they’re always headquartered somewhere. That makes their infrastructure vulnerable to political shifts, sanctions, or changes in international data law. Governments may require them to restrict access, share data, or cut off services entirely—especially to organizations in sanctioned or adversarial jurisdictions.Businesses relying on these providers risk disruption when regulations change. On-premises infrastructure, by contrast, offers reliable continuity and greater control—especially in uncertain times.#4 Cost control and operational benefitsCloud pricing may look flexible, but costs can escalate quickly.Data transfers, storage, and compute spikes all add up—fast. In contrast, on-premises infrastructure provides a predictable Total Cost of Ownership (TCO). Although upfront CapEx is higher, OpEx remains more stable over time.Organizations can invest in high-performance hardware tailored to their specific needs and amortize those costs across years. That means no surprise bills, no sudden price hikes, and no dependence on vendor pricing models.Of course, running on-prem infrastructure comes with its own challenges. It demands specialized teams for deployment, maintenance, and support. These experts are costly to recruit and retain—but they’re critical to ensure uptime, security, and performance.Still, for companies with relatively stable compute and storage needs, the long-term savings often outweigh the initial setup effort. On-prem also integrates more smoothly into existing IT workflows, without the need for internet access or additional network setup—another operational bonus.#5 Proactive threat detection and automated responsesOn-premises AI sometimes enables smarter, more customized security.Advanced platforms can continuously analyze live data streams using machine learning to detect anomalies and predict threats. When something suspicious is flagged, the system can respond instantly by quarantining data, blocking traffic, and alerting security teams.That kind of automation is essential for minimizing damage and downtime.With full infrastructure control, organizations can deploy bespoke monitoring systems that align with their threat models. Deep packet inspection, real-time anomaly detection, and behavioral analytics can be easier to configure and maintain on-prem than in shared cloud environments.These systems can also work seamlessly with WAAP and DDoS tools to detect and neutralize threats before they spread. The key is flexibility: whether on-prem or cloud-based, AI-driven security should adapt to your architecture and threat landscape, not the other way around.End-to-end visibility can give security teams a clearer picture and faster response options than generic, one-size-fits-all public cloud security tools.How to combine eon-premises control with cloud scalabilityLet’s be clear: on-premises AI isn’t perfect. It demands upfront investment. It requires skilled personnel to deploy and manage systems. And integrating AI into legacy environments takes thoughtful planning.But today’s tools are helping bridge those gaps. Modern platforms reduce the need for constant manual intervention. They support real-time updates to threat models and detection logic. As a result, security teams can spend more time on strategy and less on maintenance.Meanwhile, the cloud still plays an important role. It offers faster access to new tools, software updates, and next-gen GPU hardware.That’s why many organizations are opting for a hybrid model.Our recommendation: Keep your sensitive, high-priority workloads on-prem. Use the cloud for elastic scale and innovation. Together, they deliver the best of both worlds: performance, control, compliance, and flexibility.Secure your digital infrastructure with Gcore on-premises AI inferenceWhether you’re protecting sensitive data or running high-demand workloads, on-premises AI gives you the control and confidence you need. Securing sensitive data and managing high-demand workloads requires a level of control, performance, and predictability that only on-premises AI infrastructure delivers.Gcore Everywhere Inference Private Deployment makes it easier than ever to bring powerful serverless AI inference capabilities directly into your physical environment. Designed for scalable global performance, Everywhere Inference enables robust and secure multi-tenant AI inference deployments across on-prem and cloud environments, helping you meet data sovereignty requirements, reduce latency, and streamline deployment.Talk to us about your on-prem AI plans
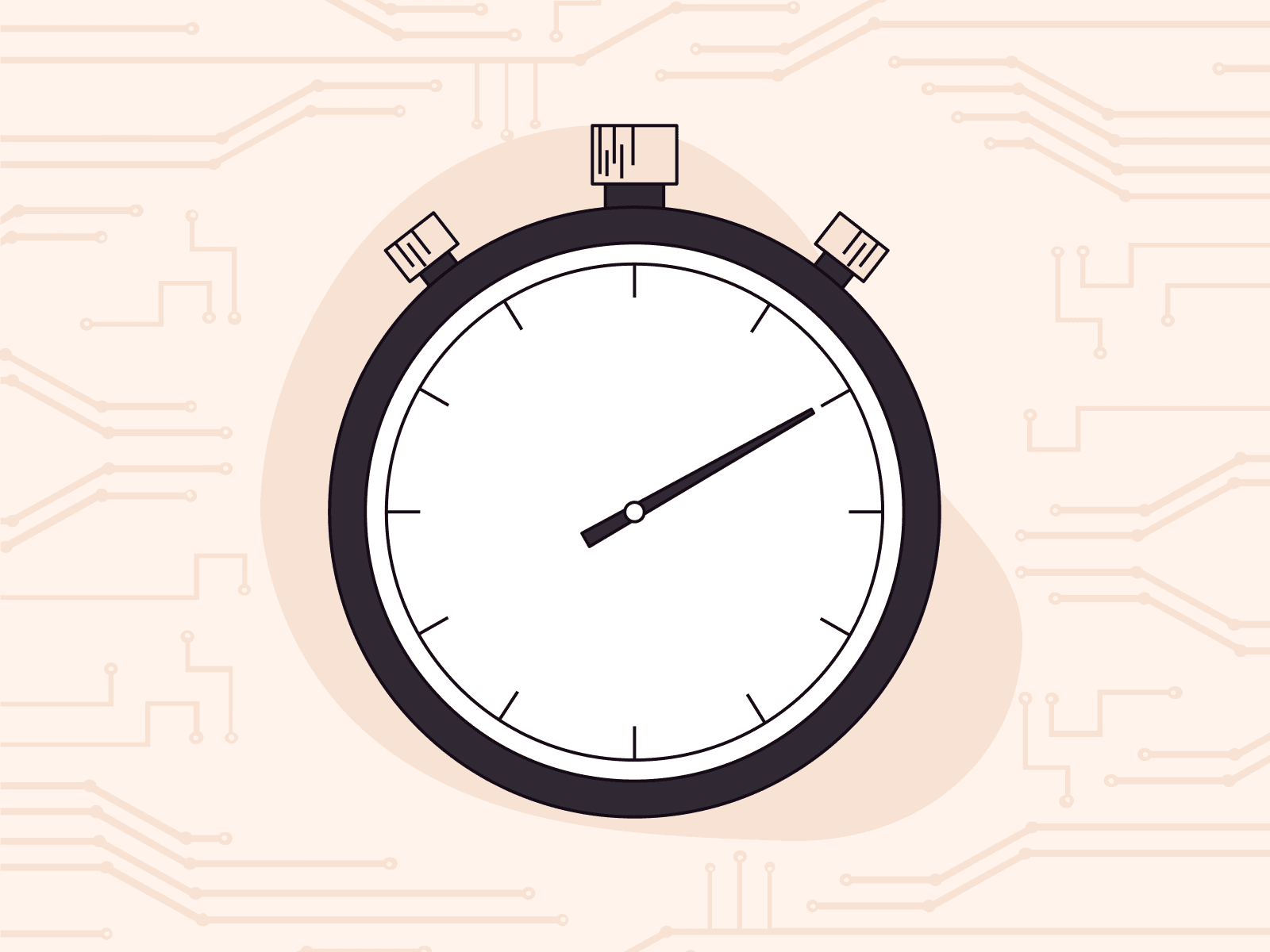
3 clicks, 10 seconds: what real serverless AI inference should look like
Deploying a trained AI model could be the easiest part of the AI lifecycle. After the heavy lifting of data collection, training, and optimization, pushing a model into production is where “the rubber hits the road”, meaning the business expects to see the benefits of invested time and resources. In reality, many AI projects fail in production because of poor performance stemming from suboptimal infrastructure conditions.There are, broadly speaking, two paths developers can take when deploying inference: DIY, which is time and resource-consuming and requires domain expertise from several teams within the business, or the ever-so-popular “serverless inference” solution. The latter is supposed to simplify the task at hand and deliver productivity, cutting down effort to seconds, not hours. Yet most platforms offering “serverless” AI inference still feel anything but effortless. They require containers, configs, and custom scripts. They bury users in infrastructure decisions. And they often assume your data scientists are also DevOps engineers. It’s a far cry from what serverless was meant to be.At Gcore, we believe real serverless inference means this: three clicks and ten seconds to deploy a model. That’s not a tagline—it’s the experience we built. And it’s what infrastructure leaders like Mirantis are now enabling for enterprises through partnerships with Gcore.Why deployment UX matters more than you thinkServerless inference isn’t just a backend architecture choice. It’s a business enabler, a go-to-market accelerator, an ROI optimizer, a technology democratizer—or, if poorly executed, a blocker.The reality is that inference workloads are a key point of interface between your AI product or service and the customer. If deployment is clunky, you’re struggling to keep up with demand. If provisioning takes too long, latency spikes, performance is inconsistent, and ultimately your service doesn’t scale. And if the user experience is unclear or inconsistent, customers end up frustrated—or worse, they churn.Developers and data scientists don’t want to manage infrastructure. They want to bring a model and get results without becoming cloud operators in the process.Dom Wilde, SVP Marketing, MirantisThat’s why deployment UX is no longer a nice-to-have. It’s the core of your product.The benchmark: 3 clicks, 10 secondsWe built Gcore Everywhere Inference to remove every unnecessary step between uploading a model and running it in production. That includes GPU provisioning, routing, scaling, isolation, and endpoint generation, all handled behind the scenes.The result is what we believe should be the default:Upload a modelConfirm deployment parametersClick deployAnd within ten seconds, you’re serving live inference.For platform teams supporting AI workloads, this isn’t just a better workflow. It’s a transformation.With Gcore, our customers can deliver not just self-service infrastructure but also inference as a product. End users can deploy models in seconds, and customers don’t have to micromanage the backend to support that.Dom Wilde, MirantisSimple frontend, powerful backendIt’s worth saying: simplifying the frontend doesn’t mean weakening the backend. Gcore’s platform is built for scale and performance, offering the following:Multi-tenant GPU isolationSmart routing based on location and loadAuto-scaling based on demandA unified API and UI for both automation and accessibilityWhat makes this meaningful isn’t just the tech, it’s the way it vanishes behind the scenes. With Gcore, Mirantis customers can deliver low-latency inference, maximize GPU efficiency, and meet data privacy requirements without touching low-level infrastructure.Many enterprises and cloud customers worry about underutilized GPUs. Now, every cycle is optimized. The platform handles the complexity so our customers can focus on building value.Dom Wilde, MirantisIf it’s not 3 clicks and 10 seconds, it’s not really serverlessThere’s a growing gap between what serverless inference promises and what most platforms deliver. Many cloud providers are focused on raw compute or orchestration, but overlook the deployment layer. That’s a mistake. Because when it comes to customer experience, ease of deployment is the product.Mirantis saw that early on and partnered with Gcore to bring inference-as-a-service to CSP and enterprise customers, fast. Now, customers can launch new offerings more quickly, reduce operational overhead, and improve the user experience with a simple, elegant deployment path.Redefine serverless AI with GcoreIf it takes a config file, a container, and a support ticket to deploy a model, it’s not serverless—it’s server-less-ish. With Gcore Everywhere Inference, we’ve set a new benchmark: three clicks and ten seconds to deploy AI. And, our model catalog offers a variety of popular models so you can get started right away.Whether you’re frustrated with slow, inefficient model deployments or looking for the most effective way to start using AI for your company, you need Gcore Everywhere Inference. Give our experts a call to discover how we can simplify your AI so you can focus on scaling and business logic.Let’s talk about your AI project
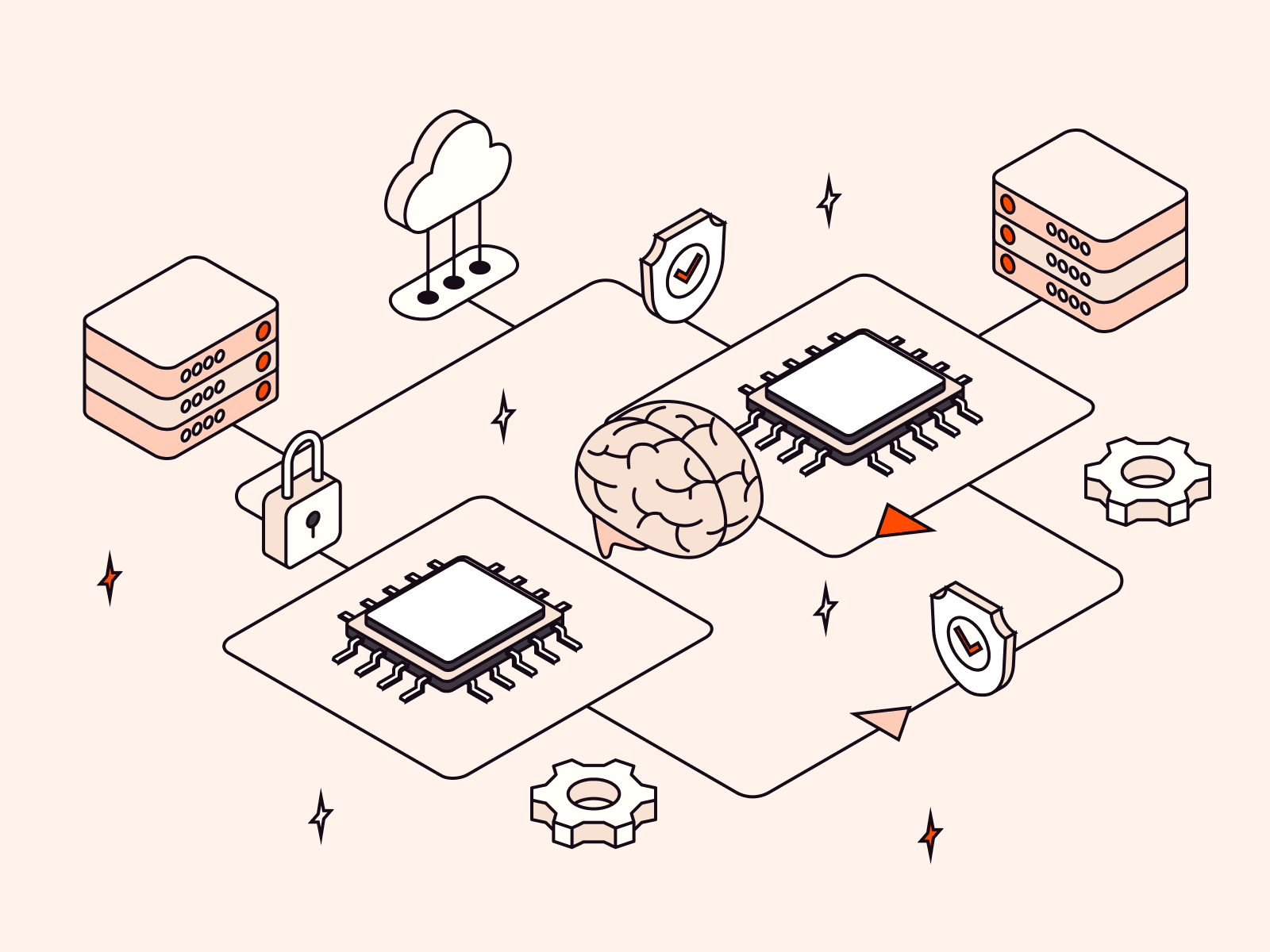
Run AI inference faster, smarter, and at scale
Training your AI models is only the beginning. The real challenge lies in running them efficiently, securely, and at scale. AI and reality meet in inference—the continuous process of generating predictions in real time. It is the driving force behind virtual assistants, fraud detection, product recommendations, and everything in between. Unlike training, inference doesn’t happen once; it runs continuously. This means that inference is your operational engine rather than just technical infrastructure. And if you don’t manage it well, you’re looking at skyrocketing costs, compliance risks, and frustrating performance bottlenecks. That’s why it’s critical to rethink where and how inference runs in your infrastructure.The hidden cost of AI inferenceWhile training large models often dominates the AI conversation, it’s inference that carries the greatest operational burden. As more models move into production, teams are discovering that traditional, centralized infrastructure isn’t built to support inference at scale.This is particularly evident when:Real-time performance is critical to user experienceRegulatory frameworks require region-specific data processingCompute demand fluctuates unpredictably across time zones and applicationsIf you don’t have a clear plan to manage inference, the performance and impact of your AI initiatives could be undermined. You risk increasing cloud costs, adding latency, and falling out of compliance.The solution: optimize where and how you run inferenceOptimizing AI inference isn’t just about adding more infrastructure—it’s about running models smarter and more strategically. In our new white paper, “How to Optimize AI Inference for Cost, Speed, and Compliance”, we break it down into three key decisions:1. Choose the right stage of the AI lifecycleNot every workload needs a massive training run. Inference is where value is delivered, so focus your resources on where they matter most. Learn when to use pretrained models, when to fine-tune, and when simple inference will do the job.2. Decide where your inference should runFrom the public cloud to on-prem and edge locations, where your model runs, impacts everything from latency to compliance. We show why edge inference is critical for regulated, real-time use cases—and how to deploy it efficiently.3. Match your model and infrastructure to the taskBigger models aren’t always better. We cover how to choose the right model size and infrastructure setup to reduce costs, maintain performance, and meet privacy and security requirements.Who should read itIf you’re responsible for turning AI from proof of concept into production, this guide is for you.Inference is where your choices immediately impact performance, cost, and customer experience, whether you’re managing infrastructure, developing models, or building AI-powered solutions. This white paper will help you cut through complexity and focus on what matters most: running smarter, faster, and more scalable inference.It’s especially relevant if you’re:A machine learning engineer or AI architect deploying models across environmentsA product manager introducing real-time AI featuresA technical leader or decision-maker managing compute, cloud spend, or complianceOr simply trying to scale AI without sacrificing controlIf inference is the next big challenge on your roadmap, this white paper is where to start.Scale AI inference seamlessly with GcoreEfficient, scalable inference is critical to making AI work in production. Whether you’re optimizing for performance, cost, or compliance, you need infrastructure that adapts to real-world demand. Gcore Everywhere Inference brings your models closer to users and data sources—reducing latency, minimizing costs, and supporting region-specific deployments.Our latest white paper, “How to optimize AI inference for cost, speed, and compliance”, breaks down the strategies and technologies that make this possible. From smart model selection to edge deployment and dynamic scaling, you’ll learn how to build an inference pipeline that delivers at scale.Ready to make AI inference faster, smarter, and easier to manage?Download the white paper
Subscribe to our newsletter
Get the latest industry trends, exclusive insights, and Gcore updates delivered straight to your inbox.