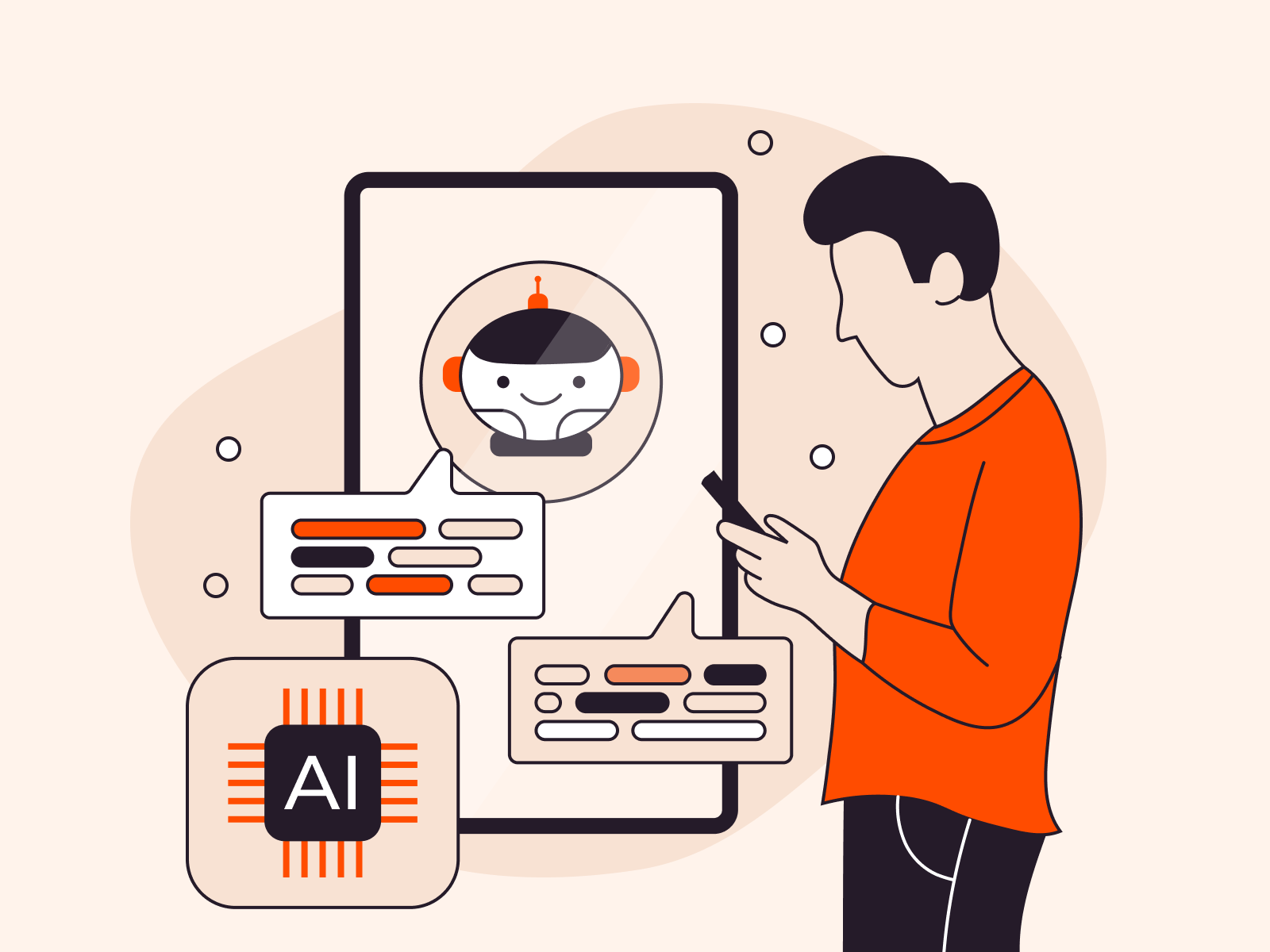
Businesses using AI are encountering a major challenge: latency. It’s frustrating for customers when they’re kept waiting for a chatbot to process their ecommerce return, stuck with a jittery, lagging game, or reading TV subtitles that are a few seconds behind the action.
Enter edge AI. This powerful technology minimizes latency by moving AI processing (called inference) physically closer to users with a distributed network of servers. By reducing latency, edge AI overcomes these customer frustrations, giving businesses that opt for edge AI a competitive edge. In this article we’ll look at three use cases for inference at the edge: gaming assets, automated captions and subtitles, and chatbots.
Edge AI Keeps Players in the Game
With one-third of the global population engaged in gaming, the gaming industry’s fixation on delivering the most immersive, responsive experiences possible comes as no surprise. One winning edge AI application is the real-time generation of in-game assets, like characters, environments, and UI elements.
The Challenge
High-quality game asset generation presents two major challenges:
- Their creation is time-consuming.
- They can cause latency for gamers.
As users demand faster, more intricate, and varied content, game developers are under pressure to offer immersive gaming experiences. This requires vast human resources, pushing up game costs and creating a punishing work schedule for development teams. Even once the AI is ready for use, cloud processing can cause latency issues, disrupting the player’s experience.
The Solution
Latency is easily improved with edge AI. By moving inference physically closer to gamers, they get faster response times compared to traditional cloud inference. Games can adapt to gamers’ decisions in real time with edge AI. When a player enters a new area or completes a challenge, the AI dynamically creates new landscapes, structures, and interactive elements, making the game world feel more responsive and immersive.
Using inference at the edge during the development process can reduce the pressure on developers to create vast quantities of complex assets:
- Generative AI and Large Language Models (LLMs) can streamline narrative generation, dialogue systems, and player support, improving overall game quality and player engagement.
- Reinforcement learning algorithms can train virtual characters to perform actions by learning from real-world motion capture data. This creates lifelike animations, enhancing the game’s realism. Retrieval-augmented generation (RAG) can also be used to optimize LLM outputs by referencing a knowledge base before generating responses, ensuring accuracy and relevance.
Real-Life Example: RAG in Pokémon
RAG is used in the Pokémon game to allow players to interact with a smart assistant that knows all about the Pokémon universe. When a player asks about a Pokémon, RAG searches through a database to find accurate details before generating a response. This means if a player wants to know about Bulbasaur’s moves, the assistant retrieves the exact information from the game’s data and responds accurately, enhancing the player’s knowledge and strategy. Using edge AI would optimize the speed with which gamers are served that information. In this way, the game ensures that the assistant provides precise and relevant information, making it easier for players to “catch ‘em all” and enjoy a more immersive and informed gaming experience.
The Results
Real-time asset generation with edge AI ensures smooth, uninterrupted gameplay and immediate feedback. Edge AI makes the gaming experience more interactive, responsive, and personalized, resulting in higher player satisfaction and longer play sessions.
Benefits of Edge Inference | Risks if Edge Inference is not used |
Real-time in-game asset generation (characters, environments) | Delays in rendering assets, disrupting gameplay |
Intelligent non-player characters (NPCs) | Poor NPC responsiveness |
Enhanced realism with dynamic updates | Lower quality graphics, less immersive gaming experiences |
Instant adaptation to player actions | Static game environments that do not react to players |
Edge AI Produces a Blockbuster Impact on Video Entertainment
The media and entertainment industry is valued at over $2.5 trillion with a steady growth trajectory, serving a global audience that wants to be entertained with zero lag, downtime, or constraints. One way edge AI can improve content in a global media market is by automating live transcription and translation, providing captions and subtitles in real time. This technology has the potential to significantly improve engagement:
- 80% of viewers are more likely to watch a full video if it has captions
- 69% of viewers choose to keep their video sound off when in public places
- 50% of consumers always prefer to consume UGC video content with the sound off
Businesses that adopt captions and subtitles stand to capture a larger market share and, if they add automated translation, can increase their reach to span the globe.
The Challenge
In traditional media distribution, transcriptions and translations were created by humans and added prior to release. But today, the pure quantity of video produced and the prevalence of live streams add two challenges:
- Automating transcription and translation to minimize the need for human resources and democratize access to these features
- Minimizing lag so captions/subtitles are aligned with the live video content
Cloud-based translation methods often face high costs and delays, impacting user engagement and satisfaction. Lag means audiences miss timely content during live events, leading to a disconnected and unsatisfying viewing experience.
The Solution
Edge AI can deliver real-time transcription and translation. These models are trained on massive language datasets and natural language processing technology to understand and translate content accurately.
Instead of processing audio and video streams in the cloud and dealing with latency, the models run on edge points of presence located geographically close to viewers. This approach allows the platform to generate real-time subtitles and dubs that sync with the original or live video.
The Results
Edge inference means translations are generated and displayed almost in real-time as video content plays, allowing viewers to instantly grasp what’s happening without missing a beat. When viewers can easily follow content in their language, they’re more likely to stay engaged and satisfied. This improved experience leads to higher viewer retention and fewer subscription cancellations.
Benefits of Edge AI | Risks if Edge AI is not used |
Immediate translation of live content | Delays in translation leading to disengagement |
Enhanced viewer experience with real-time subtitles | Viewers missing critical information due to lag |
Near-localized processing improves privacy | Higher risk of data breaches |
Reduced data transfer costs | Increased costs and dependency on cloud infrastructure |
Ability to cater to a global, multilingual audience | Limited reach and lower viewer retention |
Edge AI Supports Efficient Customer Service
AI has the potential to reduce customer service costs by up to 30%, making it an attractive solution for companies across industries. Gen AI has already proven its value in this use case. It can offer customer service via chatbots and virtual assistants.
The Challenge
Tech companies struggle to provide high-quality AI customer support:
- Latency issues with cloud-based systems can delay responses, frustrating customers.
- Managing a high volume of inquiries demands significant resources, increasing operational costs.
- Limitations with older AI models, such as inaccurate interpretation, required human intervention.
Companies need an efficient solution to offer instant support, handle complex queries, and manage growing customer demands without breaking the bank.
The Solution
Because edge AI operates close to customers, it can respond in near real time. This is a significant upgrade from traditional cloud-based systems, which can have response times upward of 500 milliseconds.
Edge AI can power advanced virtual assistants and chatbots, offering 24/7 support and reducing the need for human intervention. Edge AI inference systems can support models that escalate complex queries to human agents when necessary, ensuring accurate and efficient handling of complicated issues.
The Results
Processing data close to customers ensures inquiries are handled almost instantly. This greatly improves the customer support experience compared to slower cloud inference or waiting for a human agent to become available. It also democratizes business access to 24/7, high-quality customer support, allowing businesses to serve global customers instantly.
Automating routine customer service tasks with AI reduces the workload on human agents, so they can focus on more complex issues. This also leads to significant cost savings by reducing the need for extensive human resources
Keeping data closer to where it’s used enhances security by reducing the risk of breaches, as personal data doesn’t need to travel to distant servers. That’s a major benefit for retail companies that may need to request personal information such information during customer service interactions.
Benefits of Edge AI | Risks if Edge AI is not used |
Instant response to customer inquiries | Delayed responses |
Improved data security with near-local processing | Increased risk of data breaches |
Scalable support system handling complex queries | Inability to scale support as demand grows |
Boost Your Business with Gcore Inference at the Edge
Gcore powers edge AI with Inference at the Edge. This service brings AI inference close to your users with 180+ points of presence in 95+ countries, cutting down on delays and enabling super-fast responses for real-time AI applications. We manage all the infrastructure, so you can enjoy the business boost of edge inference without any hassle.
Experience the following benefits with Gcore Inference at the Edge:
- Flexible model deployment: Easily run open-source models, fine-tune exclusive models, or deploy custom ones. Whether you’re using a pretrained model or creating a new one, you can choose the best approach for your needs.
- Powerful GPU infrastructure: Boost your model performance with NVIDIA L40S GPUs, designed specifically for AI inference. These GPUs are available as dedicated instances or serverless endpoints, giving you the power needed to handle complex AI workloads efficiently.
- A low-latency global network: With over 180 strategically located edge points of presence (PoPs) and an average network latency of just 30 milliseconds, we ensure your AI applications deliver fast responses no matter where in the world your users are located.
- A single endpoint for global inference: Seamlessly integrate your models into applications and easily automate infrastructure management. Our single endpoint simplifies deployment, making managing and scaling your AI solutions globally straightforward.
- Model autoscaling: Our infrastructure dynamically scales based on user demand, so you only pay for the compute power you use. This helps you manage costs while ensuring you always have the resources needed to meet demand.
- Security and compliance: Benefit from integrated DDoS protection and compliance with GDPR, PCI DSS, and ISO/IEC 27001 standards. We ensure your data and applications are secure and meet the highest regulatory requirements.
If you’re ready to transform your AI workloads, consider Gcore Inference at the Edge.