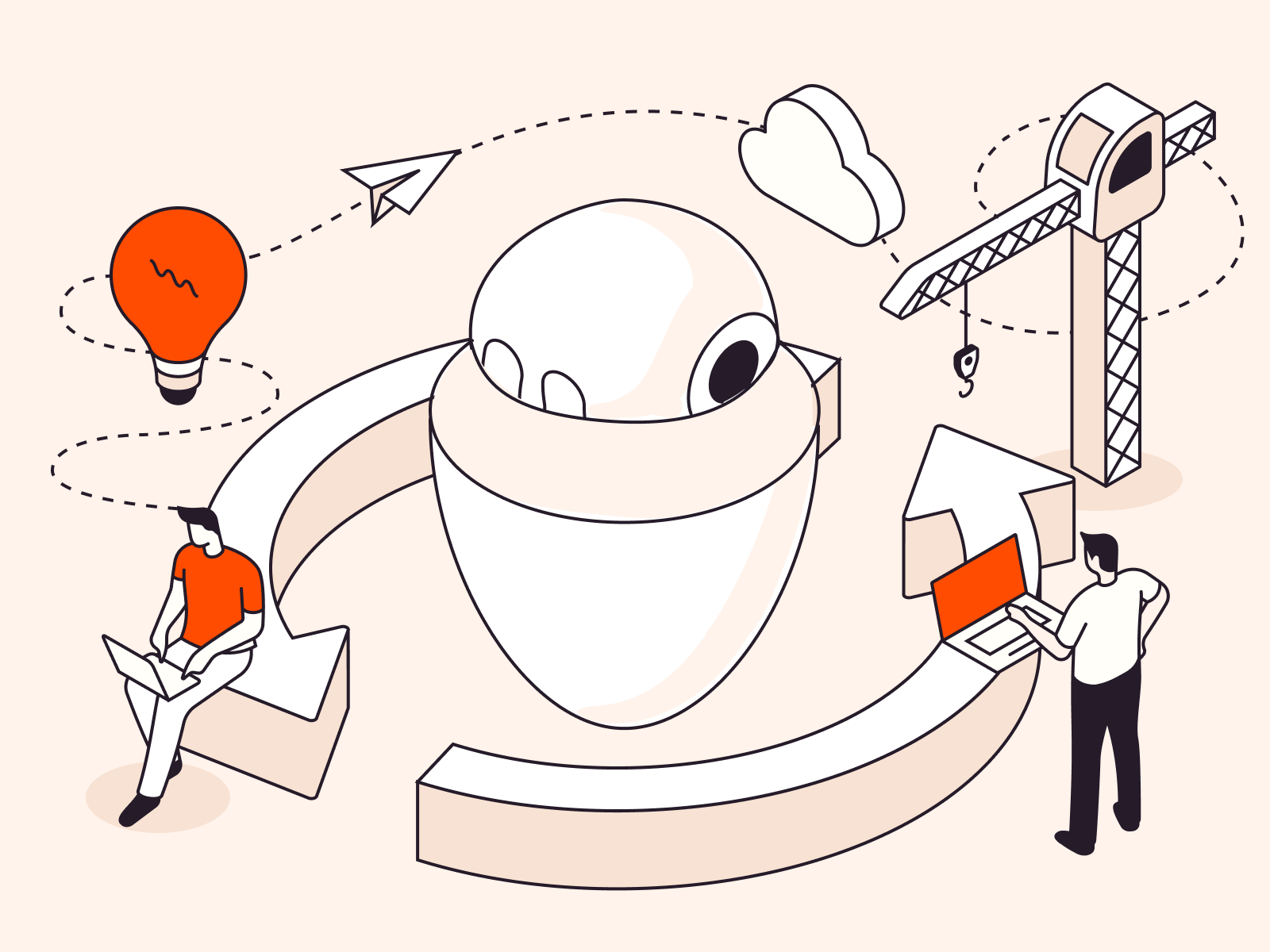
In today’s competitive market, many companies are looking to leverage Artificial Intelligence to tailor services perfectly to each customer’s preferences and improve their user experience. However, fine-tuning an AI model to operate flawlessly can be daunting. Throughout the AI lifecycle, companies encounter numerous problems, from development to deployment and maintenance. In this article, we simplify this complex journey, walking you through the essential stages from start to finish. Learn some practical tips and standards to help improve your AI projects, ensuring they are innovative, responsibly managed, and in line with regulations.
What Is an AI Lifecycle
The AI lifecycle refers to the complete approach of developing, deploying, and managing AI models that can transform business operations. It starts from initial data collection and proceeds through model building, validation, deployment, and ongoing maintenance. This structured approach is crucial for companies looking to harness AI effectively, assuring that their AI systems are not only innovative but also practical and scalable.
A recent Gartner study indicates a slight increase in AI projects successfully transitioning from pilot to production, with 54% achieving this milestone. There are many factors crucial to achieving this transition, and one of them is adhering to a structured process. That’s why it’s important to follow a disciplined approach to bring your AI projects to life. In the next section, let’s take a look at the importance of AI lifecycle management and how it can further enhance the success of your projects.
Importance of AI Lifecycle Management
Managing the AI lifecycle effectively is key to making sure AI systems work well and achieve their intended results. Here’s why it matters:
- Ensures Alignment with Business Objectives. Proper management ensures that AI initiatives support strategic business goals, not just technical achievements.
- Improves Model Performance. Regular updates and maintenance prevent issues like model drift, where a model’s performance degrades over time due to changes in underlying data patterns.
- Mitigates Risks. It helps in identifying and correcting biases in AI models, ensuring compliance with regulatory requirements, and safeguarding against ethical concerns.
- Enhances Scalability. Managing the AI lifecycle effectively allows organizations to scale their AI solutions more efficiently, adapting to increased demands or expanding the scope of AI applications.
- Drives Innovation. Continuous monitoring and updating open opportunities for improvements and innovations, keeping the AI solutions at the forefront of technology.
Now that we’ve learned about the importance of AI lifecycle management, let’s move on to explore the stages of the AI lifecycle to better understand how to successfully navigate each phase.
What Are the Stages of the AI Cycle
The AI model cycle is a structured framework that is crucial for the successful implementation and scaling of AI technologies within an organization. Here’s an overview of each stage:
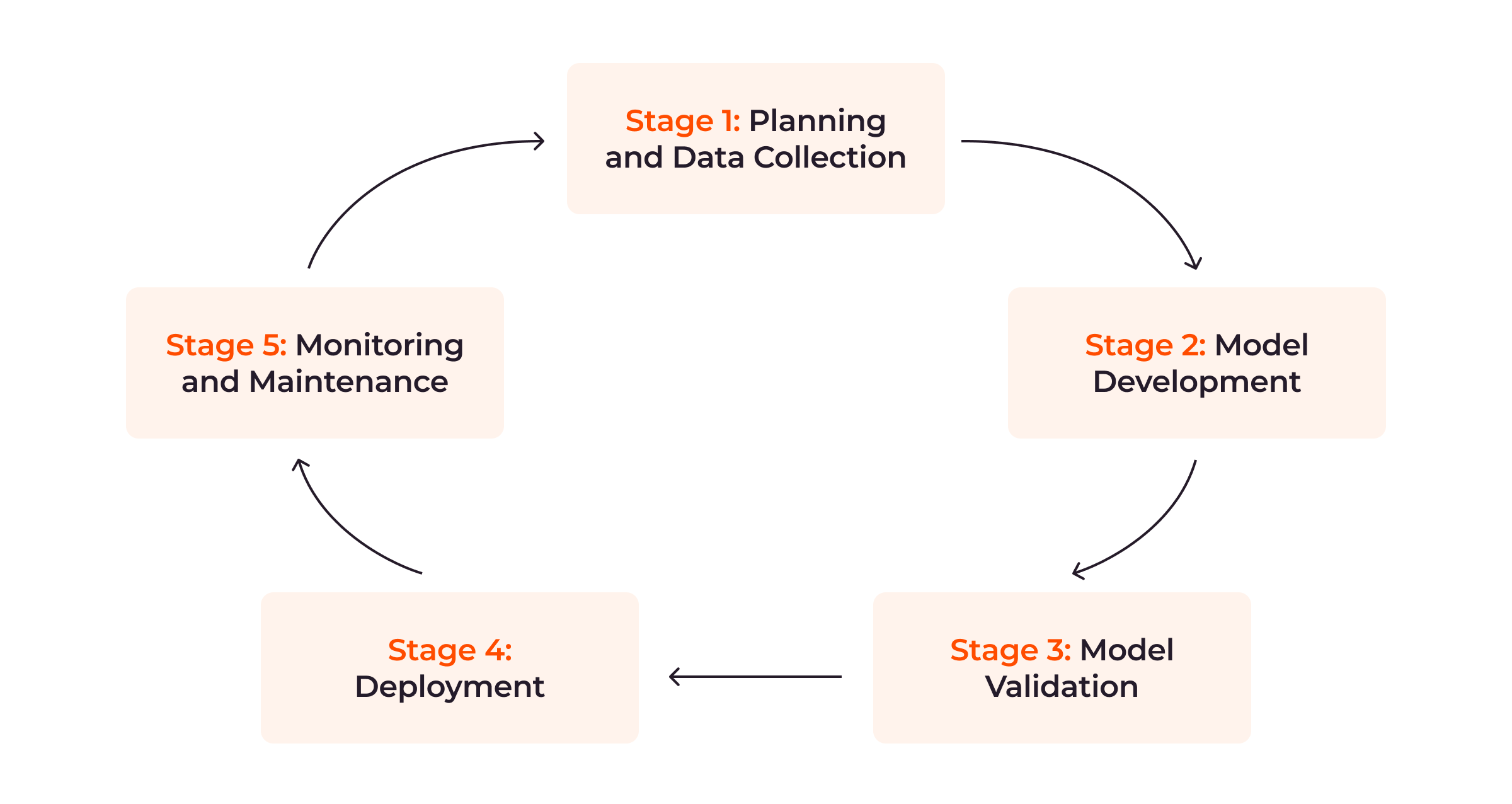
Stage 1: Planning and Data Collection
The foundation of any successful AI project begins with a clear understanding of the business problems it aims to solve and the data requirements necessary for the solution. This stage involves identifying these elements and gathering the relevant data, followed by meticulous preparation and cleaning of the data for analysis.
Stage 2: Model Development
Once the data is ready, the next step is designing and training machine learning models. This stage focuses on selecting appropriate modeling techniques and utilizing the right tools to develop models that are both effective and efficient. The choice of methods can vary widely, depending on the specific needs and goals of the project.
Stage 3: Model Validation
After model development, it is crucial to validate the models to ensure they perform as expected. This stage involves rigorous testing of the models using various methods to assess their accuracy and generalizability. Special attention is paid to avoiding overfitting, ensuring the models can perform well on new, unseen data.
Stage 4: Deployment
Deployment involves integrating the validated models into business processes. This stage requires strategic planning to address potential operational challenges and to ensure that the AI models can be seamlessly incorporated into existing systems. It’s critical to consider both the technical and business implications of deploying AI models.
Stage 5: Monitoring and Maintenance
The final stage of the AI model cycle involves the continuous monitoring of model performance once deployed. This includes regularly assessing how the models perform in the live environment and making necessary adjustments and updates based on performance data. This ongoing maintenance is vital to adapt to changes over time and to sustain the effectiveness of the AI applications.
Each stage of the AI model cycle is interconnected and crucial for transforming raw data into actionable insights and achieving the intended outcomes. By carefully managing each stage, organizations can optimize the impact of their AI investments. In the next section, we’ll explore ModelOps and discuss why it’s important for the effective operation of AI models.
ModelOps: Bridging Development and Operations
ModelOps is a vital framework that facilitates the efficient navigation and management of the AI model lifecycle within large organizations. By streamlining the implementation of AI and machine learning solutions, it addresses the scaling challenges posed by the need to manage numerous applications across an enterprise. Specifically, ModelOps focuses on the operationalization of AI models, emphasizing the processes, tools, and methodologies used to deploy, monitor, manage, and govern AI models effectively in production environments.
Key components of ModelOps in supporting the AI model lifecycle include:
- Governance. Ensuring that AI models are managed responsibly throughout their lifecycle.
- Scalability. Aiding in the expansion of AI applications to meet a wide array of enterprise needs.
- Lifecycle Management. Facilitating the stages of the AI model lifecycle, from development to deployment, including integration, testing, and version control.
- Adaptability. Including various AI technologies to ensure thorough quality and adherence to standards.
In essence, ModelOps underpins the entire AI model lifecycle, providing the necessary structure and processes to unite teams and streamline AI initiatives, from their inception to their integration into production and beyond.
What are Advanced Considerations in the AI Model Cycle
As AI continues to evolve, so do the complexities associated with its implementation and management. Advanced considerations in the AI model cycle are crucial for organizations aiming to navigate these challenges effectively. Here are some key areas to focus on:
- Addressing Ethical Considerations and Bias in AI Models. Ethical considerations and bias in AI models have gained significant attention in recent years. Organizations must proactively address these issues to ensure fairness, transparency, and accountability in their AI systems. This involves implementing ethical guidelines, conducting thorough audits of AI algorithms, and integrating diverse perspectives into the development process.
- Regulatory Compliance and its Impact on the AI Model Lifecycle. Compliance with regulations such as GDPR, CCPA, and HIPAA is essential for organizations deploying AI models, particularly in industries dealing with sensitive data. Compliance requirements impact various stages of the AI model lifecycle, from data collection and processing to model deployment and monitoring. Organizations need to stay updated on regulatory changes and implement robust compliance measures accordingly.
- Leveraging AI for Competitive Advantage Across Different Industries. AI presents significant opportunities for organizations to gain a competitive edge in various industries, from healthcare and finance to retail and manufacturing. Leveraging AI effectively requires a deep understanding of industry-specific challenges and opportunities, as well as the ability to develop customized AI solutions that address unique business needs. Organizations must continually innovate and adapt their AI strategies to stay ahead of the competition.
Consideration | Impact on AI Model Lifecycle |
Ethical Considerations and Bias | Ensures fairness and transparency in AI models. Requires thorough audits and diverse perspectives in development |
Regulatory Compliance | Impacts data collection, processing, and model deployment. Requires staying updated on regulatory changes and implementing robust compliance measures |
Leveraging AI for Competitive Advantage | Presents opportunities for gaining a competitive edge. Requires industry-specific understanding and customized AI solutions |
By integrating these considerations into their AI strategies, organizations can build trust, ensure compliance, and leverage AI to drive innovation and success across different industries.
Conclusion
Organizations that are exploring AI solutions need to navigate the complex landscape of AI lifecycle management and implement ModelOps strategically. This approach is not only about efficiency, but it also ensures that ethical considerations are met and regulatory compliance is adhered to, which helps to build trust and credibility. With this being said, Gcore is at the forefront of edge AI’s technological evolution, deploying AI across a global network designed to minimize latency and maximize performance across AI training, inference, and applications. Using advanced NVIDIA GPUs, Gcore Edge AI provides a robust, cutting-edge platform for large AI model deployment.