Transitioning AI inferencing from the cloud to the edge enhances real-time decision making by bringing data processing closer to data sources. For businesses, this shift significantly reduces latency, directly enhancing user experience by enabling near-instant content delivery and real-time interaction. This article explores edge AI’s business benefits across various industries and applications, emphasizing the importance of immediate data analysis in driving business success.
How Does AI Inference at the Edge Impact Businesses?
Deploying AI models at the edge means that during AI inference, data is processed on-site or near the user, enabling real-time, near-instant data processing and decision making. AI inference is the process of applying a trained model’s knowledge to new, unseen data, becomes significantly more efficient at the edge. Low-latency inference provided by edge AI is essential for businesses that rely on up-to-the-moment data analysis to inform decisions, improve customer experiences, and maintain a competitive edge.
How It Works: Edge vs Cloud
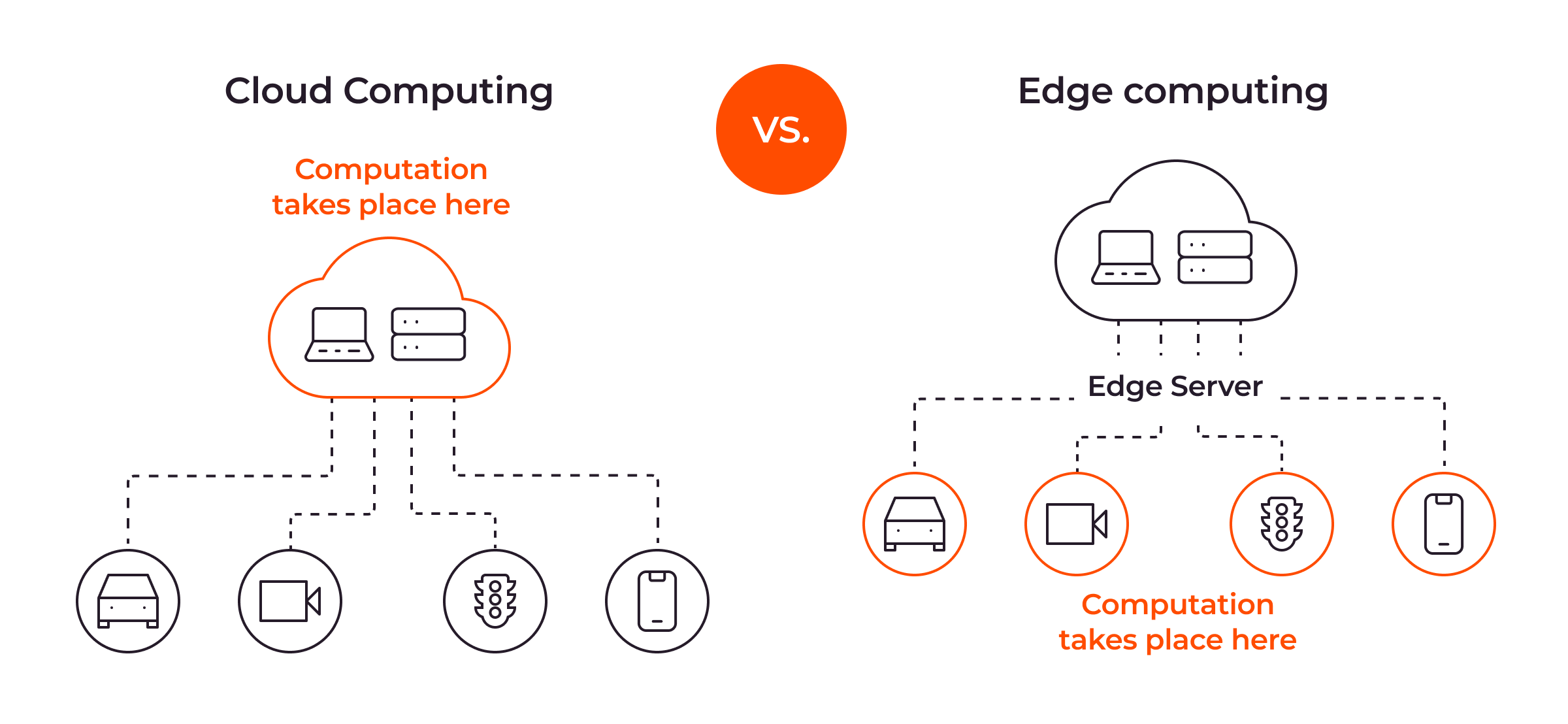
Inference at the edge removes the delays that characterize the transmission of information to distant cloud servers in the traditional model that preceded edge inference. It does so by reducing the physical distance between the device requesting AI inference, and the server where inference is performed. This enables applications to respond to changes or inputs almost instantaneously.
Benefits of AI Inference at the Edge
Shifting to edge AI offers significant benefits for businesses across industries. (In the next section, we’ll look at industry-specific benefits and use cases.)
Real-Time Data Processing
Edge AI transforms business operations by enabling data to be processed almost instantly at or near its source, crucial for sectors where time is of the essence, like gaming, healthcare, and entertainment. This technology dramatically reduces the time lag between data collection and analysis, providing immediate actionable information and allowing businesses to gain real-time insights, make swift decisions, and optimize operations.
Bandwidth Efficiency
By processing data locally, edge AI minimizes the volume of data that needs to be transmitted across networks. This reduction in data transmission alleviates network congestion and improves system performance, critical for environments with high data traffic.
For businesses, this means operations remain uninterrupted and responsive even at peak times and without needing to implement costly network upgrades. This directly translates into tangible financial savings combined with more reliable service delivery for their customers—a win-win scenario from inference at the edge.
Reduced Costs
Edge AI helps businesses minimize the need for frequent data transfers to cloud services, which substantially lowers bandwidth, infrastructure, and storage needs for extensive data management. As a result, this approach makes the entire data-handling process more cost-efficient.
Accessibility and Reliability
Edge AI’s design allows for operation even without consistent internet access by deploying AI applications on local devices, without needing to connect to distant servers. This ensures stable performance and dependability, enabling businesses to maintain high service standards and operational continuity, regardless of geographic or infrastructure constraints.
Enhanced Privacy and Security
Despite spending copious amounts of time and sharing experiences on platforms like TikTok and X, today’s users are increasingly privacy-conscious. There’s good reason for this, as data breaches are on the rise, costing organizations of all sizes millions and compromising individuals’ data. For example, the widely publicized T-Mobile breach in 2022 resulted in $350 million in customer payouts. Companies providing AI-driven capabilities have a strong hold on user engagement and generally promise users control over how models are used, respecting privacy and content ownership. Taking AI data to the edge can contribute to such privacy efforts.
Edge AI’s local data processing means that data analysis can occur directly on the device where data is collected, rather than being sent to remote servers. This proximity significantly reduces the risk of data interception or unauthorized access, as less data is transmitted over networks.
Processing data locally—either on individual devices or a nearby server—makes adherence to privacy regulations and security protocols like GDPR easier. Such regulations require that sensitive data be kept within specific regions. Edge AI achieves this high level of compliance by enabling companies to process data within the same region or country where it’s generated.
For example, a global AI company could have a French user’s data processed by a French edge AI server, and a Californian user’s data processed by a server located in California. This way, the data processing of the two users would automatically adhere to their local laws: the French user’s would be performed in accordance with the European standard GDPR, and the Californian’s according to CCPA and CPRA.
How Edge AI Meets Industries’ Low-Latency Data Processing Needs
While edge AI presents significant advantages across industries, its adoption is more critical in some use cases than others, particularly those that require speed and efficiency to gain and maintain a competitive advantage. Let’s look at some industries where inference at the edge is particularly crucial.
Entertainment
In the entertainment industry, edge AI is allowing providers to offer highly personalized content and interactive features directly to users. It enables significant added value in the form of live sports updates, in-context player information, interactive movie features, real-time user preference analysis, and tailored recommendation generation by optimizing bandwidth usage and cutting out the lag time linked to using remote servers. These capabilities promote enhanced viewer engagement and a more immersive and satisfying entertainment experience.
GenAI
Imagine a company that revolutionizes personalized content by enabling users to generate beautiful, customized images through artificial intelligence, integrating personal elements like pictures they’ve taken of themselves, products, pets, or other personal items. Applications like these already exist.
Today’s users expect immediate responses in their digital interactions. To keep its users engaged and excited, such a company must find ways to meet its users’ expectations or risk losing them to competitors.
The local processing of this entertainment-geared data to prompt image generation tightens its security, as sensitive information doesn’t have to travel over the internet to distant servers. Additionally, by processing user requests directly on devices or nearby servers, edge AI can minimize delays in image generation, making the experience of customizing images fast and allowing for real-time interaction with the application. The result: a deeper, more satisfying connection between users and the technology.
Manufacturing
In manufacturing, edge AI modernizes predictive maintenance and quality control by bringing intelligent processing capabilities right to the factory floor. This allows for real-time monitoring of equipment, leveraging advanced machine vision and the continuous and detailed analysis of vibration, temperature, and acoustic data from machinery to detect quality deviations. The practical impact is a reduction in defects and reduction in downtime via predictive maintenance. Inference at the edge allows the real-time response that’s required for this.
Major companies have already adopted edge AI in this way. For instance, Procter & Gamble’s chemical mix tanks are monitored by edge AI solutions that immediately notify floor managers of quality deviations, preventing flawed products from continuing down the manufacturing line. Similarly, BMW employs a combination of edge computing and AI to achieve a real-time overview of its assembly lines, ensuring the efficiency and safety of its manufacturing operations.
Manufacturing applications of inference at the edge significantly reduce operational costs by optimizing equipment maintenance and quality control. The technology’s ability to process data on-site or nearby transforms traditional manufacturing into a highly agile, cost-effective, and reliable operation, setting a new benchmark for the industry worldwide.
Healthcare
In healthcare, AI inference at the edge addresses critical concerns, such as privacy and security, through stringent data encryption and anonymization techniques, ensuring patient data remains confidential. Edge AI’s compatibility with existing healthcare IT systems, achieved through interoperable standards and APIs, enables seamless integration with current infrastructures. Overall, the impact of edge AI on healthcare is improved care delivery via the enabling of immediate, informed medical decisions based on real-time data insights.
Gcore partnered with a healthcare provider who needed to process sensitive medical data to generate an AI second opinion, particularly in oncological cases. Due to patient confidentiality, the data couldn’t leave the country. As such, the healthcare provider’s best option to meet regulatory compliance while maintaining high performance was to deploy an edge solution connected to their internal system and AI model. With 160+ strategic global locations and proven adherence to GDPR and ISO 27001 standards, we were able to offer the healthcare provider the edge AI advantage they needed.
The result:
- Real-time processing and reduced latency: For the healthcare provider, every second counts, especially in critical oncological cases. By deploying a large model at the edge, close to the hospital’s headquarters, we enabled fast insights and responses.
- Enhanced security and privacy: Maintaining the integrity and confidentiality of patient data was a non-negotiable in this case. By processing the data locally, we ensured adherence to strict privacy standards like GDPR, without sacrificing performance.
- Efficiency and cost reduction: We minimized bandwidth usage by reducing the need for constant data transmission to distant servers—critical for rapid and reliable data turnover—while minimizing the associated costs.
Retail
In retail, edge AI brings precision to inventory management and personalizes the customer experience across a variety of operations. By analyzing data from sensors and cameras in real-time, edge AI predicts restocking needs accurately, ensuring that shelves are always filled with the right products. This technology also powers smart checkout systems, streamlining the purchasing process by eliminating the need for manual scanning, thus reducing wait times and improving customer satisfaction. Retail chatbots and AI customer service bring these benefits to e-commerce.
Inference at the edge make it possible to employ computer vision to understand customer behaviors and preferences in real time, enabling retailers to optimize store layouts and product placements effectively. This insight helps to create a shopping environment that encourages purchases and enhances the overall customer journey. Retailers leveraging edge AI can dynamically adjust to consumer trends and demands, making operations more agile and responsive.
Conclusion
AI inferencing at the edge offers businesses across various industries the ability to process data in real time, directly at the source. This capability reduces latency while enhancing operational efficiency, security, and customer satisfaction, allowing businesses to set a new standard in leveraging technology to gain a competitive advantage.
Gcore is at the forefront of this technological evolution, activating AI inference at the edge across a global network designed to minimize latency and maximize performance. With advanced L40S GPU-based computing resources and a comprehensive list of open-source models, Gcore Edge AI provides a robust, cutting-edge platform for large AI model deployment.